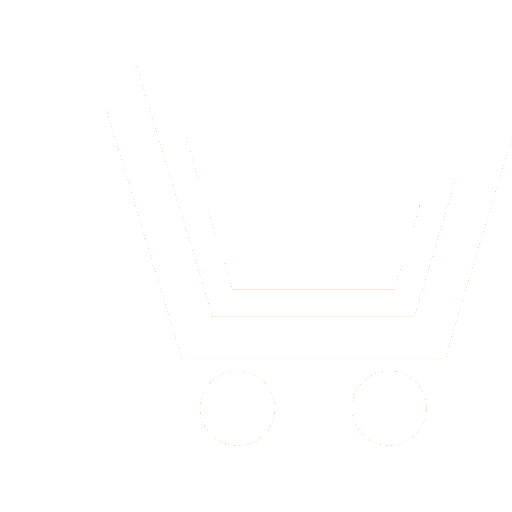
М.В. Маркитантов – мл. науч. сотрудник,
Санкт-Петербургский институт информатики и автоматизации РАН
А.А. Карпов – д.т.н., доцент, гл. науч. сотрудник,
Санкт-Петербургский институт информатики и автоматизации РАН
Постановка проблемы. Современные системы автоматического распознавания возраста и пола диктора не позволяют получить удовлетворительную точность распознавания. Это осложняется влиянием таких факторов, как, например, фоновый шум и голосовая вариативность.
Цель. Разработать подходы для автоматического распознавания возраста и пола диктора, которые позволят увеличить точность распознавания.
Результаты. Результаты исследования могут использоваться в различных автоматических системах верификации и идентификации дикторов, в частности, в работе телефонных контакт-центров, учреждений здравоохранения и для повышения эффективности целевой рекламы, что обуславливает практическую значимость работы. В работе использовались методы исследования, среди которых ведущими были следующие: анализ, синтез, обобщение и эксперимент. Проводились эксперименты с использованием разных топологий DNN, включая нейронные сети с полносвязными и сверточными слоями. Обучение и тестирование предложенных моделей производилось на корпусе немецкой речи aGender. При распознавании пола и возраста по отдельности получена точность 88,10% и 57,53% соответственно. В совместном распознавании пола и возраста диктора предложенная система достигла точности (accuracy) 48,41%.
Практическая значимость. DNN позволила достичь лучшего результата по распознаванию возраста диктора по голосу в сравнении с существующими классическими подходами.
- Ranzato M., Hinton G. Modeling pixel means and covariances using factorized third order boltzmann machines // IEEE Conference on Computer Vision and Pattern Recognition (CVPR). 2010. P. 2551−2558.
- Lee H., Ekanadham C., Ng A. Sparse deep belief netmodel for visual area v2 // Proc. of the 20th International Conference on Neural Information Processing Systems. 2007. P. 873−880.
- Dahl G., Yu D., Deng L., Acero A. Context-dependent pre-trained deep neural networks for large-vocabulary speech recognition // IEEE Transactions on Audio, Speech, and Language Processing. 2012. V. 20. P. 30−42.
- Deselaers T., Hasan S., Bender O., Ney H. A deep learning approach to machine transliteration // Proc. of the Fourth Workshop on Statistical Machine Translation. 2009. P. 233−241.
- Yu D., Wang S., Karam Z., Deng L. Language recognition using deep-structured conditional random fields // IEEE International Conference on Acoustics Speech and Signal Processing (ICASSP). 2010. P. 5030−5033.
- Schuller B., Steidl S., Batliner A., Burkhardt F., Devillers L., Müller C., Narayanan S. The INTERSPEECH 2010 paralinguistic challenge // Proc. of the 11th Annual Conference of the International Speech Communication Association, INTERSPEECH 2010. P. 2794−2797.
- Burkhardt F., Eckert M., Johannsen W., Stegmann J. A database of age and gender annotated telephone speech // Proc. of 7th International Conference on Language Resources and Evaluation (LREC 2010). 2010.
- Eyben F., Wöllmer M., Schuller B. openSMILE – the Munich versatile and fast open-source audio feature extractor // Proc. of the ACM Multimedia 2010 International Conference. 2010. P. 1459−1462.
- Kockmann M., Burget L., Cernocký J. Brno University of Technology system for Interspeech 2010 Paralinguistic Challenge // Proc. of the 11th Annual Conference of the International Speech Communication Association, INTERSPEECH 2010. P. 2822−2825.
- Meinedo H., Trancoso I. Age and gender classification using fusion of acoustic and prosodic features // Proc. of the 11th Annual Conference of the International Speech Communication Association, INTERSPEECH 2010. P. 2818−2821.
- Li M., Han K., Narayanan S. Automatic speaker age and gender recognition using acoustic and prosodic level information fusion // Computer Speech & Language. 2013. V. 27. № 1. P. 151−167.
- Yücesoy E., Nabiyev V. A new approach with score-level fusion for the classification of a speaker age and gender // Computers & Electrical Engineering. 2016. P. 29−39.
- Równicka J., Kacprzak S. Speaker Age Classification and Regression Using i-Vectors // Proc. of the 17th Annual Conference of the International Speech Communication Association (INTERSPEECH 2016): Understanding Speech Processing in Humans and Machines. 2016. P. 1402−1406.
- Sadjadi S., Slaney M., Heck L. MSR identity toolbox v1.0: A Matlab toolbox for speaker-recognition research // Speech and Language Processing Technical Committee Newsletter. 2013.
- Qawaqneh Z., Abumallouh A., Barkana B. Deep Neural Network Framework and Transformed MFCCs for Speaker's Age and Gender Classification // Knowledge-Based Systems. 2016. V. 115. P. 5−14.
- Abumallouh A., Qawaqneh Z., Barkana B. New transformed features generated by deep bottleneck extractor and a GMM-UBM classifier for speaker age and gender classification // Neural Computing and Applications. 2017. V. 30. № 8. P. 2581−2593.
- Ghahremani P., Sankar Nidadavolu P., Chen N., Villalba J., Povey D., Khudanpur S., Dehak N. End-to-end Deep Neural Network Age Estimation // Proc. of the 19th Annual Conference of the International Speech Communication Association, INTERSPEECH 2018. P. 277−281.
- Snyder D., Garcia-Romero D., Sell G., Povey D., Khudanpur S. X-Vectors: Robust DNN Embeddings for Speaker Recognition // Proc. of the IEEE International Conference on Acoustics, Speech and Signal Processing (ICASSP). 2018. P. 5329−5333.
- McFee B., Raffel C., Liang D., Ellis D., Mcvicar M., Battenberg E., Nieto O. librosa: audio and music signal analysis in Python // Proc. of the 14th python in science conference. 2015. P. 18−24.
- Paszke A., Gross S., Chintala S., Chanan G., Yang E., DeVito Z., Lin Z., Desmaison A., Antiga L., Lerer A. Automatic differentiation in PyTorch // Proc. of the 31st Conference on Neural Information Processing Systems (NIPS 2017). 2017.
- Markitantov M., Verkholyak O. Automatic Recognition of Speaker Age and Gender Based on Deep Neural Networks // Lecture Notes in Computer Science. Springer LNAI 11658. SPECOM 2019. P. 327−336.
- Bocklet T., Stemmer G., Zeißler V., Noeth E. Age and gender recognition based on multiple systems – early vs. late fusion // Proc. of the 11th Annual Conference of the International Speech Communication Association, INTERSPEECH 2010. P. 2830−2833.
- Nguyen P., Le T., Tran D., Huang X., Sharma D. Fuzzy support vector machines for age and gender classification // Proc. of the 11th Annual Conference of the International Speech Communication Association, INTERSPEECH 2010. P. 2806−2809.
- Gajsek R., Žibert J., Justin T., Štruc V., Vesnicer B., Mihelic F. Gender and affect recognition based on GMM and GMM-UBM modeling with relevance MAP estimation // Proc. of the 11th Annual Conference of the International Speech Communication Association, INTERSPEECH 2010. P. 2810−2813.