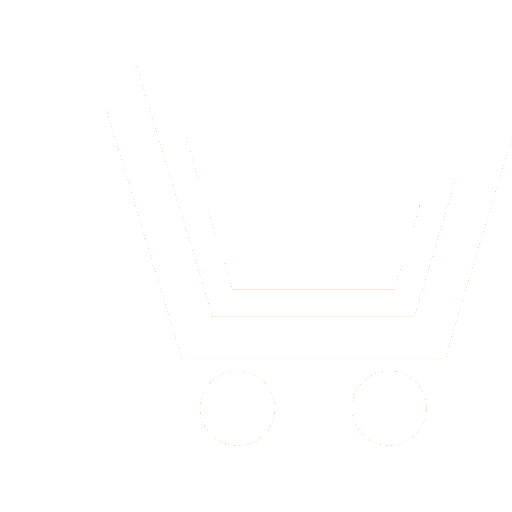
Л. Гу1, Г. У2, Е.А. Попов3, С.Б. Макаров4, Г. Дун5
1,3,4 Санкт-Петербургский политехнический университет Петра Великого (Санкт-Петербург, Россия)
2 Московский государственный технический университет им. Н.Э. Баумана (Москва, Россия)
5 Университет Цинхуа (Пекин, КНР)
1 gu2.l@edu.spbstu.ru; 2 ug@student.bmstu.ru; 3 popov@spbstu.ru; 4 makarov@cee.spbstu.ru; 5 dongge@tsinghua.edu.cn
Постановка проблемы. Обнаружение объектов на изображениях, полученных посредством дистанционного зондирования (ДЗ), является актуальной задачей в области наблюдения за Землей, обладающей большим потенциалом для предотвращения природных катастроф и мониторинга окружающей среды. Эта задача сводится к определению положения и классификации определенных объектов на изображениях, полученных с космических спутников или летательных аппаратов. Использование при решении задач классификации изображений обеспечивает более эффективный учёт пространственной зависимости в частотной (спектральной) области.
Цель. Исследовать возможность повышения точности обнаружения мелких объектов с помощью контекстной информации через операции в спектральной области.
Результаты. Предложена архитектура спектрально-ориентированной пирамиды признаков (FFPF), состоящей из двух основных компонентов: спектрально-ориентированной нейронной сети ResNet (F-ResNet) и двусторонней спектрально-ориенти-рованнай нейронной сети пирамиды признаков (BS-FPN). Рассмотрена сеть F-ResNet, содержащая модуль backbone для извлечения пространственных признаков и Фурье-блок для получения спектрального глобального контекста. Разработана сеть BS-FPN с использованием стратегии двусторонней выборки и пропускающего соединения для моделирования ассоциации признаков объектов на разных масштабах. Предложена и обучена на наборе данных DIOR архитектура FFPF, обеспечивающая среднюю точность (mAP) 73,8%.
Практическая значимость. Представленная архитектура позволяет повысить точность обнаружения мелких объектов на изображениях.
Гу Л., У Г., Попов Е.А., Макаров С.Б., Дун Г. Повышение точности обнаружения мелких объектов на изображениях с помощью контекстной информации // Радиотехника. 2024. Т. 88. № 3. С. 63−77. DOI: https://doi.org/10.18127/j00338486-202403-07
- Girshick R., Donahue J., Darrell T., Malik J. Rich feature hierarchies for accurate object detection and semantic segmentation // in Proceedings of the IEEE conference on computer vision and pattern recognition. 2014. P. 580–587.
- Girshick R. Fast r-cnn // in Proceedings of the IEEE international conference on computer vision. 2015. P. 1440–1448.
- Ren S., He K., Girshick R., Sun J. Faster r-cnn: Towards real-time object detection with region proposal networks // Advances in neural information processing systems. 2015. V. 28.
- Liu W., Anguelov D., Erhan D., Szegedy C., Reed S., Fu C.-Y., Berg A.C. Ssd: Single shot multibox detector // in European conference on computer vision. Springer. 2016. P. 21–37.
- Redmon J., Divvala S., Girshick R., Farhadi A. You only look once: Unified, real-time object detection // in Proceedings of the IEEE conference on computer vision and pattern recognition. 2016. P. 779–788.
- Hu J., Shen L., Sun G. Squeeze-and-excitation networks // in Proceedings of the IEEE conference on computer vision and pattern recognition. 2018. P. 7132–7141.
- Lin T.-Y., Dollár P., Girshick R., He K., Hariharan B., Belongie S. Feature pyramid networks for object detection // in Proceedings of the IEEE conference on computer vision and pattern recognition. 2017. P. 2117–2125.
- Van Etten A. You only look twice: Rapid multi-scale object detection in satellite imagery // arXiv preprint arXiv:1805.09512, 2018.
- Yang X., Yang J., Yan J., Zhang Y., Zhang T., Guo Z., Sun X., Fu K. Scrdet: Towards more robust detection for small, cluttered and rotated objects // in Proceedings of the IEEE/CVF International Conference on Computer Vision. 2019. P. 8232–8241.
- Qingyun F., Lin Z., Zhaokui W. An efficient feature pyramid network for object detection in remote sensing imagery // IEEE Access. 2020. V. 8. P. 93 058–93 068.
- Chi L., Jiang B., Mu Y. Fast fourier convolution // Advances in Neural Information Processing Systems. 2020. V. 33. P. 4479–4488.
- Rao Y., Zhao W., Zhu Z., Lu J., Zhou J. Global filter networks for image classification // Advances in Neural Information Processing Systems. 2021. V. 34.
- Suvorov R., Logacheva E., Mashikhin A., Remizova A., Ashukha A., Silvestrov A., Kong N., Goka H., Park K., Lempitsky V. Resolution-robust large mask inpainting with fourier convolutions // in Proceedings of the IEEE/CVF Winter Conference on Applications of Computer Vision. 2022. P. 2149–2159.
- Katznelson Y. An introduction to harmonic analysis. Cambridge University Press. 2004.
- Li K., Wan G., Cheng G., Meng L., Han J. Object detection in optical remote sensing images: A survey and a new benchmark // ISPRS Journal of Photogrammetry and Remote Sensing. 2020. V. 159. P. 296–307.
- Xia G.-S., et al. DOTA: A large-scale dataset for object detection in aerial images // in Proc. IEEE/CVF Conf. Comput. Vis. Pattern Recognit. Jun. 2018. P. 3974–3983.
- Yang A., Li M., Wu Z., et al. CDF‐net: A convolutional neural network fusing frequency domain and spatial domain features [J]. // IET Computer Vision. 2023. V. 17. № 3. Р. 319-329.
- Lin T.Y., Maire M., Belongie S., et al. Microsoft coco: Common objects in context[C] // Computer Vision–ECCV 2014: 13th Eu-ropean Conference (Zurich, Switzerland, September 6-12, 2014). Proceedings. Part V 13. Springer International Publishing. 2014. Р. 740-755.
- Huang G., Liu Z., Van Der Maaten L., et al. Densely connected convolutional networks[C] // Proceedings of the IEEE conference on computer vision and pattern recognition. 2017. Р. 4700-4708.
- Ma W., Li N., Zhu H., et al. Feature split–merge–enhancement network for remote sensing object detection [J] // IEEE Transactions on Geoscience and Remote Sensing. 2022. V. 60. Р. 1-17.
- Liu Y., Li Q., Yuan Y., et al. ABNet: Adaptive balanced network for multiscale object detection in remote sensing imagery [J] // IEEE Transactions on Geoscience and Remote Sensing. 2021. V. 60. Р. 1-14.
- Hou J.B., Zhu X., Yin X.C. Self-adaptive aspect ratio anchor for oriented object detection in remote sensing images [J] // Remote Sensing. 2021. V. 13. № 7. Р. 1318.
- Wang X., Girshick R., Gupta A., et al. Non-local neural networks [C] // Proceedings of the IEEE conference on computer vision and pattern recognition. 2018. Р. 7794-7803.
- Cao Y., Xu J., Lin S., et al. Gcnet: Non-local networks meet squeeze-excitation networks and beyond [C] // Proceedings of the IEEE/CVF international conference on computer vision workshops. 2019.
- Rippel O., Snoek J., Adams R.P. Spectral representations for convolutional neural networks [J] // Advances in neural information processing systems 2015. Р. 28.
- Zhong Z., Shen T., Yang Y., et al. Joint sub-bands learning with clique structures for wavelet domain super-resolution [J] // Advances in neural information processing systems. 2018. Р. 31.
- Chi L., Tian G., Mu Y., et al. Fast non-local neural networks with spectral residual learning [C] // Proceedings of the 27th ACM International Conference on Multimedia. 2019. Р. 2142-2151.
- Xu Q., Zhang R., Zhang Y., Wang Y., Tian Q. A fourier-based framework for domain generalization // in Proceedings of the IEEE/CVF Conference on Computer Vision and Pattern Recognition. 2021. P. 14 383–14 392.
- Han K., Wang Y., Tian Q., et al. Ghostnet: More features from cheap operations [C] // Proceedings of the IEEE/CVF conference on computer vision and pattern recognition. 2020. Р. 1580-1589.
- Liu S., Qi L., Qin H., Shi J., Jia J. Path aggregation network for instance segmentation // in Proceedings of the IEEE conference on computer vision and pattern recognition. 2018. P. 8759–8768.
- Wang J., Chen K., Xu R., Liu Z., Loy C.C., Lin D. Carafe: Content-aware reassembly of features // in Proceedings of the IEEE/CVF International Conference on Computer Vision. 2019. P. 3007–3016.
- Redmon J., Farhadi A. Yolov3: An incremental improvement // arXiv preprint arXiv:1804.02767. 2018.
- Simonyan K., Zisserman A. Very deep convolutional networks for large-scale image recognition // arXiv preprint arXiv:1409.1556. 2014.
- He K., Zhang X., Ren S., Sun J. Deep residual learning for image recognition // in Proceedings of the IEEE conference on computer vision and pattern recognition. 2016. P. 770–778.
- He K., Gkioxari G., Dollár P., Girshick R. Mask r-cnn // in Proceedings of the IEEE international conference on computer vision. 2017. P. 2961–2969.
- Lin T.-Y., Goyal P., Girshick R., He K., Dollár P. Focal loss for dense object detection // in Proceedings of the IEEE international conference on computer vision. 2017. P. 2980–2988.
- Cheng G., Si Y., Hong H., Yao X., Guo L. Cross-scale feature fusion for object detection in optical remote sensing images // IEEE Geoscience and Remote Sensing Letters. 2020. V. 18. № 3. P. 431–435.
- Cheng G., He M., Hong H., Yao X., Qian X., Guo L. Guiding clean features for object detection in remote sensing images // IEEE Geoscience and Remote Sensing Letters. 2021.
- Carion N., Massa F., Synnaeve G., et al. End-to-end object detection with transformers [C] // European conference on computer vision. Cham: Springer International Publishing. 2020. Р. 213-229.