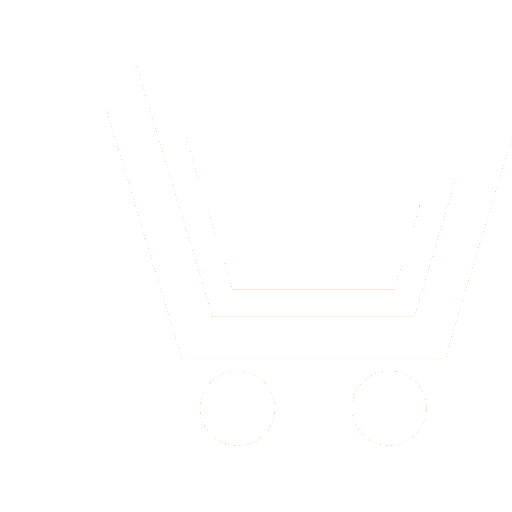
Е.В. Пугин – аспирант, кафедра «САПР»,
Муромский филиал Владимирского государственного университета им. А.Г. и Н.Г. Столетовых E-mail: egor.pugin@gmail.com
А.Л. Жизняков – д.т.н., профессор, первый зам. директора
Муромского филиала Владимирского государственного университета им. А.Г. и Н.Г. Столетовых E-mail: lvovich@newmail.ru
Рассмотрено применение нечетких множеств и нечеткой логики в задачах обработки изображений. Приведены ключевые понятия этих теорий, дано краткое описание видов нечетких множеств. Проанализированы известные подходы методов нечеткой обработки изображений в прикладных задачах. Показаны недостатки этих подходов, предложены направления по улучшению алгоритмов обработки изображений на основе нечетких признаков.
- Zakharov A., Tuzhilkin A., Zhiznyakov A. Automatic building detectionfrom satellite images using spectral graph theory // IEEE International Conference on Mechanical Engineering, Automation and Control Systems (MEACS). 12.2015.
- Privezentsev D.G., Zhiznyakov A.L. Use of characteristic image segmentsin tasks of digital image processing // IEEE International Conference «Stability and Control Processes» in Memory of V.I. Zubov (SCP). 10.2015.
- Zhiznyakov A.L., Privezentsev D.G., Zakharov A.A. Using fractal features of digital images for the detection of surface defects // Pattern Recognition and Image Analysis. Январь 2015. Т. 25. № 1. С. 122−131.
- Кофман А. Введение в теорию нечетких множеств. М.: Радио и связь. 1982. 432 с.
- Zadeh L.A. Fuzzy sets // Information and Control. 1965. V. 8. № 3. P. 338−353.
- Круглов В.В., Дли M.И., Голунов Р.Ю. Нечеткая логика и искусственные нейронные сети. М.: Физматлит. 2000. 224 с.
- Кузьмин В.Б. Построение групповых решений в пространствах четких и нечетких бинарных отношений. М.: Наука. 1982. 168 с.
- Нечеткие множества и теория возможностей: Последние достижения / Под ред. Р.Р. Ягера. М.: Радио и связь. 1986. 408 с.
- Заде Л. Понятие лингвистической переменной и его применение к принятию приближенных решений. М.: Мир. 1976. 166 с.
- Тэрано Т., Асаи К., Сугэно М.М. Прикладные нечеткие системы. М.: Мир. 1993. 368 с.
- Blizard W.D. Multiset theory // Notre Dame J. Formal Logic. December 1988. V. 30. № 1. P. 36−66.
- Zadeh L.A. The Concept of a Linguistic Variable and its Application to Approximate Reasoning // Learning Systems and Intelligent Robots. Springer Science + Business Media. 1974. P. 1−10.
- Mendel J.M., John R.I.B. Type‑2 fuzzy sets made simple // IEEE Transactions on Fuzzy Systems. April 2002. V. 10. № 2. P. 117−127.
- Ремезова Е.М. Нечеткие множества второго порядка: понятие, анализ и особенности применения // Современные проблемы науки и образования. 2013. № 5.
- Pawlak Z. Rough sets // International Journal of Computer and Information Sciences. October 1982. V. 11. № 5. P. 341−356.
- Molodtsov D. Soft set theory – First results // Computers & Mathematics with Applications. 1999. V. 37. № 4. P. 19−31.
- Молодцов Д.А. Теория мягких множеств. М.: Едиториал УРСС. 2004. 360 с.
- Kharal A., Ahmad B. Mappings on soft classes // New Mathematics and Natural Computation. 2011. V. 07. № 3. P. 471−481.
- Bezdek J.C., et al. Fuzzy Models and Algorithms for Pattern Recognition and Image Processing. Springer US. 1999. V. 4 (The Handbooks of Fuzzy Sets Series).
- Bezdek J.C. Pattern Recognition with Fuzzy Objective Function Algorithms. Norwell, MA, USA: Kluwer Academic Publishers. 1981.
- Li D., Pedrycz W., Pizzi N.J. Fuzzy wavelet packet based feature extraction method and its application to biomedical signal classification // IEEE Transactions on Biomedical Engineering. June 2005. V. 52. № 6. P. 1132−1139.
- Jensen R. Combining rough and fuzzy sets for feature selection: PhD thesis. School of Informatics University of Edinburgh. 2005.
- Каркищенко А.Н., Бутенков С.А., Кривша В.В. Распознавание в системах экологического мониторинга с применением нечетких геометрических признаков // Известия ТРТУ (Таганрогский государственный радиотехнический университет). 2000. С. 144−147.
- Геппенер В.В., Соколов М.А. Классификация подповерхностных объектов в задачах геолокации на основе использования нечетких признаков // Междунар. конф. по мягким вычислениям и измерениям (Proceedings of SCM’99 (International Conference on Soft Computing and Measurements). СПб.: 1999. С. 198−200.
- Тэрано Т., Масуи А., Коно С. Распознавание формы овощей с помощью нечеткой логики // Тез. докл. 3-го национального симпозиума по нечетким системам. Токио (Япония). 1987.
- Хирота, Араи, Хатиси. Распознавание движущихся целей с помощью нечеткой логики и робот для перемещения движущихся объектов // Тез. докл. 3-го национального симпозиума по нечетким системам. Токио (Япония). 1987.
- Obradović D., et al. Intelligent Systems: Models and Applications // Revised and Selected Papers from the 9th IEEE International Symposium on Intelligent Systems and Informatics (SISY). 2011. Ed. by Pap E. Berlin, Heidelberg: Springer Berlin Heidelberg, 2013. Chap. Fuzzy Geometry in Linear Fuzzy Space. P. 137−153.
- Глова В.И., Аникин И.В. Разработка метода распознавания размытых 2D-примитивов изображения // Вестник Казанского государственного технического университета им. А.Н. Туполева. 2000. № 4. С. 66−72.
- Глова В.И., Аникин И.В., Аджели М.А. Нечеткая модель распознавания размытых двумерных форм // Вестник Казанского государственного технического университета им. А.Н. Туполева. 2001. № 3. С. 32−36.
- Han J.H., Koczy L.T., Poston T. Fuzzy Hough transform // Second IEEE International Conference on Fuzzy Systems. 1993. V. 2. 803−808.
- Suetake N., Uchino E., Hirata K. Generalized Fuzzy Hough Transform for Detecting Arbitrary Shapes in a Vague and Noisy Image // Soft Computing. 2006. V. 10. № 12. P. 1161−1168.
- Пугин Е.В., Жизняков А.Л. Фильтрация значимых признаков нечеткого преобразования Хафа // Динамика систем, механизмов и машин. 2016. Т. 2. № 1. С. 284−290.
- Rosenfeld A. Fuzzy geometry: An updated overview // Information Sciences. 1998. V. 110. № 3/4. P. 127−133.
- Bloch I. Fuzzy relative position between objects in image processing: a morphological approach // IEEE Transactions on Pattern Analysis and Machine Intelligence. July 1999. V. 21. № 7. P. 657−664.
- Chen Z., Qiu T., Ruan S. Fuzzy adaptive level set algorithm for brain tissue segmentation // 9th International Conference on Signal Processing. 10.2008. P. 1047−1050.
- Begelrnan G., et al. Cell nuclei segmentation using fuzzy logic engine // International Conference on Image Processing (ICIP). 10/2004. V. 5. P. 2937−2940.
- Kass M., Witkin A., Terzopoulos D. Snakes: Active contour models // International Journal of Computer Vision. 1988. V. 1. № 4. P. 321−331.
- Krinidis S., Chatzis V. Fuzzy Energy-Based Active Contours // IEEE Transactions on Image Processing. December 2009. V. 18. № 12. P. 2747−2755.
- Shi J., et al. An interval type‑2 fuzzy active contour model for auroral oval segmentation // Soft Computing. 2015. P. 1−21.
- Thieu Q.T., et al. Efficient segmentation with the convex local-global fuzzy Gaussian distribution active contour for medical applications // Annals of Mathematics and Artificial Intelligence. 2015. V. 75. № 1. P. 249−266.
- Петров В.О., Привалов О.О. Модификация алгоритма активных контуров для решения задачи интерактивной сегментации растровых изображений дефектов металлических отливок // Современные проблемы науки и образования. 2008. № 6. С. 14−19.
- Höwing F., Dooley L.S., Wermser D. Linguistic Contour Modelling through a Fuzzy Active Contour // New Frontiers in Computational Intelligence and its Applications (UK: IOS Press). 2000. V. 57 (ed. by M. Mohammadian). P. 274−282. (Frontiers in Artificial Intelligence and Applications).
- Gong M., et al. An efficient bi-convex fuzzy variational image segmentation method // Information Sciences. 2015. V. 293. P. 351−369.
- Shi J., et al. Change Detectionin Synthetic Aperture Radar Images Based on Fuzzy Active Contour Models and Genetic Algorithms // MathematicalProblems in Engineering. 2014. V. 2014. № 15. P. 10−1155 (Article ID 870936).
- Тишкин Р.В. Мягкие вычисления в задачах сегментации космических изображений // Цифровая обработка сигналов. 2010. № 3. С. 25−29.
- Phophalia A., Mitra S.K., Rajwade A. A new denoising filter for brain MRimages // Proceedings of the Eighth Indian Conference on Computer Vision, Graphics and Image Processing (ICVGIP). 2012. Association for Computing Machinery (ACM).
- Phophalia A., Mitra S.K., Rajwade A. Object boundary detection using Rough Set Theory // Fourth National Conference on Computer Vision, Pattern Recognition, Image Processing and Graphics (NCVPRIPG). 12/2013. P. 1−4.
- Munshi P., Mitra S.K. A rough-set based binarization technique for fingerprint images // IEEE International Conference on Signal Processing, Computing and Control (ISPCC). 03/2012. P. 1−6.
- Pal S.K., Shankar B.U., Mitra P. Granular computing, rough entropy and object extraction // Pattern Recognition Letters. 2005. V. 26. № 16. P. 2509−2517.
- Małyszko D., Stepaniuk J. Adaptive multilevel rough entropy evolutionary thresholding // Information Sciences. 2010. V. 180. № 7. P. 1138−1158.
- Swiniarski R. An Application of Rough Sets and Haar Wavelets to Face Recognition // Revised Papers of Second International Conference «Rough Sets and Current Trends in Computing (RSCTC)». 16−19 October 2000. Banff, Canada. Ed. by Ziarko W., Yao Y. Berlin, Heidelberg: Springer Berlin Heidelberg. 2001. P. 561−568.
- Wojcik Z. Rough approximation of shapes in pattern recognition // Computer Vision, Graphics and Image Processing. 1987. V. 40. № 2. P. 228−249.
- Kimachi M., Kanayama K., Teramoto K. Incident prediction by fuzzy image sequence analysis // Proceedings of Vehicle Navigation and Information Systems Conference. 08/1994. P. 51−56.
- Hiremath P.S., Hiremath M.R.M. Face Detection and Trackingin Video Sequence using Fuzzy Geometric Face Model and Motion Estimation // International Journal of Computer Applications. November 2012. V. 58. № 15. P. 12−16.
- Cho J.-S., Yun B.-J., Ko Y.-H. Precision Tracking Based-on Fuzzy Reasoning Segmentation in Cluttered Image Sequences // Proceedings of 9th International Conference «Knowledge-Based Intelligent Information and Engineering Systems (KES)». Melbourne, Australia. 14−16 September 2005. Berlin, Heidelberg: Springer Berlin Heidelberg, 2005 (ed. by Khosla R., Howlett R.J., Jain L.C.). Part II. P. 371−377.
- Pugin E.V., Zhiznyakov A.L. Classification of features of image sequences // International Archives of the Photogrammetry, Remote Sensing and Spatial Information Sciences (ISPRS). May 2015. Т. XL–5/W6. С. 79−81.