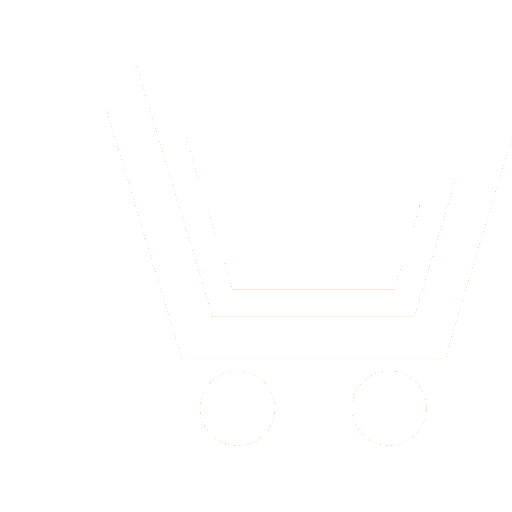
L. Gu1, G. Wu2, E.A. Popov3, S.B. Makarov4, G. Dong5
1,3,4 Peter the Great St. Petersburg Polytechnic University (St. Petersburg, Russia)
2 Moscow Bauman State Technical University (Moscow, Russia)
5 Tsinghua University (Beijing, China)
1 gu2.l@edu.spbstu.ru; 2 ug@student.bmstu.ru; 3 popov@spbstu.ru; 4 makarov@cee.spbstu.ru; 5 dongge@tsinghua.edu.cn
Formulation of the problem. Detecting objects in remote sensing images is an important technology for Earth observation and is used in various applications such as forest fire monitoring and ocean monitoring. However, due to the limited number of pixels of small objects, it is difficult to process remote sensing images. An effective way to improve small object detection is to introduce spatial context. For image classification, spectral convolution can more effectively perceive long-term spatial dependence in the frequency domain than in the spatial domain.
The goal is to improve the detection accuracy of remote sensing small objects using contextual information through frequency domain operations.
Results. A Frequency-aware Feature Pyramid Framework (FFPF) is proposed, which consists of two main components: Frequency-aware ResNet (F-ResNet) and Bilateral Spectral-aware Feature Pyramid Network (BS-FPN). F-ResNet is proposed, which consists of two parts: a spatial convolutional backbone for extracting spatial features and spectral convolutional modules (Fourier Unit) for obtaining spectral global context. Developed BS-FPN using a bilateral sampling and skipping connection strategy to model object feature association at different scales. The proposed FFPF trained on the DIOR dataset achieves an average precision (mAP) of 73.8%. Experimental results compared with other methods, ablation studies, and qualitative analysis demonstrate the effectiveness of the proposed FFPF.
Practical significance. The presented framework allows to improve the accuracy of detection of small remote sensing objects.
Gu L., Wu G., Popov E.A., Makarov S.B., Dong G. Fast fourier convolution based remote sensing image object detection for Earth observation. Radiotekhnika. 2024. V. 88. № 3. P. 63−77. DOI: https://doi.org/10.18127/j00338486-202403-07 (In Russian)
- Girshick R., Donahue J., Darrell T., Malik J. Rich feature hierarchies for accurate object detection and semantic segmentation. in Proceedings of the IEEE conference on computer vision and pattern recognition. 2014. P. 580–587.
- Girshick R. Fast r-cnn. in Proceedings of the IEEE international conference on computer vision. 2015. P. 1440–1448.
- Ren S., He K., Girshick R., Sun J. Faster r-cnn: Towards real-time object detection with region proposal networks. Advances in neural information processing systems. 2015. V. 28.
- Liu W., Anguelov D., Erhan D., Szegedy C., Reed S., Fu C.-Y., Berg A.C. Ssd: Single shot multibox detector. in European conference on computer vision. Springer. 2016. P. 21–37.
- Redmon J., Divvala S., Girshick R., Farhadi A. You only look once: Unified, real-time object detection. in Proceedings of the IEEE conference on computer vision and pattern recognition. 2016. P. 779–788.
- Hu J., Shen L., Sun G. Squeeze-and-excitation networks. in Proceedings of the IEEE conference on computer vision and pattern recognition. 2018. P. 7132–7141.
- Lin T.-Y., Dollár P., Girshick R., He K., Hariharan B., Belongie S. Feature pyramid networks for object detection. in Proceedings of the IEEE conference on computer vision and pattern recognition. 2017. P. 2117–2125.
- Van Etten A. You only look twice: Rapid multi-scale object detection in satellite imagery. arXiv preprint arXiv:1805.09512, 2018.
- Yang X., Yang J., Yan J., Zhang Y., Zhang T., Guo Z., Sun X., Fu K. Scrdet: Towards more robust detection for small, cluttered and rotated objects. in Proceedings of the IEEE/CVF International Conference on Computer Vision. 2019. P. 8232–8241.
- Qingyun F., Lin Z., Zhaokui W. An efficient feature pyramid network for object detection in remote sensing imagery. IEEE Access. 2020. V. 8. P. 93 058–93 068.
- Chi L., Jiang B., Mu Y. Fast fourier convolution. Advances in Neural Information Processing Systems. 2020. V. 33. P. 4479–4488.
- Rao Y., Zhao W., Zhu Z., Lu J., Zhou J. Global filter networks for image classification. Advances in Neural Information Processing Systems. 2021. V. 34.
- Suvorov R., Logacheva E., Mashikhin A., Remizova A., Ashukha A., Silvestrov A., Kong N., Goka H., Park K., Lempitsky V. Resolution-robust large mask inpainting with fourier convolutions. in Proceedings of the IEEE/CVF Winter Conference on Applications of Computer Vision. 2022. P. 2149–2159.
- Katznelson Y. An introduction to harmonic analysis. Cambridge University Press. 2004.
- Li K., Wan G., Cheng G., Meng L., Han J. Object detection in optical remote sensing images: A survey and a new benchmark. ISPRS Journal of Photogrammetry and Remote Sensing. 2020. V. 159. P. 296–307.
- Xia G.-S., et al. DOTA: A large-scale dataset for object detection in aerial images. in Proc. IEEE/CVF Conf. Comput. Vis. Pattern Recognit. Jun. 2018. P. 3974–3983.
- Yang A., Li M., Wu Z., et al. CDF‐net: A convolutional neural network fusing frequency domain and spatial domain features [J].. IET Computer Vision. 2023. V. 17. № 3. Р. 319-329.
- Lin T.Y., Maire M., Belongie S., et al. Microsoft coco: Common objects in context[C]. Computer Vision–ECCV 2014: 13th Eu-ropean Conference (Zurich, Switzerland, September 6-12, 2014). Proceedings. Part V 13. Springer International Publishing. 2014. Р. 740-755.
- Huang G., Liu Z., Van Der Maaten L., et al. Densely connected convolutional networks[C]. Proceedings of the IEEE conference on computer vision and pattern recognition. 2017. Р. 4700-4708.
- Ma W., Li N., Zhu H., et al. Feature split–merge–enhancement network for remote sensing object detection [J]. IEEE Transactions on Geoscience and Remote Sensing. 2022. V. 60. Р. 1-17.
- Liu Y., Li Q., Yuan Y., et al. ABNet: Adaptive balanced network for multiscale object detection in remote sensing imagery [J]. IEEE Transactions on Geoscience and Remote Sensing. 2021. V. 60. Р. 1-14.
- Hou J.B., Zhu X., Yin X.C. Self-adaptive aspect ratio anchor for oriented object detection in remote sensing images [J]. Remote Sensing. 2021. V. 13. № 7. Р. 1318.
- Wang X., Girshick R., Gupta A., et al. Non-local neural networks [C]. Proceedings of the IEEE conference on computer vision and pattern recognition. 2018. Р. 7794-7803.
- Cao Y., Xu J., Lin S., et al. Gcnet: Non-local networks meet squeeze-excitation networks and beyond [C]. Proceedings of the IEEE/CVF international conference on computer vision workshops. 2019.
- Rippel O., Snoek J., Adams R.P. Spectral representations for convolutional neural networks [J]. Advances in neural information processing systems 2015. Р. 28.
- Zhong Z., Shen T., Yang Y., et al. Joint sub-bands learning with clique structures for wavelet domain super-resolution [J]. Advances in neural information processing systems. 2018. Р. 31.
- Chi L., Tian G., Mu Y., et al. Fast non-local neural networks with spectral residual learning [C]. Proceedings of the 27th ACM International Conference on Multimedia. 2019. Р. 2142-2151.
- Xu Q., Zhang R., Zhang Y., Wang Y., Tian Q. A fourier-based framework for domain generalization. in Proceedings of the IEEE/CVF Conference on Computer Vision and Pattern Recognition. 2021. P. 14 383–14 392.
- Han K., Wang Y., Tian Q., et al. Ghostnet: More features from cheap operations [C]. Proceedings of the IEEE/CVF conference on computer vision and pattern recognition. 2020. Р. 1580-1589.
- Liu S., Qi L., Qin H., Shi J., Jia J. Path aggregation network for instance segmentation. in Proceedings of the IEEE conference on computer vision and pattern recognition. 2018. P. 8759–8768.
- Wang J., Chen K., Xu R., Liu Z., Loy C.C., Lin D. Carafe: Content-aware reassembly of features. in Proceedings of the IEEE/CVF International Conference on Computer Vision. 2019. P. 3007–3016.
- Redmon J., Farhadi A. Yolov3: An incremental improvement. arXiv preprint arXiv:1804.02767. 2018.
- Simonyan K., Zisserman A. Very deep convolutional networks for large-scale image recognition. arXiv preprint arXiv:1409.1556. 2014.
- He K., Zhang X., Ren S., Sun J. Deep residual learning for image recognition. in Proceedings of the IEEE conference on computer vision and pattern recognition. 2016. P. 770–778.
- He K., Gkioxari G., Dollár P., Girshick R. Mask r-cnn. in Proceedings of the IEEE international conference on computer vision. 2017. P. 2961–2969.
- Lin T.-Y., Goyal P., Girshick R., He K., Dollár P. Focal loss for dense object detection. in Proceedings of the IEEE international conference on computer vision. 2017. P. 2980–2988.
- Cheng G., Si Y., Hong H., Yao X., Guo L. Cross-scale feature fusion for object detection in optical remote sensing images. IEEE Geoscience and Remote Sensing Letters. 2020. V. 18. № 3. P. 431–435.
- Cheng G., He M., Hong H., Yao X., Qian X., Guo L. Guiding clean features for object detection in remote sensing images. IEEE Geoscience and Remote Sensing Letters. 2021.
- Carion N., Massa F., Synnaeve G., et al. End-to-end object detection with transformers [C]. European conference on computer vision. Cham: Springer International Publishing. 2020. Р. 213-229.