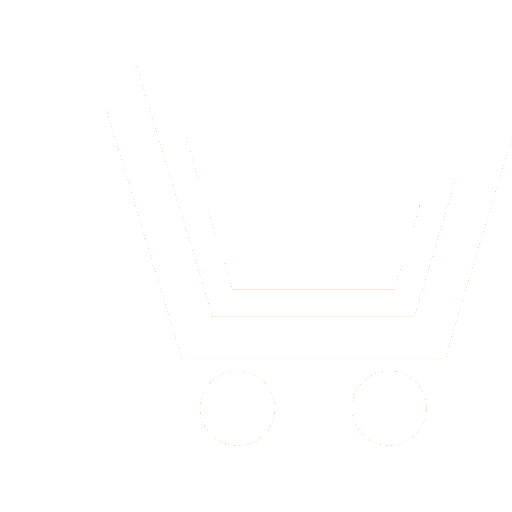
V.S. Kumov1, A.V. Samorodov2
1,2 Bauman Moscow State Technical University (Moscow, Russia)
Phenotypic features of the face and head are extremely important for geneticists, since a number of syndromes are characterized by certain distinctive features of the craniofacial morphology. The description of the patient's phenotype during the clinical examination is often subjective. Attempts are being made to automate the recognition of hereditary diseases from a face image, but the problem of constructing the best feature space for such system has not been solved. Thus, this work is devoted to study various feature spaces for automated recognition of hereditary diseases from a face image. The algorithm for recognition of hereditary diseases was trained and tested using different combinations of features: coordinates of control points, deep features, distances and indices and their z-scores. When using anthropometric features that characterize the structure of the face and their z-scores, it is possible to build a classifier that provides more than 90 % classification accuracy for 8 and 9 classes at rank r = 3. The selection of the features using a greedy algorithm, do not lead to an increase in classification accuracy, therefore, it is advisable to use the full set of 32 distances or their z-scores. Classification accuracy is higher when using distances and indices than when using their z-scores, which is due to errors in automatic age determination. A study of the dependence of the probabilities of correct, erroneous, and indeterminate recognitions on the decision threshold showed that at a zero error rate (at a threshold equal to 0.5) for rank r = 2, the probability of correct recognitions and refusal to make a decision are 80 % and 20 %, respectively, at taking into account the values of the indices, which indicates the possibility of reducing the error and the risk of incorrect recognition while maintaining high probability of correct recognition. The highest classification accuracy values were obtained using combined features, including both geometric and deep features.
Kumov V.S., Samorodov A.V. Investigation of the feature space for building a system for automated recognition of hereditary diseases from a face image. Biomedicine Radioengineering. 2022. V. 25. № 5. Р. 49-57. DOI: https://doi.org/10.18127/j15604136-202205-06 (In Russian)
- Pisarchik G.A., Malinovskaya Yu.V. Meditsinskaya genetika: Uchebno-metodicheskoye posobiye. Minsk: IVTs Minfina. 2017. 156 s.
(in Russian). - Babtseva A.F., Yutkina O.S., Romantsova E.B. Meditsinskaya genetika: Uchebnoye posobiye dlya studentov lechebnogo i pediatricheskogo fakultetov. Blagoveshchensk: GBOU VPO Amurskaya gosudarstvennaya meditsinskaya akademiya. 2012. 165 s. (in Russian).
- Bochkov N.P., Puzyrev V.P., Smirnikhina S.A. Klinicheskaya genetika. M.: GEOTAR-Media. 2002. (in Russian).
- Hart T.C., Hart P.S. Genetic studies of craniofacial anomalies: clinical implications and applications. Orthodontics & craniofacial research. 2009. V. 12. № 3. P. 212-220.
- Farkas L.G. (ed.). Anthropometry of the Head and Face. Lippincott Williams & Wilkins. 1994.
- Deutsch C.K. et al. The Farkas system of craniofacial anthropometry: methodology and normative databases. Handbook of Anthropometry. NY: Springer. 2012. P. 561–573.
- Hochheiser H. et al. The FaceBase Consortium: a comprehensive program to facilitate craniofacial research. Developmental Biology. 2011. V. 355. № 2. P. 175–182.
- Farkas L.G., Munro I.R. (ed.). Anthropometric facial proportions in medicine. Charles C Thomas Pub Limited. 1987.
- Farkas L.G. et al. Proportion indices in the craniofacial regions of 284 healthy North American white children between 1 and 5 years of age. Journal of Craniofacial Surgery. 2003. V. 14. № 1. P. 13–28.
- Robinson P.N. et al. The Human Phenotype Ontology: a tool for annotating and analyzing human hereditary disease. The American J. of Human Genetics. 2008. V. 83. № 5. P. 610–615.
- Ferry Q. et al. Diagnostically relevant facial gestalt information from ordinary photos. Elife. 2014. V. 3. № e02020. P. 1–22.
- Gurovich Y. et al. Identifying facial phenotypes of genetic disorders using deep learning. Nature medicine. 2019. V. 25. № 1. P. 60–64.
- Kumov V., Samorodov A. Recognition of genetic diseases based on combined feature extraction from 2D face images. 2020 26th Conference of Open Innovations Association (FRUCT). IEEE. 2020. С. 1–7.
- Kumov V.S., Samorodov A.V., Solonichenko V.G., Kanivets I.V., Gorgisheli K.V. Biotekhnicheskaya sistema dlya avtomatizirovannykh issledovaniy vrozhdennykh morfogeneticheskikh variantov litsa. Biotekhnosfera. 2021. № 1 (66). S. 3–9. (in Russian).
- Deng Y. et al. Accurate 3D Face Reconstruction with Weakly-Supervised Learning: From Single Image to Image Set. IEEE Computer Vision and Pattern Recognition Workshop (CVPRW) on Analysis and Modeling of Faces and Gestures (AMFG). 2019.
- Cornejo J.Y.R., Pedrini H. Recognition of Genetic Disorders Based on Deep Features and Geometric Representation. Iberoamerican Congress on Pattern Recognition. Springer. Cham. 2018. P. 665–672.
- Pooch E.H.P., Alva T.A.P., Becker C.D.L. A Computational Tool for Automated Detection of Genetic Syndrome Using Facial Images. Brazilian Conference on Intelligent Systems. Springer. Cham. 2020. P. 361–370.
- Parkhi O.M., Vedaldi A., Zisserman A. Deep face recognition. in Proceedings of the British Machine Vision. 2015. V. 1. № 3. P. 6.
- Dalrymple K.A., Gomez J., Duchaine B. The Dartmouth Database of Children’s Faces: Acquisition and validation of a new face stimulus set. PloS one. 2013. V. 8. № 11. P. e79131.
- Gender toolpie. URL: https://gender.toolpie.com/