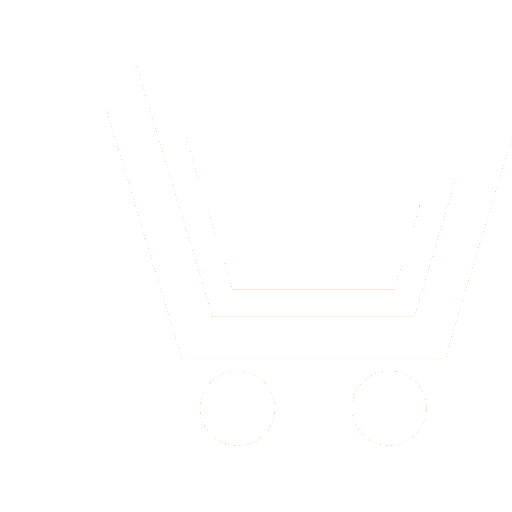
V.S. Kumov1, A.V. Samorodov2
1,2 Bauman Moscow State Technical University (Moscow, Russia)
The analysis of craniofacial morphology is extremely important in clinical genetics, since up to 40 % of all genetic diseases are accompanied by multiple changes in the structure of the face and head. The complexity and subjectivity of the description of the phenotype complicates the procedure of clinical differential diagnosis. Attempts are being made to automate the process of assessing phenotypic traits, but no accessible and interpretable method has been proposed for assessing congenital morphogenetic variants of the face that does not require 3D scanning. The purpose of this work is to develop and study a method for automated assessment of congenital morphogenetic variants of a face from a 2D image. A study was conducted to estimate the error of phenotypic parameters evaluation and the accuracy of face congenital morphogenetic variants recognition. A comparison was made of two approaches to the assessment of phenotypic parameters using direct 2D image and the result of 3D reconstruction. The method of automated assessment of congenital morphogenetic variants from a single 2D frontal face image, based on localization of facial points in a reconstructed 3D image, makes it possible to identify them with an average accuracy of 90 %. As a result of the research, the method was developed for the automated assessment of congenital morphogenetic variants from a single 2D frontal image of the face, based on localization of facial points in the reconstructed 3D image.
Kumov V.S., Samorodov A.V. Study of the method for automated assessment of congenital morphogenetic variants of the face in 2D images. Biomedicine Radioengineering. 2023. V. 26. № 4. P. 28–36. DOI: https://doi.org/10.18127/j15604136-202302-03 (In Russian)
- Batysheva T.T., Kondakova O.B., Kvasova O.V. Nasledstvennyye zabolevaniya v praktike detskogo nevrologa. Metodicheskiye rekomendatsii № 24. Moskva, 2015. 32 s. (in Russian).
- Ginzburg B.G. Metody opredeleniya chastoty vrozhdennykh porokov razvitiya i vrozhdennykh morfogeneticheskikh variantov u detey v sisteme geneticheskogo monitoringa. Pediatriya. Zhurn. im. G.N. Speranskogo. 1999. № 4. S. 41–44. (in Russian).
- Meleshkina A.V., Chebysheva S.N., Burdayev N.I. Malyye anomalii razvitiya u detey. Diagnostika i vozmozhnosti profilaktiki. Consilium medicum. 2015. V. 17. № 6. S. 68–72. (in Russian).
- Wright C.F. et al. Paediatric genomics: diagnosing rare disease in children. Nature Reviews Genetics. 2018. V. 19. № 5. P. 253–268.
- Robinson P.N. et al. The Human Phenotype Ontology: a tool for annotating and analyzing human hereditary disease. The American J. of Human Genetics. 2008. V. 83. № 5. P. 610–615.
- Weinreich S.S. et al. Orphanet: a European database for rare diseases. Nederlands tijdschrift voor geneeskunde. 2008. V. 152. № 9. P. 518–519.
- Hart T.C., Hart P.S. Genetic studies of craniofacial anomalies: clinical implications and applications. Orthodontics & craniofacial research. 2009. V. 12. № 3. P. 212–220.
- Hochheiser H. et al. The FaceBase Consortium: a comprehensive program to facilitate craniofacial research. Developmental Biology. 2011. V. 355. № 2. P. 175–182.
- Dalrymple K.A., Gomez J., Duchaine B. The Dartmouth Database of Children’s Faces: Acquisition and validation of a new face stimulus set. PloS one. 2013. V. 8. № 11. P. e79131.
- Ferry Q. et al. Diagnostically relevant facial gestalt information from ordinary photos. Elife. 2014. V. 3. № e02020. P. 1–22.
- Gender toolpie. URL: https://gender.toolpie.com/
- Deng Y. et al. Accurate 3D Face Reconstruction with Weakly-Supervised Learning: From Single Image to Image Set. IEEE Computer Vision and Pattern Recognition Workshop (CVPRW) on Analysis and Modeling of Faces and Gestures (AMFG). 2019.
- Deep3DFaceReconstruction. URL: https://github.com/microsoft/Deep3DFaceReconstruction
- Farkas L.G. (ed.). Anthropometry of the Head and Face. Lippincott Williams & Wilkins, 1994.
- Deutsch C.K. et al. The Farkas system of craniofacial anthropometry: methodology and normative databases. Handbook of Anthropometry. NY: Springer, 2012. P. 561–573.
- Palmer R.L., Helmholz P., Baynam G. Cliniface: phenotypic visualisation and analysis using non-rigid registration of 3D facial images. The International Archives of the Photogrammetry, Remote Sensing and Spatial Information Sciences. 2020. V. 43. P. 301–308.
- Kumov V.S., Samorodov A.V. Issledovaniye prostranstva priznakov dlya postroyeniya sistemy avtomatizirovannogo raspoznavaniya nasledstvennykh zabolevaniy po izobrazheniyu litsa. Biomeditsinskaya radioelektronika. 2022. T. 25. № 5. S. 49–57. (in Russian).
- Kumov V., Samorodov A. Recognition of genetic diseases based on combined feature extraction from 2D face images. 2020 26th Conference of Open Innovations Association (FRUCT). IEEE. 2020. P. 1–7.
- Cornejo J.Y.R., Pedrini H. Recognition of Genetic Disorders Based on Deep Features and Geometric Representation. Iberoamerican Congress on Pattern Recognition. Springer. Cham. 2018. P. 665–672.
- Gurovich Y. et al. Identifying facial phenotypes of genetic disorders using deep learning. Nature medicine. 2019. V. 25. № 1. P. 60–64.
- Muers L. et al. Clinical versus automated assessments of morphological variants in twins with and without neurodevelopmental disorders. American Journal of Medical Genetics Part A. 2020. V. 182A. P. 1177–1189.