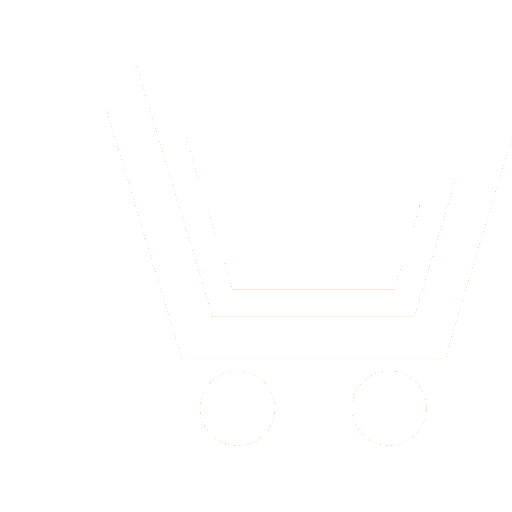
Б.И. Исмайлов1
1 Азербайджанский государственный университет нефти и промышленности (г. Баку, Азербайджан)
Постановка проблемы. Современная медицина интенсивно увеличивает парк измерительной и диагностической аппаратуры. Среди современных методов анализа измерительной и визуальной информации можно отметить применение искусственного интеллекта и его раздела «глубокое обучение» для диагностики сложных заболеваний. Компьютеризированная диагностика заболевания, используя измерительные, визуальные, сложно формализуемые симптомы и скрытые признаки заболеваний с помощью глубокого обучения и алгоритмов рекуррентных нейронных сетей, позволит оказать поддержку при постановке диагноза заболевания и выбрать соответствующий режим управления работой аппарата поддержания искусственного дыхания.
Цель работы – применение современных методов решения проблемы диагностики респираторных заболеваний пациентов с подозрением на инфицированность вирусом COVID-19 и разработка алгоритмов и методов диагностики и принятия обоснованных решений для управления режимами работы технических средств искусственной вентиляции легких, для больных, инфицированных вирусом COVID-19 и другими респираторными заболеваниями.
Результаты. Обработка измерительной информации и отображение диагностических данных для решения задач диагностики позволят сократить время принятия решения благодаря использованию современных методов, таких как визуальное мышление. Сочетая опыт, интуицию врача и возможности визуального мышления по аргументации проявлений тех или иных болезненных проявлений и возможности по выявлению скрытых закономерностей, предложенная диагностическая система позволит сократить процесс принятия обоснованного решения.
Проведённый обзор достижений медицинской диагностики подтвердил правильность выбора предложенного метода – применение искусственного интеллекта для анализа, оценки, постановки диагноза и на основе этих знаний управление режимами работы аппаратной части системы поддержки дыхания.
Практическая значимость. Предложенные алгоритмы поиска закономерностей, влияний и проявлений симптомов заболевания позволит систематизировать диагностические критерии, исключить человеческий фактор при обработке большого объёма измерительно-диагностической информации. Анализ индивидуальных респираторных и физиологических показателей с учётом феномена памяти (имеется в виду история болезни, реакция организма на проведённую терапию и хроника проявления недуга) позволит предложить наиболее приемлемый режим работы аппарата поддержания искусственного дыхания.
Исмайлов Б.И. Глубокое обучение в медицинской диагностике и контроле функций системы искусственного дыхания // Биомедицинская радиоэлектроника. 2023. Т. 26. № 1. С. 5-17. DOI: https://doi.org/10.18127/j15604136-202301-01
- Poincaré H. Sur la problem des trois corps et les équations de la dynamique // Acta Mathematica. 1890. V. 13. P. 1–271. https://projecteuclid.org/journals/acta-mathematica/volume-13/issue-1-2
- Mangiarotti S., Peyre M., Zhang Y., Huc M., Roger F., Kerr Y. Chaos theory applied to the outbreak of COVID19: an ancillary approach to decision making in pandemic context. Epidemiology and Infection. 2020. Cambridge University Press. 9 p. https://doi.org/10.1017/S0950268820000990
- Borah M., Gayan A., Sharma J.S., Chen Y.G., Wei Z., Pham V-T. Is fractional-order chaos theory the new tool to model chaotic pandemics as Covid-19? // Nonlinear Dynamics. 2022. 29 p. https://doi.org/10.1007/s11071-021-07196-3
- Sprott J.C. Chaos and Time Series Analysis. Oxford; New York: Oxford University Press. 2003. 507 p. https://www.worldcat.org/title/chaos-and-time-series-analysis/oclc/493261461
- Vladimirsky E.I., Ismailov B.I. Synergetic methods of control of chaotic systems. Baku: "ELM". 2011. 240 p. https://sng1lib.org/book/3198414/966b89?id=3198414&secret=966b89
- Kafraj M.S., Nazarimehr F., Ghosh D., Rajagopal K., Jafari S., Sprott J.C. Effects of Amplitude, Maximal Lyapunov Exponent, and Kaplan–Yorke Dimension of Dynamical Oscillators on Master Stability Function // International Journal of Bifurcation and Chaos. 2022. V. 32(05). https://doi.org/10.1142/S0218127422500675
- Eckman J., Kamphorst S., Ruelle D. Recurrence Plots of Dynamical Systems // Europhysics Letters. 1987. V. 4 (9). P. 973–977. https://iopscience.iop.org/article/10.1209/0295-5075/4/9/004/meta
- Vladimirsky E.I., Ismailov B.I. Transient and recurrence processes in open system // International Journal of Advanced and Applied Sciences (IJAAS). 2017. V. 4(10). P. 106–115. https://doi.org/10.21833/ijaas.2017.010.015
- Ismailov B. Hidden Oscillations in Fractional-order Multidimensional Chaotic Systems // Journal of “Advances in Research”, SCIENCEDOMAIN international. 2016. V. 8(2). P. 1–5. https://doi.org/10.9734/AIR/2016/28044
- Ferrara V., Santis S, De, Melchiori F.M. Art for improving skills in medical education: the validation of a scale for measuring the Visual Thinking Strategies method // Clin. Ter. 2020. V. 171 (3). P. e253–259. https://doi.org/10.7417/CT.2020.2223
- Zhou Xi., Ye Q., Yang X., Chen J., Ma H., Xia J., Ser J.D., Yang G. AI-based Medical e-Diagnosis for Fast and Automatic Ventricular Volume Measurement in the Patients with Normal Pressure Hydrocephalus. 2022. https://doi.org/10.48550/arXiv.2202.00650
- Hendrickson M.A., Seo Y., Hokanson B. Evaluating the Effectiveness of Visual Thinking Strategies // Proceedings of the AECT 2019 Convention. 2019. P. 92–101. https://www.semanticscholar.org/paper/evaluating-the-effectiveness-of-visual-thinking-hendrickson/ 8eabcc160dcb42ab470145ac6101245b12fad853
- Stokes K. Castaldo R., Federici C., Pagliara S., Maccaro A., Cappuccio F., Fico G., Salvatore M., Franzese M., Pecchia L. The use of artificial intelligence systems in diagnosis of pneumonia via signs and symptoms: A systematic review // Biomedical Signal Processing and Control. 2022. V. 72. 103325. 14 p. https://doi.org/10.1016/j.bspc.2021.103325
- Keogh F.M., Lee A., Gibbon F. Visual Thinking Strategies: Experiences of an Arts based Curriculum in an Irish University Medicine and Health Faculty // All Ireland Journal of Higher Education. 2020. V. 12. № 1. P. 24. http://ojs.aishe.org/index.php/aishe-j/article/view/408
- Faris H., Habib M., Faris M, Elayan H, Alomari A. An intelligent multimodal medical diagnosis system based on patients’ medical questions and structured symptoms for telemedicine // Informatics in Medicine Unlocked. 2021. V. 23. 100513. https://doi.org/10.1016/ j.imu.2021.100513
- Georgiou A., Li J., Hardie R-A., Wabe N., Horvath A.R., Post J.J., Eigenstetter A., Lindeman R., Lam Q., Badrick T., Pearce C. Diagnostic Informatics — The Role of Digital Health in Diagnostic Stewardship and the Achievement of Excellence, Safety, and Value // Frontiers in Digital Health. 2021. https://doi.org/10.3389/fdgth.2021.659652
- Manera M. Perspectives on Complexity, Chaos and Thermodynamics in Environmental Pathology // IJERPH. 2021. V. 18. Iss. 11. P. 11. https://doi.org/10.3390/ijerph18115766
- Toker D., Sommer F.T., D’Esposito M. A simple method for detecting chaos in nature // Communication Biology. 2020. 13 p. http://doi.org/10.1038/s42003-019-0715-9
- Vladimirsky E.I., Ismailov B.I. Physics of Open System. Non-standard approaches in the context of studies of multidimensional coupled chaotic systems of fractional order // International Conference on Recent Innovations in Electrical, Electronics & Communication Engineering (ICRIEECE), IEEE Bhubaneswar Subsection. India. 2018. P. 229–230. http://doi.org/10.1109/ICRIEECE44171.2018
- Ismailov B.I. Thermodynamic – Informational Paradigm in the Context of the Formation of a Mathematical Model of Transient Processes in an Open System // European Journal of Engineering Research and Science. 2017. V. 2. № 10. P. 17–20. https://doi.org/10.24018/ejers.2017.2.10.494
- Dondorp A.M., Hayat M., Aryal D., Beane A., Schultz M.J. Respiratory Support in COVID-19 Patients, with a Focus on Resource-Limited Settings // The American Journal of Tropical Medicine and Hygiene. 2020. V. 102. Iss. 6. P. 1191–1197. https://doi.org/ 10.4269/ajtmh.20-0283
- Mayr E., Diamond J. What Evolution Is. Basic Books. 2002. 318 p. http://www.goodreads.com/book/show/602988. What_Evolution_Is
- Dwivedi K., Sharkey M., Condliffe R., Uthoff J.M., Alabed S., Metherall P., Lu H., Wild Jim M, Hoffman E. A., Swift A.J., Kiely D.G. Pulmonary Hypertension in Association with Lung Disease: Quantitative CT and Artificial Intelligence to the Rescue? State-of-the-Art Review // Diagnostics. 2021. V. 11. 679. 20 p. https://doi.org/10.3390/diagnostics11040679
- Attaway A.H., Scheraga R.G., Bhimraj A., Biehl M., Hatipoğlu U. Severe covid-19 pneumonia: pathogenesis and clinical management // BMJ. 2021. V. 372. 19 p. https://doi.org/10.1136/bmj.n436
- Berlin D.A., R.M. Gulick R.M., Martinez F.J. Severe Covid-19 // The new England journal of medicine. 2020. V. 383. № 25.
P. 2451–2460. https://doi.org/10.1056/NEJMcp2009575 - Munshi L., Hall J.B. Respiratory Support During the COVID-19 Pandemic // JAMA. 2021. V. 325(17). P. 1723–1725. https://doi.org/ 10.1001/jama.2021.4975
- Haleema A., Javaid M., Singh R.P., Suman R. Applications of Artificial Intelligence (AI) for cardiology during COVID-19 pandemic // Sustainable Operations and Computers. 2021. V. 2. P. 71–78. https://doi.org/10.1016/j.susoc.2021.04.003
- Gurzhin S.G., Nguyen V.L. Implementation of a non-contact method for monitoring the processes of breathing and heartbeat of a patient during a magnetic therapy session // Journal Biomedical Radioelectronics. 2021. № 4. P. 23-32. https://doi.org/10.18127/ j15604136-202104-04
- Sazykina L.V., Gazizova D.Sh., Nishonov N.A., Makoveev S.N., Kofranek I., Shevchenko G.V. Application of the thermodilution method to assess the hemodynamics of patients with pulmonary hypertension // Journal Biomedical Radioelectronics. 2020. № 5. P. 5–17. https://doi.org/10.18127/j15604136-202005-01
- Wolfa Y.I., Katsnelsonb M.I., Koonin E.V. Physical foundations of biological complexity // PNAS. 2018. V. 115. № 37. E8678–E8687. https://doi.org/10.1073/pnas.1807890115
- Kirillov S.N., Mamushev D.Yu. Remote diagnosis of diseases of the upper respiratory tract based on the analysis of speech signal parameters // Journal Biomedical Radioelectronics. 2020. № 3. P. 68–74. https://doi.org/10.18127/j15604136-202003-09
- Aziz M.M., Jihad O.M. Stability, Chaos Diagnose and Adaptive Control of Two Dimensional Discrete - Time Dynamical System // Open Access Library Journal. 2021. V. 8. № 3. https://doi.org/10.4236/oalib.1107270
- Jackson T., Radunskaya A. Applications of Dynamical Systems in Biology and Medicine. Springer Science+Business Media, LLC. 2015. 240 p. https://doi.org/10.1007/978-1-4939-2782-1
- Majumdar K., Jayachandran S. A Geometric Analysis of Time Series Leading to Information Encoding and A New Entropy Measure. 2018. chrome-extension://mhjfbmdgcfjbbpaeojofohoefgiehjai/index.html
- Gharajedaghi J. Systems Thinking. Managing Chaos and Complexity. Elsevier. 2006. 357 p. chrome-extension: //mhjfbmdgcfjbbpaeojofohoefgiehjai/index.html
- Wegenkittl R., Loffelmann H., Groller E. Visualizing the Behavior of Higher Dimensional Dynamical Systems // IEEE Xplore. 2002. http://dx.doi.org/10.1145/266989.267038
- Jiarui Li., Sawaragi T., Horiguchi Y. Introduce structural equation modelling to machine learning problems for building an explainable and persuasive model // Sice Journal of Control, Measurement, and System Integration. 2021. V. 14. № 2. P. 67–79. https://doi.org/10.1080/18824889.2021.1894040
- Gunzler D.D., Perzynski A.T., Carle A.C. Structural Equation Modeling for Health and Medicine. 2021. 322 p. https://doi.org/10.1201/ 9780203701133
- Hobbs B., Ord A. Nonlinear dynamical analysis of GNSS data: quantification, precursors and synchronization // Progress in Earth and Planetary Science. 2018. 35 p. https://progearthplanetsci.springeropen.com/articles/10.1186/s40645-018-0193-6
- What are Neural Networks? IBM Cloud Education. 2020. https://www.ibm.com/cloud/learn/neural-networks
- Barzegar V., Laflamme S., Hu C., Dodson J. Ensemble of recurrent neural networks with long short-term memory cells for high-rate structural health monitoring // Mechanical Systems and Signal Processing. 2022. V. 164. 108201. https://doi.org/10.1016/ j.ymssp.2021.108201
- Daitche A., Tél T. Memory effects in chaotic advection of inertial particles // New Journal of Physics. 2014. V. 16. 31 p. 073008. https://doi.org/10.1088/1367-2630/16/7/073008
- Tobore I., Li J., Yuhang L., Al-Handarish Y., Kandwal A., Nie Z., Wang L. Deep Learning Intervention for Health Care Challenges: Some Biomedical Domain Considerations // JMIR mHealth and uHealth. 2019. V. 7. № 8. http://dx.doi.org/10.2196/11966