350 руб
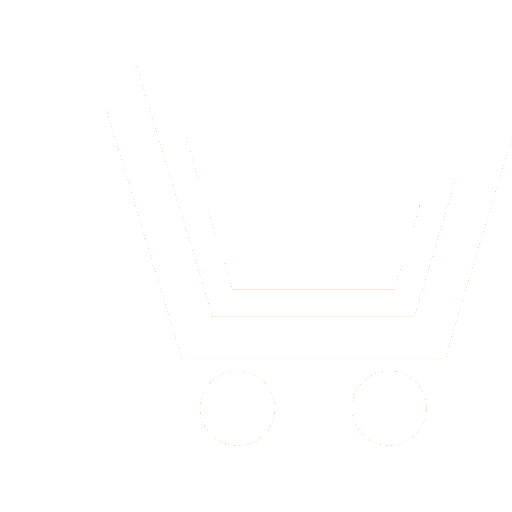
Журнал «Радиотехника» №6 за 2018 г.
Статья в номере:
Подход к трансформации кластерного дерева признаков в векторное пространство признаков
Тип статьи:
научная статья
УДК: 004.4, 681.3
Авторы:
П.В. Дударин – аспирант, Ульяновский государственный технический университет
E-mail: p.dudarin@ulstu.ru
Н.Г. Ярушкина – д.т.н., профессор, зав. кафедрой «Информационные системы», Ульяновский государственный технический университет E-mail: jng@ulstu.ru
Аннотация:
Представлен подход к трансформации дерева признаков в векторное пространство признаков для задачи кластеризации. Показано, что основными преимуществами предложенного подхода являются: сохранение информации из исходной иерархической структуры и снижение размерности пространства признаков.
Страницы: 63-72
Список источников
- Jain A.K., Murty M.N., Flynn P.J. Data Clustering: A Review // ACM Computing Surveys (CSUR) (USA). 1999. V. 31. № 3. P. 264−323.
- Amorim Renato. Feature Weighting for Clustering: Using K-Means and the Minkowski. LAP Lambert Academic Publishing. 2012.
- Modha D.S., Spangler W.S. Feature Weighting in K-Means Clustering // Machine Learning. 2003. 52: 217. doi.org/10.1023/A:1024016609528.
- Zhang T., Ramakrishnan R.; Livny M. BIRCH: an efficient data clustering method for very large databases // Proceedings of the ACM SIGMOD international conference on Management of data (SIGMOD). 1996. P. 103−114. doi:10.1145/233269.233324.
- Li J., Wang K., Xu L. Chameleon based on clustering feature tree and its application in customer segmentation // Ann Oper Res. 2009. P. 168−225. doi.org/10.1007/s10479-008-0368-4.
- Mansoori E.G. GACH: a grid based algorithm for hierarchical clustering of high-dimensional data // Soft Computing. 2004. V. 18. № 5. P. 905−922.
- Федеральный закон «О стратегическом планировании в Российской Федерации» № 172-ФЗ от 28.07.2014 г. URL = http://pravo.gov.ru/proxy/ips/?docbody=&nd=102354386 (02.05.2018).
- Dudarin P., Pinkov A., Yarushkina N. Methodology and the algorithm for clustering economic analytics object, Automation of Control Processes. 2017. V. 47. № 1. P. 85−93.
- Ester M., Kriegel H.P., Sander J., Xu X. A Density-Based Algorithm for Discovering Clusters in Large Spatial Databases with Noise // Proceedings of the 2ndInternational Conference on Knowledge Discovery and Data Mining. Portland, OR. AAAI Press. 1996. P. 226−231.
- Vincent D. Blondel, Jean-Loup Guillaume, Renaud Lambiotte, Etienne Lefebvre. Fast unfolding of communities in large networks // J. Stat. Mech. 2008.
- Zhang J., Wang Y., Feng J. A hybrid clustering algorithm based on PSO with dynamic crossover // Soft Computing. 2014. V. 18. № 5. P. 961−979.
- Q. Le, T. Mikolov. Distributed Representations of Sentences and Documents // Proceedings of the 31st International Conference on Machine Learning (PMLR). 2014. 32(2). 1188−1196.
- Mikolov T., Sutskever I., Chen K., Corrado G., Dean J. Distributed representations of words and phrases and their compositionality // Proceedings of the 26thInternational Conference on Neural Information Processing Systems. Lake Tahoe (Nevada). 5−10 December 2013. P. 3111−3119.
- Dudarin P.V., Yarushkina N.G. An Approach to Fuzzy Hierarchical Clustering of Short Text Fragments Based on Fuzzy Graph Clustering // Proceedings of the Second International Scientific Conference «Intelligent Information Technologies for Industry» (IITI). 2017. Advances in Intelligent Systems and Computing. 2018. V. 679. Springer. Cham.
- Дударин П.В., Ярушкина Н.Г. Формирование признаков из иерархического классификатора для кластеризации коротких текстовых фрагментов // Нечеткие системы и мягкие вычисления. 2017. Т. 12. № 2. С. 87−96.
- Дударин П.В., Ярушкина Н.Г. Алгоритм построения иерархического классификатора коротких текстовых фрагментов на основе кластеризации нечеткого графа // Радиотехника. 2017. № 6.
- Rosenfeld A. Fuzzy graphs // Zadeh L.A., Fu K.S., Tanaka K., Shimura M. (Eds.) Fuzzy Sets and Their Applications to Cognitive and Decision Processes. Academic Press. New York. 1975. P. 77−95.
- Ruspini E.H. A new approach to clustering // Inform. and Control. 1969. 15 (1). 22−32.
- Raymond T Yeh, Bang S.Y. Fuzzy relation, fuzzy graphs and their applications to clustering analysis // Fuzzy Sets and their Applications to Cognitive and Decision Pro-cesses. Academic Press. P. 1975. P. 125−149. ISBN 9780127752600.
- Jolliffe I.T. Principal Component Analysis. Springer-Verlag. 1986. P. 487. doi:10.1007/b98835, ISBN 978-0-387-95442-4.
- Ball, Geoffrey H., Hall, David J. Isodata: a method of data analysis and pattern classification. Stanford Research Institute, Menlo Park (United States). Office of Naval Re-search. Information Sciences Branch. 1965.
- Brendan J. Frey and Delbert Dueck, Clustering by Passing Messages Between Data Points. Science. Feb. 2007.
- Comaniciu D., Meer P. Mean shift: A robust approach toward feature space analysis // IEEE Transactions on Pattern Analysis and Machine Intelligence. 2002.
- Rokach L., Maimon O. Clustering Methods // Maimon O., Rokach L. (eds). Data Mining and Knowledge Discovery Handbook. Springer. Boston. MA. 2005.
- Pedregosa F. et al. Scikit-learn: Machine Learning in Python // Journal of Machine Learning Research. 2011. V. 12. P. 2825−2830.
- Hubert L., Arabie P. Comparing partitions // Journal of Classification. 1985. V. 2. № 1. P. 193−218. doi:10.1007/BF01908075.
- Rousseeuw P.J. Silhouettes: a Graphical Aid to the Interpretation and Validation of Cluster Analysis // Computational and Applied Mathematics. 1987. P. 20: 53−65. doi:10.1016/0377-0427(87)90125-7.
Дата поступления: 24 мая 2018 г.