350 rub
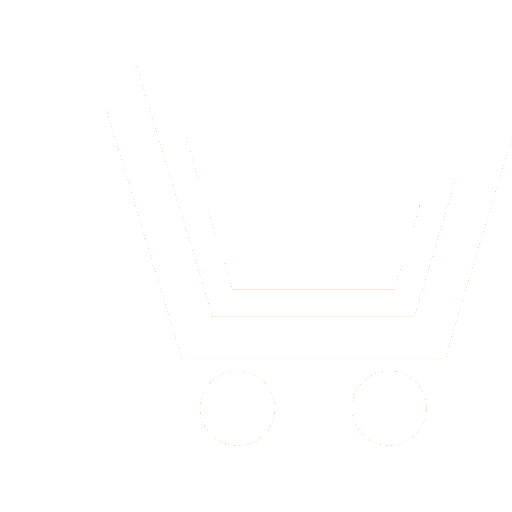
Journal Neurocomputers №1 for 2011 г.
Article in number:
Bulev factorial analysis by means of attractor neural network and its some appendices
Keywords:
Boolean factor analysis
recurrent neural network
Hopfield-like neural network
associative memory
unsupervised learning
Authors:
A. A. Frolov, D. Gusek, P. Yu. Polyakov
Abstract:
The usual problem meeting at the analysis of great volumes of data, search of their adequate representation in space of smaller dimension is. One of the most effective methods used for it is the factorial analysis. In the present work we suppose to use as a method Bulean the factorial analysis attractor of the Hopfield neural network. Features of functioning of an offered neural network speak step by step an example Bulean the factorial analysis is artificial the created data file. Efficiency of a method in the appendix to the analysis of results of voting in the State Dumas of the Russian Federation and is shown to the analysis of clauses presented on the International conferences on neural networks.
Pages: 25-46
References
- Amit, D. J.,Modeling brain function: The world of attractor neural networks // Cambridge Univ Pr. 1992.
- Barlow, H. B.,Cerebral cortex as model builder // In D. Rose and V. G. Dodson, editors, Models of the visual cortex. P. 37-46. Wiley. Chichester. 1985.
- Belohlavek, R. andVychodil, V., Discovery of optimal factors in binary data via a novel method of matrix decomposition // Journal of Computer and System Sciences, 76(1):3-20. 2010.
- Dempster, A. P., Laird, N. M., andRubin, D. B., Maximum likelihood from incomplete data via the EM algorithm // Journal of the Royal Statistical Society. Series B (Methodological). 39(1):1-38. 1977.
- Doya, K., What are the computations of the cerebellum, the basal ganglia and the cerebral cortex - // Neural networks. 12(7-8):961-974. 1999.
- Foldiak, P.,Forming sparse representations by local anti-hebbian learning. Biological Cybernetics, 64:165 - 170. 1990.
- Frolov, A. A., Husek, D., Muraviev, I. P., and Polyakov, P. Y., Boolean factor analysis by attractor neural network // IEEE Transactions on Neural Networks. 18(3):698-707. 2007.
- Frolov, A. A., Husek, D., Polyakov, P. J., Rezankova, H., and Snasel, V., Binary factorization of textual data by Hopfield-like neural network // In Proc. Computational Statistics (Compstat 04). P. 1035-1041. 2004.
- Frolov, A. A., Husek, D., andPolyakov, P. Y., Recurrent neural network based Boolean factor analysis and its application to automatic terms and documents categorization // IEEE Transactions on Neural Networks. 20(7):1073-1086. 2009.
- Frolov, A. A., Husek, D., andPolyakov, P. Y., Origin and Elimination of Two Global Spurious Attractors in Hopfield-like Neural Network Performing Boolean Factor Analysis. Neurocomputing, 73(7-9): 1394-1404. 2010.
- Frolov, A. A., Sirota, A. M., Husek, D., Muraviev, I. P., and Polyakov, P. J., Binary factorization in Hopfield-like neural networks: single-step approximation and computer simulations. Neural Network Word. 14:139-152. 2004.
- Frolov, A. A., Husek, D., Polyakov, P., and Rezankova, H., New Neural Network Based Approach Helps to Discover Hidden Russian Parliament Voting Patterns // In IEEE International Joint Conference on Neural Networks. P. 6518-6523. 2006.
- Frolov, A. A., Husek, D., Rezankova, H., Snasel, V., and Polyakov, P., Clustering variables by classical approaches and neural network Boolean factor analysis // In IEEE International Joint Conference on Neural Networks. P. 3742-3746. 2008.
- Georgiev, P., Theis, F., andCichocki, A., Sparse component analysis blind source separation of underdetermined mixtures // IEEE Transactions on Neural Networks. 16(4):992-996. 2005.
- Hodge, V. J. and Austin, J.,Hierarchical word clustering - automatic thesaurus generation. Neurocomputing. 48:819-846. 2002.
- Hoppner, F., Klawonn, F., Kruse, R., andRunkler, T., Fuzzy cluster analysis. John Wiley & Sons, New York. 1999.
- Koldovsky, Z., Ticharsky, P., andOja, E., Efficient variant of algorithm fast ICA for independent component analysis attaining the Cramer-Rao lower bound // IEEE Transactions on Neural Networks. 17(5):1265-1277. 2006.
- Kussul, E. M.,Associative neuron-like structures. Naukova Dumka. Kiev. 1992.
- Lucke, J. and Sahani, M., Maximal causes for non-linear component extraction // The Journal of Machine Learning Research. 9:1227-1267. 2008.
- Marr, D., A Theory for Cerebral Neocortex. Proceedings of the Royal Society of London // Series B, Biological Sciences (1934-1990). 176(1043):161-234. 1970.
- Marr, D., Simple Memory: A Theory for Archicortex. Philosophical Transactions of the Royal Society of London. Series B, Biological Sciences (1934-1990). 262(841):23-81. 1971.
- Spratling, M. W., Learning image components for object recognition // Journal of Machine Learning Research. 7:793-815. 2006.
- Zafeiriou, S., Tefas, A., Bucie, I., andPitas, I., Exploiting discriminant information in nonnegative matrix factorization with application to frontal face verification // IEEE Transactions on Neural Networks. 17(3):683-695. 2006.
- http://www.indem.ru/indemstat.
- http://www.publicwhip.org.uk.