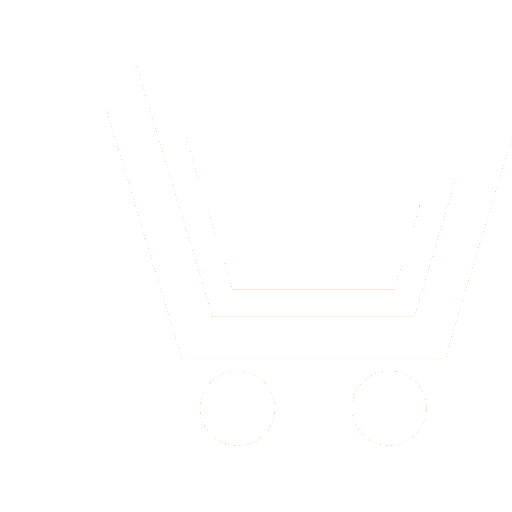
G.L. Safina – Ph.D.(Eng.), Associate Professor, Department «Applied Mathematics»,
National Research Moscow State University of Civil Engineering
E-mail: minkinag@mail.ru
A.G. Tashlinsky – Dr.Sc.(Eng.), Professor, Head of Department «Radio Engineering», Ulyanovsk State Technical University
E-mail: tag@ulstu.ru
M.G. Tsarev – Post-graduate Student, Ulyanovsk State Technical University E-mail: michael.tsaryov@gmail.com
An optimal distance between the samples, providing a maximum of information on the mismatch of the images at a given sample size, exists for estimation of two images mutual information from a local sample of their samples. The problem to determine this distance under conditions of additive noisy images is considered. Its solution is based on the fact that the maximum information about image mismatch occurs with the maximum of the ratio of the mathematical expectation module of the mutual information gradient to its root-mean-square deviation. Expressions for the optimal distance determination are obtained. It is shown that it depends on the correlation function of the image and the signal-to-noise ratio.
- Stanier S.A. Improved image-based deformation measurement for geotechnical applications Stanier // Canadian Geotechnical Journal. 2015. T. 53. № 5. P. 727−739.
- Shalev-Shwartz Shai and Zhang Tong. Accelerated proximal stochastic dual coordinate ascent for regularized loss minimization // Mathematical Programming. 2016. V. 155. № 1. P. 105−145.
- Rocco I., Arandjelovic R., Sivic J. Convolutional neural network architecture for geometric matching // Proc. CVPR. 2017. T. 2. P 6148−6157.
- Tashlinskij A.G., Voronov S.V. Ispol’zovanie vzaimnoj informaczii kak czelevoj funkczii kachestva oczenivaniya parametrov izobrazhenij // Avtomatizacziya proczessov upravleniya. 2014. № 3 (37). S. 32−39.
- Tashlinskiy A.G., Zhukova A.V. Effectiveness of correlation and information measures for synthesis of recurrent algorithms for estimating spatial deformations of video sequences // CEUR Workshop Proceedings. 2017. V. 1901. P. 235−239.
- Shannon C.E., Weaver W. The mathematical theory of communication // The Mathematical Theory of Communication. 1949. P 29−125.
- Maes F.,Vandermeulen D., Suetens P. Medical image registration using mutual information // Proc. IEEE. 2003. V. 91. № 10. P 1699−1722.
- Tashlinskii A.G., Safina G.L., Voronov S.V. Pseudogradient optimization of objective function in estimation of geometric interframe image deformations // Pattern recognition and image analysis. 2012. V. 22. № 2. P. 386−392.
- Xoreva A.M., Tashlinskij A.G., Smirnov P.V. Vy’bor konechny’x raznostej pri naxozhdenii psevdogradienta czelevoj funkczii v proczedurax oczenivaniya mezhkadrovy’x deformaczij izobrazhenij // Radiotexnka. 2012. № 9. S. 56−60.
- Parzen E. On Estimation of a Probability Density Function and Mode // Annals of Math. Statistics. 1962. V. 33. P. 1065−1076.
- Tashlinskij A.G., Voronov S.V., Voronov I.V. Analiz czelevy’x funkczij v zadache oczenivaniya vzaimny’x geometricheskix deformaczij izobrazhenij // Avtomatizacziya proczessov upravleniya. 2013. № 4(34). S. 26−29.
- Viola P., Wells W.M. Alignment by maximization of mutual information // International Journal of Computer Vision. 1997. V. 24. P 137−154.
- Tashlinskii A.G., Minkina G.L. Pseudogradient Optimization in the Estimation of Geometric Interrame Image Deformations // Pattern Recognition and Image Analysis. 2008. V. 18. № 4. P. 707−712.