350 rub
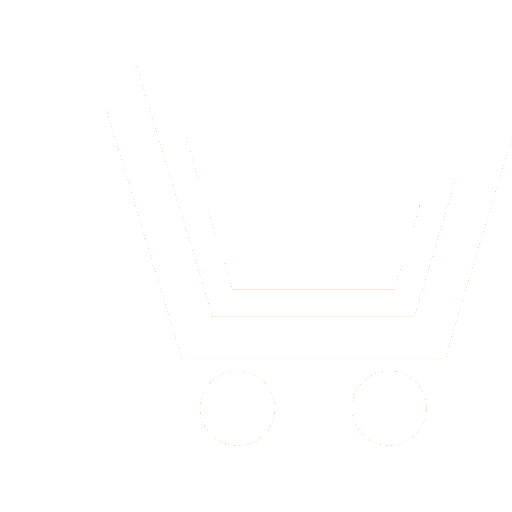
Journal Dynamics of Complex Systems - XXI century №4 for 2012 г.
Article in number:
Credit scoring based on artificial neural networks
Authors:
A.M. Poroshina
Abstract:
This paper analysis the application of artificial neural networks to tackle the problem of credit risk evaluation. They help to classify borrowers according its credit risk and to develop effective credit scorings systems on consumer credit market. We provide analysis related works, which based on using neural networks to credit risk modeling. The article emphasizes key advantages and disadvantages of artificial neural networks and to present ideas for future studies.
Pages: 83-88
References
- Порошина А.М. Нейросетевой подход в оценке банковских кредитных рисков // Нейрокомпьютеры: разработка, применение. 2012. № 5. С. 54-60.
- Ясницкий Л.Н. Искусственный интеллект. М.: БИНОМ. Лаборатория знаний. 2011.
- Abdou H., Pointon J., and El-Masry A. Neural nets versus conventional techniques in credit scoring in Egyptian banking // Expert Systems with Applications. 2008. V. 35. P. 1275-1292.
- Akkoç S. An empirical comparison of conventional techniques, neural networks and the three stage hybrid Adaptive Neuro Fuzzy Inference System (ANFIS) model for credit scoring analysis: The case of Turkish credit card data // European Journal of Operational Research. 2012. V. 222. P. 168-178.
- Chen M.C., Huang S.H. Credit scoring and rejected instances reassigning through evolutionary computation techniques // Expert Systems with Applications. 2003. V. 24. P. 433-441.
- Chuang C-L., Huang S-T. A hybrid neural network approach for credit scoring // Expert Systems. 2011. V. 28. № 2. P. 185-196.
- Finlay S. Multiple classifier architectures and their application to credit risk assessment // European Journal of Operational Research. 2011. V. 210. P. 368-378.
- Khashman A. A Neural Network Model For Credit Risk Evaluation // International Journal of Neural System. 2009. V. 19. № 4. P. 285-294.
- Khashman A. Neural network for credit risk evaluation: Investigation of different neural network models and learning schemes // Expert Systems with Applications. 2010. V. 37. P. 6233-6239.
- Kumar P.R., Ravi V. Bankruptcy prediction in banks and firms via statistical and intelligent techniques. A review // European Journal of Operational Research. 2007. V. 180. P. 1-28.
- Lee T.-S., Chiu C.-C., Lu C.-J., and Chen, I.-F. Credit scoring using the hybrid neural discriminant technique // Expert Systems with Applications. 2002. V. 23. P. 245-254.
- Lim M.K. and Sohn S.Y. Cluster-based dynamic scoring model // Expert Systems with Applications. 2007. V. 32. P. 427-431.
- Ong C. S., Huang J. J. and Tzeng G. H. Building credit scoring models using genetic programming // Expert Systems with Applications. 2005. V. 29. P. 41-47.
- Šušteršiča M., Mramorb D., Zupanc J. Consumer credit scoring models with limited data // Expert Systems with Applications. 2009. V. 36. № 3. P. 4736-4744.
- Tsai C.F., Wu J.W. Using neural network ensembles for bankruptcy prediction and credit scoring // Expert Systems with Applications. 2008. V. 34. P. 2639-2649.
- Yu L., Wang
S.Y. and Lai K.K. Credit
risk assessment with a multistage neural network ensemble learning approach //
Expert Systems with Applications. 2008. V. 34.
P. 1434-1444. - West D. Neural network credit scoring models // Computers & Operations Research. 2000. V. 27. P. 1131-1152.
- Wang C.M., Huang Y.F. Evolutionary-based feature selection approaches with new criteria for data mining: A case study of credit approval data // Expert Systems with Applications. 2009. V. 36. № 3. P. 5900-5908.
- Xu X., Zhou C., and Wang, Z.
Credit scoring algorithm based on link analysis ranking with support vector
machine // Expert Systems with Applications. 2009. V. 36. № 2.
P. 2625-2632.