350 rub
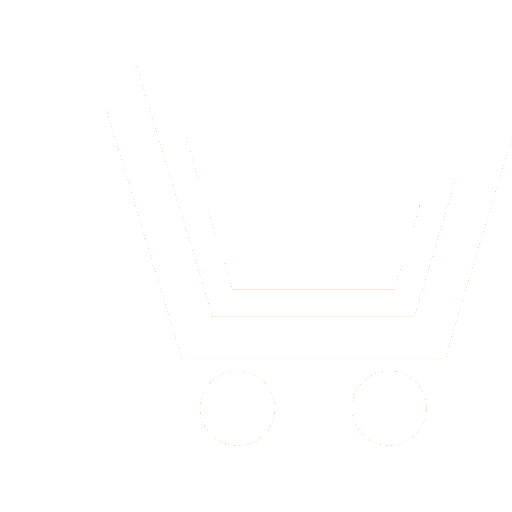
Journal Biomedical Radioelectronics №8 for 2016 г.
Article in number:
Estimation electroencephalogram entropy ro-bust algorithm
Authors:
O.V. Cvetkov Dr.Sc. (Eng.), Professor, Department of Biotechnical Systems, Saint Petersburg Electrotechnical University «LETI»
E-mail: ovts@bk.ru
Y.A. Zhivolupova Post-graduate Student, Department of Biotechnical Systems, Saint Petersburg Electrotechnical University «LETI»
E-mail: iulia.zhivolupova@gmail.com
V.V. Savchenko Senior Research Scientist, Department of Biotechnical Systems, Saint Petersburg Electrotechnical University «LETI»
E-mail: savchenko@mail.ru
Abstract:
Nonlinear analysis of the electroencephalogram signal (EEG) comprises entropy, fractal and other analysis methods. They are based on the assumption that the EEG is a process that reflect activities of complex nonlinear dynamic self-organizing system. Evaluation of system characteristics in different experimental situations may provide useful information about brain functions and shed light on the mechanisms of human and higher animals information processing.
In practice, results of these studies are inapplicable due to the high variability of estimates and their dependence on a number of factors which difficult to control in the experiment.
Since we assume that, in general, experiments are conducted using different equipment, it is necessary to standardize a number of characteristics in the process of EEG pre-processing because they vary in a wide range depending on the manufacturer.
To overcome the problem of comparability of research results obtained in different laboratories, it is necessary:
1) standardize the conditions of EEG registration and analysis (for entropy estimation),
2) ensure independence of entropy estimation on the factors that can not be standardized.
Proposed operations with the original EEG signal have helped to bring a number of EEG-signal characteristics to the common standards.
As a result, we obtain the following algorithm for robust processing of EEG signal and estimation of its entropy:
1. Lead input signal to the standard conditions (bandwidth of 0.5 - 35 Hz and a sampling rate of 500 Hz)).
2. Convert the original sequence of samples in a locally-rank code.
3. Calculate Sample entropy obtained by locally-rank code.
4. Multiply SE on the correcting factor (0,86) and sampling frequency (500) to obtain an unbiased estimate, measured in bit / s.
The algorithm is based on application of a Sample entropy with prior locally-rank coding and allows to obtain robust estimation of EEG signals and constitutes a new tool for researchers.
Entropy graphs analysis requires some experience, and in the case of development of the basic principles lets you quickly assess the quality of the recorded EEG, define the intervals of its stationary, select transitions between states and other interested researchers moments, to assess the individual characteristics of the subject.
Pages: 20-25
References
- Cvetkov O.V. EHntropijjnyjj analiz dannykh v fizike, biologii i tekhnike. SPb.: Izd-vo SPbGEHTU «LEHTI». 2015. 202 s.
- Shalbaf R., Behnam H., Sleigh J., Voss L. Measuring the effects of sevoflurane on electroencephalogram using sample entropy // Acta Anaesthesiol Scand. 2012. V. 56. P. 880-889.
- Abásolo D., Hornero R., Espino P. Entropy analysis of the EEG background activity in Alzheimer-s disease patients // Physiological Measurement. 2006. V. 27(3). P. 241-253.
- Yum M.K. et al. Effect of a ketogenic diet on EEG: Analysis of sample entropy // Seizure. 2008. V. 17. P. 561-566.
- Huang J.-R. et al. Application of multivariate empirical mode de-composition and sample entropy in EEG signals via artificial neural networks for interpreting depth of anesthesia // Entropy. 2013. V. 15. № 9. P. 3325-3339.
- Bruce E.N., Bruce M.C., Vennelaganti S. Sample entropy tracks changes in EEG power spectrum with sleep state and aging // Journal of Clinical Neurophysiology. 2009. V. 26. № 4. P. 257-266.
- Mathew B.A. Entropy of electroencephalogram (EEG) signals changes with sleep state / master\'s theses. University of Kentucky. 2003. P. 203.
- Cvetkov O.V. Vychislenie ocenki ehntropii biosignala, invariantnojj k izmeneniju ego amplitudy, s ispolzovaniem rangovogo jadra // Izvestija vuzov. Ser. Radioehlektronika. 1991. № 8. S. 108-110.
- Tietze H., Wurzburg I. Stages of fatigue during long-duratin driving reflected in alpha-related events in the EEG // International Conference on Traffic and Transport Psychology. Bern. 2000.
- Bein B. Entropy // Best Practice & Research Clinical Anaesthesiology. 2006. V. 20. № 1. P. 101-109.