350 руб
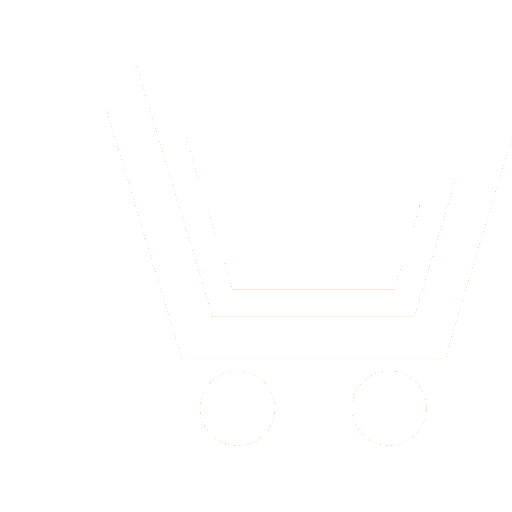
Журнал «Динамика сложных систем - XXI век» №4 за 2012 г.
Статья в номере:
Модели искусственных нейронных сетей в кредитном скоринге
Авторы:
А.М. Порошина - преподаватель, кафедра «Прикладная математика и моделирование в социальных системах», мл. научн. сотрудник, лаборатория инвестиционного анализа, Национальный исследовательский университет - Высшая школа экономики. E-mail: AMPoroshina@gmail.com
Аннотация:
Представлен анализ применения искусственных нейронных сетей для построения эффективных систем кредитного скоринга на рынке потребительского кредитования. Проведенный обзор эмпирических работ сопровождается рассмотрением ключевых преимуществ и недостатков использования искусственных нейронных сетей, а также выделением возможных направлений исследований в области моделирования кредитного риска.
Страницы: 83-88
Список источников
- Порошина А.М. Нейросетевой подход в оценке банковских кредитных рисков // Нейрокомпьютеры: разработка, применение. 2012. № 5. С. 54-60.
- Ясницкий Л.Н. Искусственный интеллект. М.: БИНОМ. Лаборатория знаний. 2011.
- Abdou H., Pointon J., and El-Masry A. Neural nets versus conventional techniques in credit scoring in Egyptian banking // Expert Systems with Applications. 2008. V. 35. P. 1275-1292.
- Akkoç S. An empirical comparison of conventional techniques, neural networks and the three stage hybrid Adaptive Neuro Fuzzy Inference System (ANFIS) model for credit scoring analysis: The case of Turkish credit card data // European Journal of Operational Research. 2012. V. 222. P. 168-178.
- Chen M.C., Huang S.H. Credit scoring and rejected instances reassigning through evolutionary computation techniques // Expert Systems with Applications. 2003. V. 24. P. 433-441.
- Chuang C-L., Huang S-T. A hybrid neural network approach for credit scoring // Expert Systems. 2011. V. 28. № 2. P. 185-196.
- Finlay S. Multiple classifier architectures and their application to credit risk assessment // European Journal of Operational Research. 2011. V. 210. P. 368-378.
- Khashman A. A Neural Network Model For Credit Risk Evaluation // International Journal of Neural System. 2009. V. 19. № 4. P. 285-294.
- Khashman A. Neural network for credit risk evaluation: Investigation of different neural network models and learning schemes // Expert Systems with Applications. 2010. V. 37. P. 6233-6239.
- Kumar P.R., Ravi V. Bankruptcy prediction in banks and firms via statistical and intelligent techniques. A review // European Journal of Operational Research. 2007. V. 180. P. 1-28.
- Lee T.-S., Chiu C.-C., Lu C.-J., and Chen, I.-F. Credit scoring using the hybrid neural discriminant technique // Expert Systems with Applications. 2002. V. 23. P. 245-254.
- Lim M.K. and Sohn S.Y. Cluster-based dynamic scoring model // Expert Systems with Applications. 2007. V. 32. P. 427-431.
- Ong C. S., Huang J. J. and Tzeng G. H. Building credit scoring models using genetic programming // Expert Systems with Applications. 2005. V. 29. P. 41-47.
- Šušteršiča M., Mramorb D., Zupanc J. Consumer credit scoring models with limited data // Expert Systems with Applications. 2009. V. 36. № 3. P. 4736-4744.
- Tsai C.F., Wu J.W. Using neural network ensembles for bankruptcy prediction and credit scoring // Expert Systems with Applications. 2008. V. 34. P. 2639-2649.
- Yu L., Wang
S.Y. and Lai K.K. Credit
risk assessment with a multistage neural network ensemble learning approach //
Expert Systems with Applications. 2008. V. 34.
P. 1434-1444. - West D. Neural network credit scoring models // Computers & Operations Research. 2000. V. 27. P. 1131-1152.
- Wang C.M., Huang Y.F. Evolutionary-based feature selection approaches with new criteria for data mining: A case study of credit approval data // Expert Systems with Applications. 2009. V. 36. № 3. P. 5900-5908.
- Xu X., Zhou C., and Wang, Z.
Credit scoring algorithm based on link analysis ranking with support vector
machine // Expert Systems with Applications. 2009. V. 36. № 2.
P. 2625-2632.