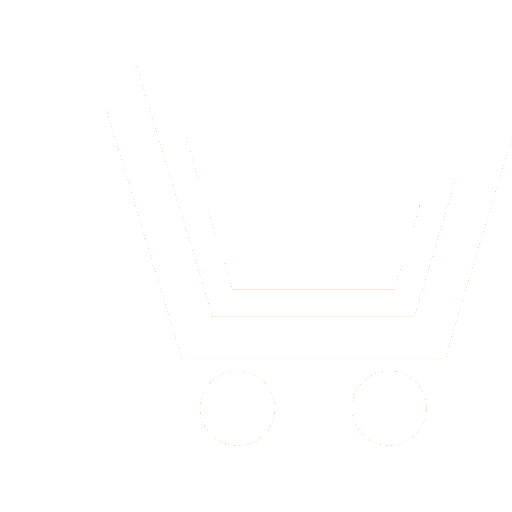
O. S. Litvinov – Dr.Sc. (Phys.-Math.), Professor, Bauman Moscow State Technical University
E-mail: oleglitv@mtu-net.ru
A. N. Zabelin – Student,
E-mail: z307s@yandex.ru
B. E. Vintaykin – Dr.Sc. (Phys.-Math.), Professor,
Bauman Moscow State Technical University
E-mail: vintaikb@mail.ru
V. S. Boruta – Ph.D. (Phys.-Math.), Associate Professor, Bauman Moscow State Technical University
E-mail: borutav@mail.ru
Adaptive antenna arrays (AAA) can be used for optimal signal receiving to counteraction of electromagnetic interference in modern radioelectronic means. The use of the neural network control algorithm in AAA provides the universality of application and solution of a wider class of tasks. The application of discussed adaptation algorithms is possible in communication systems, radar, radio navigation and other areas where it is necessary to improve the quality of received signals.
Reception of a useful signal is simulated against the background of broadband interference, coming from different angular directions. In a mathematical description, a wideband interference is represented as a set of equivalent, spaced-out narrowband interferences. In the process of suppression such interferences, significant attenuation of the useful signal is possible and carrier-to-noise-andinterference ratio (CNIR) due to one of equivalent spatially separated interference in the main lobe radiation patterns.
The main task of AAA design is development of weight-coefficient (WC) control block which adjusts amplitude attenuation and phase shift of each receiving channel in order to maximize signal to noise ratio of resulting signal. The article presents a comparison of the neural network amplitude-phase algorithm for controlling AAA and the traditional control algorithm with criteria of maximizing CNIR based direct matrix inversion. The selected criterion provides fast and deep reducing of interference, it is used for weak and short time signals.
The neural network algorithm is based on the application of a multilayered neural network of direct propagation. To train the network, in this paper we used a teacher-based learning algorithm – the error back propagation algorithm.
The training dataset is composed of a set of vectors of the total input signal and set of WC vectors obtained during the operation of the traditional adaptation algorithm. For the simulation of test signal-interference situation used a narrowband and wideband interference arriving from different angular directions. In this paper, we selected a linear equidistant AAA with non-directional elements and 10 complex WC. For the neural network, we chose a configuration consisting of an input and output layer with a size of 20 elements, and two hidden layers containing 20 neurons each. The signal and interference had the same amplitude, they came to the antenna in same phase and without phase noise. The algorithms have been modeled in the Matlab environment with Neural Network Toolbox extension package.It has been shown that the neural network algorithm of amplitude-phase control of AAA provides better efficiency of noise suppression in the frequency band of more than 5%, in comparison with the traditional algorithm, and is not inferior to the traditional algorithm in the degree of preservation of the useful signal power at the output of AAA. The use of a neural network provides the versatility of the algorithm for various scenarios of the signal-interference environment.
- Pistol'kors A.A., Litvinov O.S. Vvedenie v teoriyu adaptivnykh antenn. M.: Nauka. 1991. S. 5, 12–40. (in Russian)
- Monzigo R.A., Miller T.U. Adaptivnye antennye reshetki: Per. s angl. M.: Mir. 1986. S. 12–14. (in Russian)
- Litvinov O.S. O podavlenii pomekhovogo signala v polose chastot adaptivnoj antennoj reshetkoj. Radiotekhnika i elektronika. 1982. T. 27. Vyp. 11. S. 2264–2267. (in Russian)
- Litvinov O.S., Murod'yants D.V. Issledovanie spektral'nykh kharakteristik podavleniya pomekh v adaptivnykh antennykh reshetkakh na nejrosetevom upravlenii. Sb. tezisov dokladov XX Mezhdunar. nauch.-tekhnich. konf. «Nejroinformatika-2018». (in Russian)
- Khajkin S. Nejronnye seti: polnyj kurs. Izd. 2-e, ispr.: Per. s angl. M.: ID «Vil'yams». 2006. S. 219–340. (in Russian)
- Uossermen F. Nejrokomp'yuternaya tekhnika: Teoriya i praktika. M.: Mir. 1992. S. 26–54. (in Russian)