350 rub
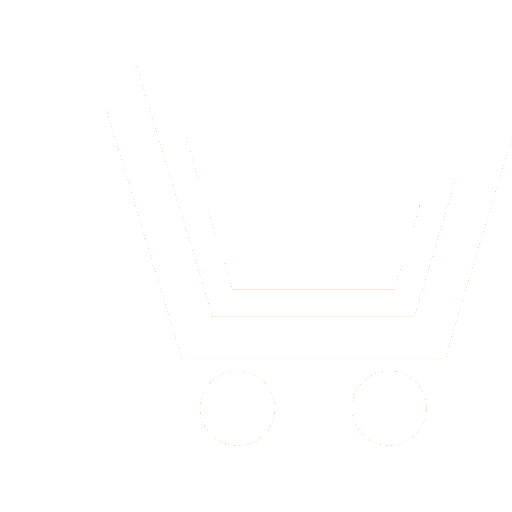
Journal Biomedical Radioelectronics №1 for 2012 г.
Article in number:
Return Interval Statistics of Extremes in Multiplicative Cascade Model: An Analytical Approach
Authors:
O.A. Markelov, M.I. Bogachev, A.A. Sokolova, A.R. Kayumov
Abstract:
In the last decade, multiplicative cascades have been introduced as an efficient tool to simulate data with nonlinear long-range dependence that can be observed very broadly in nature, including many processes and structures in biology and physiology. In has been shown that this long-range dependence affects significantly return interval statistics leading to broader probability density functions and pronounced conditional memory. Recently several tools aiming at predicting extremes in physiological records that may lead to undesirable physiological conditions and implementation of biofeedback algorithms to avoid them that utilize the return interval approach have been suggested. Finite size effect appears as a limitation for the available solutions based on finite data simulations. The paper suggests an analytical functional form that is capable of describing the probability density function of return intervals in a deterministic multiplicative cascade model for any given threshold level and data length. It is also shown that this functional form remains valid in the randomized multiplicative cascade that is the most common general model representing nonlinear long-range memory in biological and physiological data. The solution aims at overcoming finite size limitations in applications related to prediction and biofeedback utilizing the return interval approach.
Pages: 45-48
References
- Peng C.-K., Buldyrev S. V., Havlin S. et al. Mosaic organization of DNA nucleotides // Phys. Rev. E. 1994. V. 49. P. 1685-1689.
- Yu Z.-G., Ahn V., Lau K.-S. Multifractal and correlation analyses of protein sequences from complete genomes // Phys. Rev. E. 2003. V. 68. P. 021913 (1-10).
- Ivanov P. Ch., Rosenblum M.G., Peng C.-K. et al. Scaling behaviour of heartbeat intervals obtained by wavelet-based time-series analysis // Nature (London). 1996. V. 383. P. 323 - 327.
- Ivanov P. Ch., Amaral L.A.N., Goldberger A.L. et al. Multifractality in human heartbeat dynamics // Nature (London). 1999. V. 399. P. 461 - 465.
- Huikuri H.V., Mäkikallio T.H., Perkiömäki J.Measurement of heart rate variability by methods based on nonlinear dynamics // J. Electrocardiol. 2003. V. 36. P. 95 - 99.
- Bogachev M.I., Kireenkov I.S., Nifontov E.M., Bunde A. Statistics of return intervals between large heartbeat intervals and their usability for prediction of disorders. // New Journal of Physics. 2009. V. 11. P. 063036 (1 - 18).
- Sokolova A., Bogachev M.I., Bunde A. Clustering of ventricular arrhythmic complexes in heart rhythm // Phys. Rev. E. 2011. V. 83. P. 021918(1 - 7).
- Богачев М.И. Оценка значимости кратковременной и долговременной зависимости при прогнозировании кратковременной динамики физиологических ритмов. // Биомедицинская радиоэлектроника. 2010. № 12. C. 3 - 11.
- Mandelbrot B.B. Gaussian Self-Affinity and Fractals. New York: Springer. 2001.