350 руб
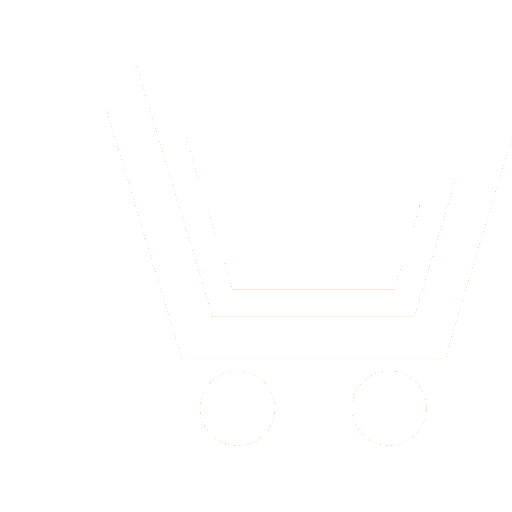
Журнал «Нейрокомпьютеры: разработка, применение» №1 за 2011 г.
Статья в номере:
Булевский факторный анализ на основе аттракторной нейронной сети и некоторые его приложения
Ключевые слова:
булевский факторный анализ
аттракторная нейронная сеть
анализ результатов голосования
задача полосок
синаптические связи
нейродинамика
Авторы:
А. А. Фролов - д. б. н., к. ф.-м. н., зав. лаб «Математическая нейробиология обучения» Института внешней нервной деятельности и нейрофизиологии РАН
Д. Гусек - доктор технической кибернетики, с. н. с. Института Информатики АН Чешской республики, член секции Международной ассоциации вычислительной статистики (IASC) Международного института статистики (ISI)
П. Ю. Поляков - аспирант Научно-исследовательского института системных исследований РАН
Аннотация:
Предложено использовать аттракторную нейронную сеть типа Хопфилда в методе булевского факторного анализа. Демонстрируется эффективность метода в приложении к анализу результатов голосования в Государственной думе РФ и статей, представленных на Международные конференции по нейронным сетям.
Страницы: 25-46
Список источников
- Amit, D. J.,Modeling brain function: The world of attractor neural networks // Cambridge Univ Pr. 1992.
- Barlow, H. B.,Cerebral cortex as model builder // In D. Rose and V. G. Dodson, editors, Models of the visual cortex. P. 37-46. Wiley. Chichester. 1985.
- Belohlavek, R. andVychodil, V., Discovery of optimal factors in binary data via a novel method of matrix decomposition // Journal of Computer and System Sciences, 76(1):3-20. 2010.
- Dempster, A. P., Laird, N. M., andRubin, D. B., Maximum likelihood from incomplete data via the EM algorithm // Journal of the Royal Statistical Society. Series B (Methodological). 39(1):1-38. 1977.
- Doya, K., What are the computations of the cerebellum, the basal ganglia and the cerebral cortex - // Neural networks. 12(7-8):961-974. 1999.
- Foldiak, P.,Forming sparse representations by local anti-hebbian learning. Biological Cybernetics, 64:165 - 170. 1990.
- Frolov, A. A., Husek, D., Muraviev, I. P., and Polyakov, P. Y., Boolean factor analysis by attractor neural network // IEEE Transactions on Neural Networks. 18(3):698-707. 2007.
- Frolov, A. A., Husek, D., Polyakov, P. J., Rezankova, H., and Snasel, V., Binary factorization of textual data by Hopfield-like neural network // In Proc. Computational Statistics (Compstat 04). P. 1035-1041. 2004.
- Frolov, A. A., Husek, D., andPolyakov, P. Y., Recurrent neural network based Boolean factor analysis and its application to automatic terms and documents categorization // IEEE Transactions on Neural Networks. 20(7):1073-1086. 2009.
- Frolov, A. A., Husek, D., andPolyakov, P. Y., Origin and Elimination of Two Global Spurious Attractors in Hopfield-like Neural Network Performing Boolean Factor Analysis. Neurocomputing, 73(7-9): 1394-1404. 2010.
- Frolov, A. A., Sirota, A. M., Husek, D., Muraviev, I. P., and Polyakov, P. J., Binary factorization in Hopfield-like neural networks: single-step approximation and computer simulations. Neural Network Word. 14:139-152. 2004.
- Frolov, A. A., Husek, D., Polyakov, P., and Rezankova, H., New Neural Network Based Approach Helps to Discover Hidden Russian Parliament Voting Patterns // In IEEE International Joint Conference on Neural Networks. P. 6518-6523. 2006.
- Frolov, A. A., Husek, D., Rezankova, H., Snasel, V., and Polyakov, P., Clustering variables by classical approaches and neural network Boolean factor analysis // In IEEE International Joint Conference on Neural Networks. P. 3742-3746. 2008.
- Georgiev, P., Theis, F., andCichocki, A., Sparse component analysis blind source separation of underdetermined mixtures // IEEE Transactions on Neural Networks. 16(4):992-996. 2005.
- Hodge, V. J. and Austin, J.,Hierarchical word clustering - automatic thesaurus generation. Neurocomputing. 48:819-846. 2002.
- Hoppner, F., Klawonn, F., Kruse, R., andRunkler, T., Fuzzy cluster analysis. John Wiley & Sons, New York. 1999.
- Koldovsky, Z., Ticharsky, P., andOja, E., Efficient variant of algorithm fast ICA for independent component analysis attaining the Cramer-Rao lower bound // IEEE Transactions on Neural Networks. 17(5):1265-1277. 2006.
- Kussul, E. M.,Associative neuron-like structures. Naukova Dumka. Kiev. 1992.
- Lucke, J. and Sahani, M., Maximal causes for non-linear component extraction // The Journal of Machine Learning Research. 9:1227-1267. 2008.
- Marr, D., A Theory for Cerebral Neocortex. Proceedings of the Royal Society of London // Series B, Biological Sciences (1934-1990). 176(1043):161-234. 1970.
- Marr, D., Simple Memory: A Theory for Archicortex. Philosophical Transactions of the Royal Society of London. Series B, Biological Sciences (1934-1990). 262(841):23-81. 1971.
- Spratling, M. W., Learning image components for object recognition // Journal of Machine Learning Research. 7:793-815. 2006.
- Zafeiriou, S., Tefas, A., Bucie, I., andPitas, I., Exploiting discriminant information in nonnegative matrix factorization with application to frontal face verification // IEEE Transactions on Neural Networks. 17(3):683-695. 2006.
- http://www.indem.ru/indemstat.
- http://www.publicwhip.org.uk.