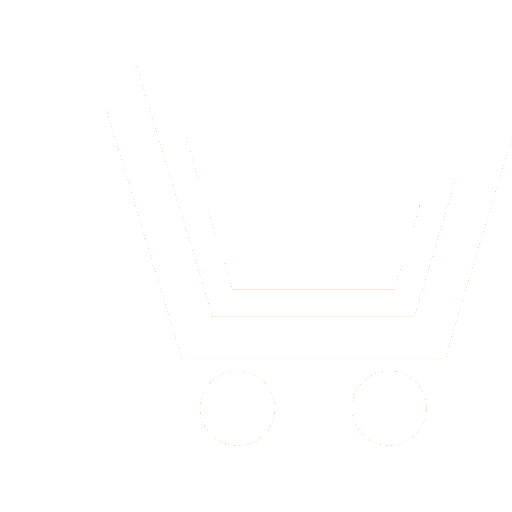
И.А. Левадний1, А.Н. Дмитриев2, С.И. Щукин3
1–3 Московский государственный технический университет им. Н.Э. Баумана (Москва, Россия)
1 levadniyia@student.bmstu.ru, 2 dmitalex@bmstu.ru, 3 schookin@bmstu.ru
Постановка проблемы. Современные методы исследования гемодинамики и функциональной активности головного мозга играют критически важную роль в диагностике и лечении неврологических заболеваний. Выбор подходящего метода или их комбинации позволяет значительно улучшить диагностику, терапевтические стратегии и реабилитационные программы. Сложность и разнообразие методов визуализации требуют систематизации и оценки их эффективности.
Цель. Анализ и сравнение современных неинвазивных инструментальных методов исследования гемодинамики и функциональной активности головного мозга, выявление их ключевых особенностей и ограничений, а также определение перспектив использования мультимодальных подходов в научных исследованиях и клинической практике.
Результаты. Рассмотрены методы, включая электроэнцефалографию (ЭЭГ), реоэнцефалографию (РЭГ), магнитно-резонансную томографию (МРТ), ближнюю инфракрасную спектроскопию (БИКС), позитронно-эмиссионную томографию (ПЭТ), ультразвуковую допплерографию (УЗДГ), радиотермографию и магнитоэнцефалографию (МЭГ). Каждый метод имеет свои преимущества и ограничения, что позволяет их использовать комплексно для исследования мозговой активности и кровообращения. Оценены их временное и пространственное разрешения, чувствительность к глубине залегания источников и глубине проникновения, портативность и возможность длительного мониторирования. Особое внимание уделено мультимодальному подходу и использованию методов в персонализированной медицине.
Практическая значимость. На основе мультимодальных подходов, основанных на комбинации различных методов, таких как ЭЭГ, МРТ и УЗДГ, возможно получать дополнительную информацию о протекании неврологических заболеваний. Внедрение компьютерного моделирования даст возможность выявлять биофизические параметры, отражающие физиологические характеристики.
Левадний И.А., Дмитриев А.Н., Щукин С.И. Неинвазивные инструментальные методы исследования гемодинамики и функциональной активности головного мозга // Биомедицинская радиоэлектроника. 2024. T. 27. № 6. С. 103−121. DOI: https://doi.org/10.18127/ j15604136-202406-10
- Ding C. et al. Global, regional, and national burden and attributable risk factors of neurological disorders: The Global Burden of Dis-ease study 1990–2019. Frontiers in Public Health. 2022. V. 10. P. 952161.
- Dobeson C.B. et al. Perfusion MRI in the evaluation of brain metastases: current practice review and rationale for study of baseline MR perfusion imaging prior to stereotactic radiosurgery (STARBEAM-X). The British Journal of Radiology. 2023. V. 96. № 1152. P. 20220462.
- Chouliaras L., O’Brien J.T. The use of neuroimaging techniques in the early and differential diagnosis of dementia. Mol Psychiatry. 2023. V. 28. № 10. P. 4084–4097.
- Zhou X. et al. Review of recent advances in frequency-domain near-infrared spectroscopy technologies. Biomed. Opt. Express. 2023. V. 14. № 7. P. 3234.
- Lavina B. Brain Vascular Imaging Techniques. IJMS. 2016. V. 18. № 1. P. 70.
- Baranger J. et al. Brain perfusion imaging in neonates. NeuroImage: Clinical. 2021. V. 31. P. 102756.
- Siddiqi S.H. et al. Causal mapping of human brain function. Nat Rev Neurosci. 2022. V. 23. № 6. P. 361–375.
- Zhou J. et al. Fluid metabolic pathways after subarachnoid hemorrhage. Journal of Neurochemistry. 2022. V. 160. № 1. P. 13–33.
- Markram H. The Human Brain Project. Sci Am. 2012. V. 306. № 6. P. 50–55.
- Lorents A. et al. Human Brain Project Partnering Projects Meeting: Status Quo and Outlook. eNeuro. 2023. V. 10. № 9. P. ENEURO.0091-23.2023.
- Elam J.S. et al. The Human Connectome Project: A retrospective. NeuroImage. 2021. V. 244. P. 118543.
- Siva N. What happened to the Human Brain Project? The Lancet. 2023. V. 402. № 10411. P. 1408–1409.
- Fregnac Y. Flagship Afterthoughts: Could the Human Brain Project (HBP) Have Done Better? eNeuro. 2023. V. 10. № 11. P. ENEURO.0428-23.2023.
- Chen Z. et al. Functional connectome of human cerebellum. NeuroImage. 2022. V. 251. P. 119015.
- Yamasaki T. et al. Connectopathy in Autism Spectrum Disorders: A Review of Evidence from Visual Evoked Potentials and Diffusion Magnetic Resonance Imaging. Front. Neurosci. 2017. V. 11. P. 627.
- Kelly J.P. et al. Cerebral Visual Impairment Characterized by Abnormal Visual Orienting Behavior with Preserved Visual Cortical Activation. Invest. Ophthalmol. Vis. Sci. 2021. V. 62. № 6. P. 15.
- Claassen J.A.H.R. et al. Regulation of cerebral blood flow in humans: physiology and clinical implications of autoregulation. Physio-logical Reviews. 2021. V. 101. № 4. P. 1487–1559.
- Lorenz E.A., Su X., Skjæret-Maroni N. A review of combined functional neuroimaging and motion capture for motor rehabilitation. J NeuroEngineering Rehabil. 2024. V. 21. № 1. P. 3.
- High Density EEG – Le Bonheur Children’s Hospital [Electronic resource]. URL: https://www.lebonheur.org/our-services/ neuroscience-institute/advanced-diagnostics-and-testing/high-density-eeg-/ (accessed: 31.07.2024).
- Ghosh R. et al. Automatic Eyeblink and Muscular Artifact Detection and Removal from EEG Signals Using k-Nearest Neighbor Classifier and Long Short-Term Memory Networks. IEEE Sensors J. 2023. V. 23. № 5. P. 5422–5436.
- Chiarion G. et al. Connectivity Analysis in EEG Data: A Tutorial Review of the State of the Art and Emerging Trends. Bioengineering. 2023. V. 10. № 3. P. 372.
- Lu Z. et al. Application of graph frequency attention convolutional neural networks in depression treatment response. Front. Psychiatry. 2023. V. 14. P. 1244208.
- Ardabili S.Z. et al. A Novel Approach for Automatic Detection of Driver Fatigue Using EEG Signals Based on Graph Convolutional Networks. Sensors. 2024. V. 24. № 2. P. 364.
- Yousefi M.R. et al. Comparing EEG-Based Epilepsy Diagnosis Using Neural Networks and Wavelet Transform. Applied Sciences. 2023. V. 13. № 18. P. 10412.
- Gao Y. et al. Deep Convolutional Neural Network-Based Epileptic Electroencephalogram (EEG) Signal Classification. Front. Neurol. 2020. V. 11. P. 375.
- Sun J. et al. OPEN A hybrid deep neural. Scientific Reports.
- Haghayegh S. et al. Automated Sleep Stages Classification Using Convolutional Neural Network from Raw and Time-Frequency Electroencephalogram Signals: Systematic Evaluation Study. J Med Internet Res. 2023. V. 25. P. e40211.
- Tang X. et al. Motor Imagery EEG Decoding Based on Multi-Scale Hybrid Networks and Feature Enhancement. IEEE Trans. Neural Syst. Rehabil. Eng. 2023. V. 31. P. 1208–1218.
- Hjorth B., Rodin E. An eigenfunction approach to the inverse problem of EEG. Brain Topogr. 1988. V. 1. № 2. P. 79–86.
- Grech R. et al. Review on solving the inverse problem in EEG source analysis. J NeuroEngineering Rehabil. 2008. V. 5. № 1. P. 25.
- Michel C.M., He B. EEG source localization. Handbook of Clinical Neurology. Elsevier. 2019. V. 160. P. 85–101.
- Vorwerk J. et al. The multipole approach for EEG forward modeling using the finite element method. NeuroImage. 2019. V. 201. P. 116039.
- Rodionov R.V. Razrabotka algoritmov i programmny`x sredstv dlya lokalizacii istochnikov e`lektricheskoj aktivnosti mozga cheloveka. dis. ... kand. texn. nauk: 05.13.09 – MGTU M., 2000. 179 s.
- He B. et al. Electrophysiological Source Imaging: A Noninvasive Window to Brain Dynamics. Annu. Rev. Biomed. Eng. 2018. V. 20. № 1. P. 171–196.
- Choi J.H., Lee C.O., Jung H.K. An Iterative Distributed Source Method for The Divergence of Source Current in EEG Inverse Problem. Journal of the Korean Society for Industrial and Applied Mathematics. 2008. T. 12. № 3. S. 191–199.
- Baillet S. et al. Evaluation of inverse methods and head models for EEG source localization using a human skull phantom. Phys. Med. Biol. 2001. V. 46. № 1. P. 77–96.
- Fiederer L.D.J. et al. The role of blood vessels in high-resolution volume conductor head modeling of EEG. NeuroImage. 2016. V. 128. P. 193–208.
- Vorwerk J., Wolters C.H., Baumgarten D. Global sensitivity of EEG source analysis to tissue conductivity uncertainties. Front. Hum. Neurosci. 2024. V. 18. P. 1335212.
- Astapenko E.M. Biotexnicheskaya sistema mnogokanal`ny`x e`lektroimpedansny`x issledovanij parametrov gemodinamiki golovnogo mozga: dis... kand. texn. nauk: 05.11.17 – MGTU im. N.E`. Baumana. M., 2012. 130 s.
- Hoang Tin N., Thai Thanh Tam T., Minh Thu P. The noninvasive neuromonitoring in medicine: Rheoencephalography (REG). AJMSM. 2023. V. 11. № 3. P. 67–73.
- Chitturi V., Farrukh N. Spatial resolution in electrical impedance tomography: A topical review. Journal of Electrical Bioimpedance. 2019. V. 8. № 1. P. 66–78.
- Szabo S. et al. Rheoencephalography: A non-invasive method for neuromonitoring. Journal of Electrical Bioimpedance. 2024. V. 15. № 1. P. 10–25.
- Goren N. et al. Data Descriptor: Multi-frequency electrical impedance tomography and neuroimaging data in stroke patients. SCIENTIFIC DATA.
- Wang L. et al. Suppressing interferences of EIT on synchronous recording EEG based on comb filter for seizure detection. Front. Neurol. 2022. V. 13. P. 1070124.
- Bodo M. A noninvasive, continuous brain monitoring method: rheoencephalography (REG). DRCSF. 2020. V. 1. № 2. P. 103–119.
- Bodo M. et al. Correlation of rheoencephalography and laser Doppler flow: a rat study. Journal of Electrical Bioimpedance. 2019. V. 7. № 1. P. 55–58.
- Nacional`ny`j centr biotexnologicheskoj informacii. URL: https://pubmed.ncbi.nlm.nih.gov/ (Data obrashheniya: 05.10.2024).
- McDermott B. et al. Brain Haemorrhage Detection Through SVM Classification of Electrical Impedance Tomography Measurements. Brain and Human Body Modeling / ed. Makarov S., Horner M., Noetscher G. Cham: Springer International Publishing, 2019. P. 211–244.
- Ke X.-Y. et al. Advances in electrical impedance tomography-based brain imaging // Military Med Res. 2022. V. 9. № 1. P. 10.
- Liu X. et al. Fast Iterative Shrinkage-Thresholding Algorithm with Continuation for Brain Injury Monitoring Imaging Based on Electrical Impedance Tomography. Sensors. 2022. V. 22. № 24. P. 9934.
- Guo Y. et al. Electrical impedance tomography provides information of brain injury during total aortic arch replacement through its correlation with relative difference of neurological biomarkers. Sci Rep. 2024. V. 14. № 1. P. 14236.
- Galiamov A.Z. et al. Bioimpedance Spectroscopy for the Postmastectomy Lymphedema Diagnostics. 2020 Ural Symposium on Biomedical Engineering, Radioelectronics and Information Technology (USBEREIT). Yekaterinburg, Russia: IEEE, 2020. P. 0012–0015.
- Kwon D. Brain imaging: fMRI advances make scans sharper and faster. Nature. 2023. V. 617. № 7961. P. 640–642.
- Sturzbecher M.J., de Araujo D.B. Simultaneous EEG-fMRI: Integrating Spatial and Temporal Resolution. The Relevance of the Time Domain to Neural Network Models / ed. Rao A.R., Cecchi G.A. Boston, MA: Springer US, 2012. P. 199–217.
- Matarasso A.K. et al. Combined real-time fMRI and real time fNIRS brain computer interface (BCI): Training of volitional wrist ex-tension after stroke, a case series pilot study. PLoS ONE / ed. Mattia D. 2021. V. 16. № 5. P. e0250431.
- Simon J. et al. Functional Magnetic Resonance Imaging-Based Brain Computer Interfaces. Neural Interface Engineering: Linking the Physical World and the Nervous System / ed. Guo L. Cham: Springer International Publishing. 2020. P. 17–47.
- Bollmann S., Barth M. New acquisition techniques and their prospects for the achievable resolution of fMRI. Progress in Neurobiology. 2021. V. 207. P. 101936.
- Tajik-Parvinchi D. et al. Functional magnetic resonance imaging (fMRI) in typical and atypical brain development: Challenges and suggestions. 2022.
- Wang Z. Arterial Spin Labeling Perfusion MRI Signal Processing Through Traditional Methods and Machine Learning. Investigative magnetic resonance imaging. 2022. V. 26. P. 220–228.
- Chen S. et al. Consistency analysis between 3D arterial spin labeling and dynamic susceptibility contrast perfusion magnetic resonance imaging in perfusion imaging of brain tumor. Nan Fang Yi Ke Da Xue Xue Bao. China, 2021. V. 41. № 8. P. 1283–1286.
- Telischak N., Detre J., Zaharchuk G. Arterial spin labeling MRI: Clinical applications in the brain: Arterial Spin Labeling MRI. Journal of Magnetic Resonance Imaging. 2014. V. 41.
- Hernandez-Garcia L., Lahiri A., Schollenberger J. Recent progress in ASL. NeuroImage. 2019. V. 187. P. 3–16.
- Lindner T. et al. Current state and guidance on arterial spin labeling perfusion MRI in clinical neuroimaging. Magnetic Resonance in Med. 2023. V. 89. № 5. P. 2024–2047.
- ESNR 2018. Neuroradiology. 2018. V. 60. № S2. P. 407–533.
- Thrippleton M.J. et al. Quantifying blood‐brain barrier leakage in small vessel disease: Review and consensus recommendations. Alzheimer’s & Dementia. 2019. V. 15. № 6. P. 840–858.
- Zenaro E., Piacentino G., Constantin G. The blood-brain barrier in Alzheimer’s disease. Neurobiology of Disease. 2017. V. 107. P. 41–56.
- Safonova L.P. et al. Control of cognitive functions using spectrophotometry and evoked potentials. Biomedical Radioelectronics. 2022.
- Mariia B. et al. Personalized Adjustment of Near Infrared Spectroscopy Studies Using Fmri Data for Research in Neuroscience. 2023 Systems and Technologies of the Digital HealthCare (STDH). Tashkent, Uzbekistan: IEEE. 2023. P. 39–42.
- Lange F. Cerebral time-domain near-infrared spectroscopy for clinical use. Frontiers in Biophotonics and Imaging / ed. Mahajan S., Reichelt S. Glasgow, United Kingdom: SPIE. 2021. P. 6.
- Cardim D., Robba C. Principles of Transcranial Doppler Ultrasonography. Echography and Doppler of the Brain / ed. Robba C., Citerio G. Cham: Springer International Publishing, 2021. P. 3–7.
- Demene C. et al. Transcranial ultrafast ultrasound localization microscopy of brain vasculature in patients. Nat Biomed Eng. 2021. V. 5. № 3. P. 219–228.
- Zhou S. et al. Transcranial volumetric imaging using a conformal ultrasound patch. Nature. 2024. V. 629. № 8013. P. 810–818.
- Ali M.F.A. Transcranial Doppler ultrasonography (uses, limitations, and potentials): a review article. Egypt J Neurosurg. 2021. V. 36. № 1. P. 20.
- Lameka K., Farwell M.D., Ichise M. Chapter 11 - Positron Emission Tomography. Handbook of Clinical Neurology / ed. Masdeu J.C., González R.G. Elsevier, 2016. V. 135. P. 209–227.
- Catana C. Development of Dedicated Brain PET Imaging Devices: Recent Advances and Future Perspectives. J Nucl Med. 2019. V. 60. № 8. P. 1044–1052.
- Sollini M. et al. PET imaging in cardiovascular infections. Nuclear Medicine and Molecular Imaging / ed. Signore A. Oxford: Elsevier, 2022. P. 627–655.
- Sedankin M.K. Antenny`-applikatory` dlya radiotermometricheskogo issledovaniya teplovy`x polej vnutrennix tkanej biologicheskogo ob``ekta: avtoref… dis. kan. texn. nauk. M., 2013. 16 p.
- Vesnin S.G. et al. Portable microwave radiometer for wearable devices. Nexo Revista Cientifica. 34. 1431–1447. 10.5377/nexo.v34i04.12690.
- Rodrigues D.B. et al. Microwave Radiometry for Noninvasive Monitoring of Brain Temperature. Emerging Electromagnetic Technologies for Brain Diseases Diagnostics, Monitoring and Therapy / ed. Crocco L. et al. Cham: Springer International Publishing, 2018. P. 87–127.
- Groumpas E. et al. Real-Time Passive Brain Monitoring System Using Near-Field Microwave Radiometry. IEEE Trans. Biomed. Eng. 2020. V. 67. № 1. P. 158–165.
- Karanasiou I.S., Uzunoglu N.K. The Inverse Problem of a Passive Multiband Microwave Intracranial Imaging Method. 2005 IEEE Engineering in Medicine and Biology 27th Annual Conference. Shanghai, China: IEEE. 2005. P. 1642–1645.
- Koutsoupidou M. et al. The effect of using a dielectric matching medium in focused microwave radiometry: an anatomically detailed head model study. Med Biol Eng Comput. 2018. V. 56. № 5. P. 809–816.
- Gudkov A.G. i dr. Ispol`zovanie metoda mnogokanal`noj mikrovolnovoj radiometrii dlya funkcional`noj diagnostiki golovnogo mozga. Medicinskaya texnika. 2019. № 2. P. 22–25.
- Barratt E.L. et al. Mapping the topological organisation of beta oscillations in motor cortex using MEG. NeuroImage. 2018. V. 181. P. 831–844.
- Fedosov N., Shevtsov O., Ossadtchi A. Motor-Imagery BCI with Low-Count of Optically Pumped Magnetometers. 2021 Third International Conference Neurotechnologies and Neurointerfaces (CNN). Kaliningrad, Russian Federation: IEEE. 2021. P. 16–18.
- Malmivuo J., Plonsey R. Bioelectromagnetism Principles and Applications of Bioelectric and Biomagnetic Fields. Oxford University Press, 1995.
- Maslennikov Yu.V. Magnitometricheskie sistemy` na osnove SKVIDov dlya biomedicinskix primenenij: avtoref… dis. d-ra texn. nauk. M., 2016. 32 s.
- Rawnaque F.S. et al. Technological advancements and opportunities in Neuromarketing: a systematic review. Brain Inf. 2020. V. 7. № 1. P. 10.
- Ng A., Swanevelder J. Resolution in ultrasound imaging. Continuing Education in Anaesthesia Critical Care & Pain. 2011. V. 11. № 5. P. 186–192.
- Lishhuk V.A., Bokeriya L.A. Matematicheskie modeli i metody` v intensivnoj terapii: sorokaletnij opy`t. K 50-letiyu NCzSSX im. A.N. Bakuleva RAMN. Ch. 3. 1996–2006 gody`. Klinicheskaya fiziologiya krovoobrashheniya. 2006. № 4. S. 12–25.
- Chen T. et al. A computer-aided diagnosis system for brain tumors based on artificial intelligence algorithms. Front. Neurosci. 2023. V. 17. P. 1120781.
- Tixomirov A.N. Komp`yuternaya sistema mnogokanal`noj e`lektroimpedansnoj kardiografii: dis... kand. texn. nauk: 2.2.12. M. 2021. 102 s.