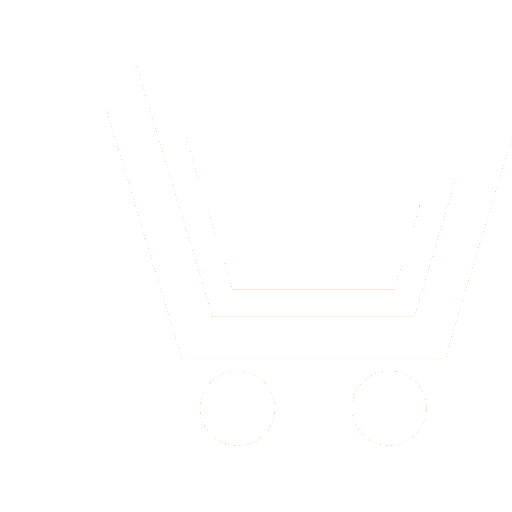
B.I. Ismailov1
1 Azerbaijan State University of Oil and Industry (Baku, Azerbaijan)
Formulation of the problem. Modern medicine is intensively increasing the fleet of measuring and diagnostic equipment. Among the modern methods of analysis of measuring and visual information, one can note the use of Artificial Intelligence and its Deep Learning section for the diagnosis of complex diseases. Computerized diagnosis of the disease using measuring, visual, difficult to formalize symptoms and hidden signs of diseases using Deep Learning and Recurrent Neural Network algorithms will provide support in the diagnosis of the disease, and select the appropriate control mode for the operation of the artificial respiration apparatus.
Objective – application of modern methods for solving the problem of diagnosing respiratory diseases in patients with suspected infection with the COVID-19 virus. Development of algorithms and methods for diagnosing and making informed decisions to control the operating modes of mechanical ventilation equipment for patients infected with the COVID-19 virus and other respiratory diseases.
Results. Processing measurement information and displaying diagnostic data to solve diagnostic problems will reduce decision time through the use of modern methods such as Visual Thinking. Combining the experience, intuition of the doctor and the possibilities of Visual Thinking in arguing the manifestations of certain painful manifestations and the ability to identify hidden patterns, the proposed diagnostic system will shorten the process of making an informed decision.
The review of the achievements of medical diagnostics confirmed the correctness of the choice of the proposed method - the use of artificial intelligence for analysis, evaluation, diagnosis and, based on this knowledge, control of the operating modes of the respiratory support system hardware.
Practical significance. The proposed algorithms for searching for patterns, influences and manifestations of symptoms of the disease will make it possible to systematize diagnostic criteria, eliminate the human factor when processing a large amount of measurement and diagnostic information. An analysis of individual respiratory and physiological parameters, taking into account the phenomenon of memory, meaning the history of the disease, the reaction of the body to the therapy and the chronicle of the manifestation of the disease, will allow us to offer the most acceptable mode of operation of the artificial respiration apparatus.
Ismailov B.I. Deep learning in medical diagnostics and control of the functions of the artificial respiration system. Biomedicine Radio-engineering. 2023. V. 26. № 1. P. 5-17. DOI: https://doi.org/10.18127/j15604136-202301-01
- Poincaré H. Sur la problem des trois corps et les équations de la dynamique. Acta Mathematica. 1890. V. 13. P. 1–271. https://projecteuclid.org/journals/acta-mathematica/volume-13/issue-1-2
- Mangiarotti S., Peyre M., Zhang Y., Huc M., Roger F., and Kerr Y. Chaos theory applied to the outbreak of COVID19: an ancillary approach to decision making in pandemic context. Epidemiology and Infection. 2020. Cambridge University Press. 9 p. https://doi.org/10.1017/S0950268820000990
- Borah M., Gayan A., Sharma J.S., Chen Y.G., Wei Z., Pham V-T. Is fractional-order chaos theory the new tool to model chaotic pandemics as Covid-19? Nonlinear Dynamics. 2022. 29 p. https://doi.org/10.1007/s11071-021-07196-3
- Sprott J.C. Chaos and Time Series Analysis. Oxford; New York: Oxford University Press. 2003. 507 p. https://www.worldcat.org/title/chaos-and-time-series-analysis/oclc/493261461
- Vladimirsky E.I., Ismailov B.I. Synergetic methods of control of chaotic systems. Baku, "ELM". 2011. 240 p. https://sng1lib.org/book/3198414/966b89?id=3198414&secret=966b89
- Kafraj M.S., Nazarimehr F., Ghosh D., Rajagopal K., Jafari S., Sprott J.C. Effects of Amplitude, Maximal Lyapunov Exponent, and Kaplan–Yorke Dimension of Dynamical Oscillators on Master Stability Function. International Journal of Bifurcation and Chaos. 2022. V. 32(05). https://doi.org/10.1142/S0218127422500675
- Eckman J., Kamphorst S., Ruelle D. Recurrence Plots of Dynamical Systems. Europhysics Letters. 1987. V. 4 (9). P. 973–977. https://iopscience.iop.org/article/10.1209/0295-5075/4/9/004/meta
- Vladimirsky E.I., Ismailov B.I. Transient and recurrence processes in open system. International Journal of Advanced and Applied Sciences (IJAAS). 2017. V. 4(10). P. 106–115. https://doi.org/10.21833/ijaas.2017.010.015
- Ismailov B. Hidden Oscillations in Fractional-order Multidimensional Chaotic Systems. Journal of “Advances in Research”, SCIENCEDOMAIN international. 2016. V. 8(2). P. 1–5. https://doi.org/10.9734/AIR/2016/28044
- Ferrara V., Santis S, De, Melchiori F. M. Art for improving skills in medical education: the validation of a scale for measuring the Visual Thinking Strategies method. Clin. Ter. 2020. V. 171 (3). P. e253–259. https://doi.org/10.7417/CT.2020.2223
- Zhou Xi., Ye Q., Yang X., Chen J., Ma H., Xia J., Ser J.D., Yang G. AI-based Medical e-Diagnosis for Fast and Automatic Ventricular Volume Measurement in the Patients with Normal Pressure Hydrocephalus. 2022. https://doi.org/10.48550/arXiv.2202.00650
- Hendrickson M.A., Seo Y., and Hokanson B. Evaluating the Effectiveness of Visual Thinking Strategies. Proceedings of the AECT 2019 Convention. P. 92–101. https://www.semanticscholar.org/paper/evaluating-the-effectiveness-of-visual-thinking-hendrickson/8eabcc160dcb42ab470145ac6101245b12fad853
- Stokes K. Castaldo R., Federici C., Pagliara S., Maccaro A., Cappuccio F., Fico G., Salvatore M., Franzese M., and Pecchia L. The use of artificial intelligence systems in diagnosis of pneumonia via signs and symptoms: A systematic review. Biomedical Signal Processing and Control. 2022. V. 72. 103325. 14 p. https://doi.org/10.1016/j.bspc.2021.103325
- Keogh F.M., Lee A., and Gibbon F. Visual Thinking Strategies: Experiences of an Arts based Curriculum in an Irish University Medicine and Health Faculty. All Ireland Journal of Higher Education. 2020. V. 12. № 1. 24 p. http://ojs.aishe.org/index.php/aishe-j/article/view/408
- Faris H., Habib M., Faris M, Elayan H, Alomari A. An intelligent multimodal medical diagnosis system based on patients’ medical questions and structured symptoms for telemedicine. Informatics in Medicine Unlocked. 2021. V. 23. 100513. https://doi.org/10.1016/j.imu.2021.100513
- Georgiou A., Li J., Hardie R-A., Wabe N., Horvath A.R., Post J.J., Eigenstetter A., Lindeman R., Lam Q., Badrick T., Pearce C. Diagnostic Informatics — The Role of Digital Health in Diagnostic Stewardship and the Achievement of Excellence, Safety, and Value. Frontiers in Digital Health. 2021. https://doi.org/10.3389/fdgth.2021.659652
- Manera M. Perspectives on Complexity, Chaos and Thermodynamics in Environmental Pathology. IJERPH. 2021. V. 18. Iss. 11. 11 p. https://doi.org/10.3390/ijerph18115766
- Toker D., Sommer F.T., D’Esposito M. A simple method for detecting chaos in nature. Communication Biology. 2020. 13 p. http://doi.org/10.1038/s42003-019-0715-9
- Vladimirsky E.I., Ismailov B.I. Physics of Open System. Non-standard approaches in the context of studies of multidimensional coupled chaotic systems of fractional order. International Conference on Recent Innovations in Electrical, Electronics & Communication Engineering (ICRIEECE), IEEE Bhubaneswar Subsection. India. 2018. P. 229–230. http://doi.org/10.1109/ICRIEECE44171.2018
- Ismailov B.I. Thermodynamic – Informational Paradigm in the Context of the Formation of a Mathematical Model of Transient Processes in an Open System. European Journal of Engineering Research and Science. 2017. V. 2. № 10. P. 17–20. https://doi.org/10.24018/ejers.2017.2.10.494
- Dondorp A.M., Hayat M., Aryal D., Beane A., and Schultz M.J. Respiratory Support in COVID-19 Patients, with a Focus on Resource-Limited Settings. The American Journal of Tropical Medicine and Hygiene. 2020. V. 102. Iss. 6. P. 1191–1197. https://doi.org/10.4269/ajtmh.20-0283
- Mayr E., Diamond J. What Evolution Is. Basic Books. 2002. 318 p. http://www.goodreads.com/book/show/602988. What_Evolution_Is
- Dwivedi K., Sharkey M., Condliffe R., Uthoff J.M., Alabed S., Metherall P., Lu H., Wild Jim M, Hoffman E. A., Swift A.J., and Kiely D.G. Pulmonary Hypertension in Association with Lung Disease: Quantitative CT and Artificial Intelligence to the Rescue? State-of-the-Art Review. Diagnostics. 2021. V. 11. 679. 20 p. https://doi.org/10.3390/diagnostics11040679
- Attaway A.H., Scheraga R.G., Bhimraj A., Biehl M., Hatipoğlu U. Severe covid-19 pneumonia: pathogenesis and clinical management. BMJ. 2021. V. 372. 19 p. https://doi.org/10.1136/bmj.n436
- Berlin D.A., R.M. Gulick R.M., and Martinez F.J. Severe Covid-19. The new England journal of medicine. 2020. V. 383. № 25. P. 2451–2460. https://doi.org/10.1056/NEJMcp2009575
- Munshi L., Hall J.B. Respiratory Support During the COVID-19 Pandemic. JAMA. 2021. V. 325(17). P. 1723–1725. https://doi.org/10.1001/jama.2021.4975
- Haleema A., Javaid M., Singh R.P., Suman R. Applications of Artificial Intelligence (AI) for cardiology during COVID-19 pandemic. Sustainable Operations and Computers 2021. V. 2. P. 71–78. https://doi.org/10.1016/j.susoc.2021.04.003
- Gurzhin S.G., Nguyen V.L. Implementation of a non-contact method for monitoring the processes of breathing and heartbeat of a patient during a magnetic therapy session. Journal Biomedical Radio Electronics. 2021. № 4. P. 23-32. https://doi.org/10.18127/j15604136-202104-04
- Sazykina L.V., Gazizova D.Sh., Nishonov N.A., Makoveev S.N., Kofranek I., Shevchenko G.V. Application of the thermodilution method to assess the hemodynamics of patients with pulmonary hypertension. Journal Biomedical Radio Electronics. 2020. № 5. P. 5–17. https://doi.org/10.18127/j15604136-202005-01
- Wolfa Y.I., Katsnelsonb M.I., and Koonin E.V. Physical foundations of biological complexity. PNAS. 2018. V. 115. № 37. E8678–E8687. https://doi.org/10.1073/pnas.1807890115
- Kirillov S.N., Mamushev D.Yu. Remote diagnosis of diseases of the upper respiratory tract based on the analysis of speech signal parameters. Journal Biomedical Radio Electronics. 2020. № 3. P. 68–74. https://doi.org/10.18127/j15604136-202003-09
- Aziz M.M., O.M. Jihad O.M. Stability, Chaos Diagnose and Adaptive Control of Two Dimensional Discrete - Time Dynamical System. Open Access Library Journal. 2021. V. 8. № 3. https://doi.org/10.4236/oalib.1107270
- Jackson T., Radunskaya A. Applications of Dynamical Systems in Biology and Medicine. Springer Science+Business Media, LLC. 2015. 240 p. https://doi.org/10.1007/978-1-4939-2782-1
- Majumdar K., Jayachandran S. A Geometric Analysis of Time Series Leading to Information Encoding and A New Entropy Measure. 2018. chrome-extension://mhjfbmdgcfjbbpaeojofohoefgiehjai/index.html
- Gharajedaghi J. Systems Thinking. Managing Chaos and Complexity. Elsevier. 2006. 357 p. chrome-extension: //mhjfbmdgcfjbbpaeojofohoefgiehjai/index.html
- Wegenkittl R., Loffelmann H., and Groller E. Visualizing the Behavior of Higher Dimensional Dynamical Systems. IEEE Xplore. 2002. http://dx.doi.org/10.1145/266989.267038
- Jiarui Li., Sawaragi T., and Horiguchi Y. Introduce structural equation modelling to machine learning problems for building an explainable and persuasive model. Sice Journal of Control, Measurement, and System Integration. 2021. V. 14. № 2. P. 67–79. https://doi.org/10.1080/18824889.2021.1894040
- Gunzler D.D., Perzynski A.T., Carle A.C. Structural Equation Modeling for Health and Medicine. 2021. 322 p. https://doi.org/10.1201/9780203701133
- Hobbs B., and Ord A. Nonlinear dynamical analysis of GNSS data: quantification, precursors and synchronization. Progress in Earth and Planetary Science. 2018. 35 p. https://progearthplanetsci.springeropen.com/articles/10.1186/s40645-018-0193-6
- What are Neural Networks? IBM Cloud Education. 2020. https://www.ibm.com/cloud/learn/neural-networks
- Barzegar V., Laflamme S., Hu C., Dodson J. Ensemble of recurrent neural networks with long short-term memory cells for high-rate structural health monitoring. Mechanical Systems and Signal Processing. 2022. V. 164. 108201. https://doi.org/10.1016/j.ymssp.2021.108201
- Daitche A., Tél T. Memory effects in chaotic advection of inertial particles. New Journal of Physics. 2014. V. 16. 31 p. 073008. https://doi.org/10.1088/1367-2630/16/7/073008
- Tobore I., Li J., Yuhang L., Al-Handarish Y., Kandwal A., Nie Z., and Wang L. Deep Learning Intervention for Health Care Challenges: Some Biomedical Domain Considerations. JMIR mHealth and uHealth. 2019. V. 7. № 8. http://dx.doi.org/10.2196/11966