350 rub
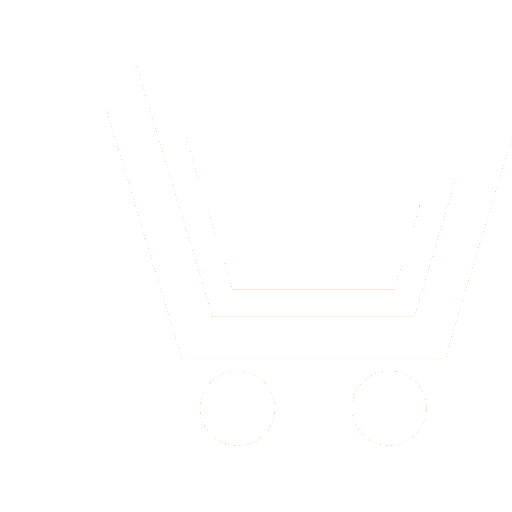
Journal Biomedical Radioelectronics №3 for 2012 г.
Article in number:
An atrial arrhythmia classification using wavelet transform of the ECG and machine learning approach
Keywords:
support vector machine
atrial arrhythmias
stationary wavelet transform
heart rate variability
Authors:
M.V. Voitikova, A.P. Voitovich
Abstract:
Classification of atrial arrhythmias problem in cardiology can be solved by the machine learning approach. In case of atrial arrhythmia classification (atrial fibrillation - AF and atrial flutter - AFL) we offer the support vector machine (SVM) based classifier. The classification problem consists of: i) the extraction of the ECG fragments which correspond to electric activity of the atrium and definition of statistical signs of arrhythmia type, ii) the dimensionality reduction of the feature parameters space. iii) the training of the binary classifier by training set of ECG samples with known diagnosis and iv) the classification of the test samples of ECG.
Initially 10 different features are extracted from the input ECG and HRV signals and a subset of these features is selected to train the SVM-based classifier. Components of the vector represent the energy distribution of the wavelet coefficients of the ECG (ECG fragments - intervals concluded between 2 consecutive R-waves) and the statistical parameters of heart rate variability (the average RR-interval and standard deviation).
SVM constructs the set of hyperplanes in multi-dimensional features space with the best separation and largest distance to the nearest training data points of two classes - AF and AFL. We considered the RBF kernel as a kernel function for SVM and selected the best kernel parameters empirically. SVM classifier training for AF and AFL arrhythmias is considered finished at for maximum value of quality indicator of signals classification (in our case this value is equal 0.98).
Pages: 12-18
References
- Рябыкина Г.В., Соболев А.В. Вариабельность ритма сердца. М. 2001.
- Yaghouby F., Ayatollahi A., Bahramali R. et. al. Towards automatic detection of atrial fibrillation: A hybrid computational approach // Computers in Biology and Medicine. 2010. V. 40. P.919 - 930.
- Войтикова М.В., Войтович А.П. Определение формы фибрилляции предсердий человека на основе вейвлет-анализа электрокардиограммы // Известия HAH Беларуси. 2006. № 1. С. 94 - 99.
- Войтикова М.В., Войтович А.П. Применение вейвлет-анализа вариабельности ритма сердца человека для диагностики желудочковой тахикардии и фибрилляции // Биомедицинская радиоэлектроника. 2008. № 12. C. 21 - 26.
- Yaghouby F., Ayatollahi A. An arrhythmia classification method based on selected features of heart rate variability signal and support vector machine-based classifier // IFMBE Proceedings 25/IV. 2009. P. 1928-1931.
- Tsipouras M.G., Goletsis Y., Fotiadis D.I. A method for arr-hythmic episode classification in ECGs using fuzzy logic and Markov models // Proceedings of the computers in cardiology. 2004. P. 361 - 364.
- Vapnik V. Statistical learning theory. Berlin: Springer. 1998.
- MIT-BIH ECG database. Physionet Bank. Massachusetts Institute of Technology.
- Heart rate variability. Standards of measurement, physiological interpretation and clinical use. Task force of the European society of cardiology and the North American society of pacing and electrophysiology // Eur. Heart J. 1996. V. 17. P. 354 - 381.
- Добеши И. Десять лекций по вейвлетам. Москва, Ижевск: НИЦ «Регулярная и хаотическая динамика». 2001.
- Rosso O.A., Martin M.T., Figliola A. EEG analysis using wavelet-based information tools // Journal of Neuroscience Methods. 2006. V. 153. P. 163-182.