350 руб
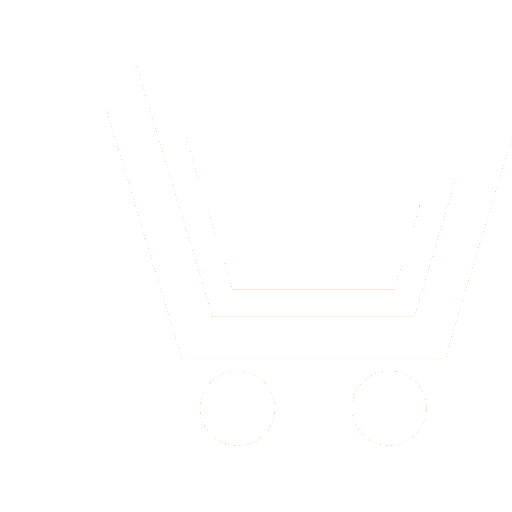
Журнал «Нейрокомпьютеры: разработка, применение» №4 за 2016 г.
Статья в номере:
Влияние «прореживания» пакетов на качество классификации потоков сетевого трафика методами машинного обучения
Ключевые слова:
сетевой трафик
классификация
технологии машинного обучения
алгоритмы классификации C4.5
SVM
AdaBoost
NaiveBayes
BayesNet
прореживание
Авторы:
О.И. Шелухин - д.т.н., профессор, зав. кафедрой «Информационная безопасность и автоматизация», Московский технический университет связи и информатики. E-mail: sheluhin@mail.ru
Ю.А. Калугин - аспирант, кафедра «Информационная безопасность и автоматизация», Московский технический университет связи и информатики. E-mail: derron210@gmail.com
Аннотация:
Исследовановлияние прореживания пакетов на точность классификации трафика методами машинного обучения на основе использования пакетных трассировок. Приведена оценка эффективности алгоритмов классификации C4.5, SVM, AdaBoost, NaiveBayes, BayesNet; в качестве приложений, используемых для классификации, рассматривались: web, p2p, ftp, mail.
Страницы: 14-24
Список источников
- Internet Assigned Numbers Authority (IANA), http://www.iana.org/assignments/port-numbers, as of August 12, 2008.
- Karagiannis T., Broido A., Faloutsos M. Transport layer identification of P2P traffic. In Proc. of ACM SIGCOMM IMC, August. 2004.
- Moore A., Papagiannaki K.Toward the accurate identification of network Applications. In Proc. of PAM Conf., March. 2005.
- Moore A., Zuev D. Internet trafficc classification using bayesian analysis techniques. In ACM SIGMETRICS Performance Evaluation Review.ACM. 2005. V.33.P.50-60. 2005.
- Sen S., Spatscheck O., Wang D. Accurate, scalable in-network identification of p2p traffic using application signatures,» in Proc. of WWW Conf.. May. 2004.
- OpenDPI, the Open Source version of ipoque\'s DPI software, http://www.opendpi.org/.
- nDPI, Open and Extensible GPLv3 Deep Packet Inspection Library, http://www.ntop.org/products/ndpi/.
- Carela-Espanol V., Barlet-Ros P., Cabellos-Aparicio A., Sole-Pareta J. Analysis of the impact of sampling on NetFlow traffic classification // Comput. Netw. 2011. V. 55.№ 5.P. 1083-1099.
- Cohen J.А coefficient of agreement for nominal scales. Educ. and Psychol.Meas. 1960. V. 20.№ 1.P. 37-46.
- Karagiannis T., Papagiannaki K., Faloutsos M. BLINC: Multilevel Traffic Classification in the Dark. In Proc. ACM SIGCOMM, Philadelphia, Pennsylvania, USA, August 2005.
- Information on See5/C5.0 - RuleQuest Research Data Mining Tools. 2011. [Online]. Available: http://www.rulequest.com/see5-info.html.
- Lim T.-S., Loh W.-Y., Shih Y. A comparison of prediction accuracy, complexity, and training time of thirty-three old and new classification algorithms // Machine Learn. 2000. V.40,Is. 3.P. 203-229.
- Moore A. W., Zuev D. Internet traffic classification using Bayesian analysis techniques // ACM SIGMETRICS 2005. Banff. Alberta (Canada). June2005. N.Y.: ACM, 2005. P. 50-60.
- Platt J. Fast Training of Support Vector Machines using Sequential Minimal Optimization / In B.Schoelkopf,C. Burges, A. Smola, editors, Advances in Kernel Methods - Support Vector Learning. 1998.
- Freund Y., SchapireR.E. Decision-theoretic generalization of on-line learning and an application to boosting // Journal of Computer and System Sciences. № 55. 1997.
- Weka: http://www.cs.waikato.ac.nz/ml/weka/.