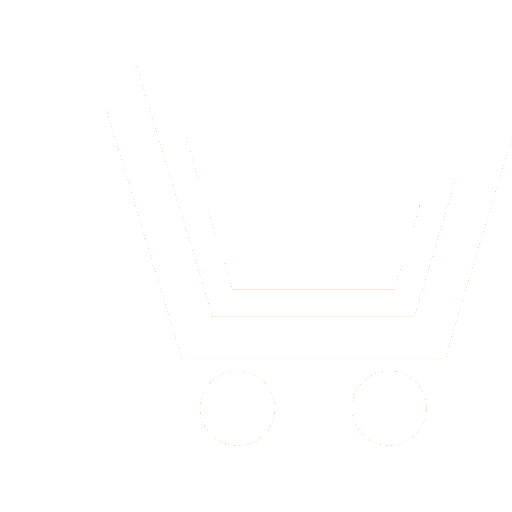
А.А. Нигрей – аспирант, Омский государственный университет путей сообщения (ОмГУПС)
E-mail: aa.nig@yandex.ru
С.С. Жумажанова – аспирант, радиотехнический факультет, Омский государственный технический университет (ОмГТУ)
E-mail: samal_shumashanova@mail.ru
А.Е. Сулавко – к.т.н., доцент, кафедра комплексной защиты информации; ст. науч. сотрудник, Омский государственный технический университет (ОмГТУ)
E-mail: sulavich@mail.ru
Постановка проблемы. Психофизиологическое состояние (ПФС) человека напрямую влияет на его возможность вести трудовую деятельность. В работе рассмотрены следующие ПФС: стресс, сон (все фазы и стадии), сонливость (засыпание), алкогольное опьянение. Данные состояния являются наиболее важными с точки зрения необходимости их своевременной идентификации в процессе профессиональной деятельности сотрудников, чья работа связана с высокой концентрацией внимания и повышенной опасностью.
Цель работы – аналитико-синтетическое исследование проблемы автоматической оценки (распознавания) психофизиологического состояния человека по параметрам электроэнцефалограмм (ЭЭГ).
Результаты. Проанализированы, описаны и обобщены существующие методы и подходы к определению данных состояний по параметрам ЭЭГ. Приведены достигнутые результаты по идентификации данных состояний с использованием методов машинного обучения и распознавания образов.
Практическое значение. Большинство рассмотренных исследований опираются на анализ ритмов ЭЭГ, которые коррелируют с ПФС. Можно выделить наиболее перспективные методы распознавания состояний с использованием нейронных сетей и каскадов классификаторов. Несмотря на высокий уровень точности рассмотренных подходов, апробированные в лабораторных условиях методы имеют ряд проблем, которые на сегодняшний день являются нерешенными и требуют дальнейших исследований.
- Леонова А.Б., Кузнецова А.С. Функциональные состояния и работоспособность человека в профессиональной деятельности // Психология труда, инженерная психология эргономика. Гл. 13 / Под ред. Е.А. Климова и др., М.: Юрайт. 2015.
- Богомолов А.В., Гридин Л.А., Кукушкин Ю.А., Ушаков И.Б. Диагностика состояния человека: математические подходы. М.: Медицина. 2003. 464 с.
- Michael J. Aminoff, Aminoff's Electrodiagnosis in Clinical Neurology (Sixth Edition). 2012.
- Lukas S.E. et al. Topographic distribution of EEG alpha activity during ethanol-induced intoxication in women // J. Stud. Alcohol. 1989. V. 50. № 2. P. 176–185.
- Yang Q. et al. Cortical synchrony change under mental stress due to time pressure // 2010 3rd International Conference on Biomedical Engineering and Informatics. 2010. V. 5. P. 2004–2007.
- Jensen O., Goel P., Kopell N., Pohja M., Hari R., Ermentroutf B. On the human sensorimotor-cortex beta rhythm: Sources and modeling // NeuroImage. 2005. V. 26. P. 347–355.
- Choi Y., Kim M., Chun C. Measurement of occupants’ stress based on electroencephalograms (EEG) in twelve combined environments // Build. Environ. 2015. V. 88. P. 65–72.
- Кирой В.Н., Ермаков П.Н. Электроэнцефалограмма и функциональные состояния человека. Ростов-на-Дону: Изд-во Ростовского ун-та. 1998.
- Harmony T. et al. EEG delta activity: an indicator of attention to internal processing during performance of mental tasks // Int. J. Psychophysiol. 1996. V. 24. № 1. P. 161–171.
- Gärtner M., Grimm S., Bajbouj M. Frontal midline theta oscillations during mental arithmetic: effects of stress // Front. Behav. Neurosci. 2015. V. 9.
- Pineda J.A. The functional significance of mu rhythms: Translating “seeing” and “hearing” into “doing” // Brain Research Reviews. 2005. V. 50. № 1. P. 57–68.
- Sege C.T., Bradley M.M., Lang P.J. Startle modulation during emotional anticipation and perception // Psychophysiology. 2014. № 10. P. 977–981.
- Weinberg A., Sandre A. Distinct associations between low positive affect, panic, and neural responses to reward and threat during late stages of affective picture processing // Biological Psychiatry: Cognitive Neuroscience and Neuroimaging. 2017.
- Karthikeyan P., Murugappan M., Yaacob S. A review on stress inducement stimuli for assessing human stress using physiological signals // 2011 IEEE 7th International Colloquium on Signal Processing and its Applications. 2011. P. 420–425.
- Marchewka A., Zurawski L., Jednorog K., Grabowska A. The Nencki Affective Picture System (NAPS): introduction to a novel, standardized, wide-range, high-quality, realistic picture database // Behavioral research methods. 2014. № 46. P. 596–610.
- Seo S.-H., Lee J.-T. Stress and EEG // Converg. Hybrid Inf. Technol. 2010.
- Choi Y., Kim M., Chun C. Measurement of occupants’ stress based on electroencephalograms (EEG) in twelve combined environments // Build. Environ. 2015. V. 88. P. 65–72.
- Alonso J.F. et al. Stress assessment based on EEG univariate features and functional connectivity measures // Physiol. Meas. 2015. V. 36. № 7. P. 1351–1365.
- Atencio A.C. et al. Computing stress-related emotional state via frontal cortex asymmetry to be applied in passive-ssBCI // 5th ISSNIP-IEEE Biosignals and Biorobotics Conference (2014): Biosignals and Robotics for Better and Safer Living (BRC). 2014. P. 1–6.
- Ryu K., Myung R. Evaluation of mental workload with a combined measure based on physiological indices during a dual task of tracking and mental arithmetic // Int. J. Ind. Ergon. 2005. V. 35. № 11. P. 991–1009.
- Tong S., Thakor N.V. Quantitative EEG analysis methods and clinical applications. 2009.
- Subhani A.R., Xia L., Malik A.S. EEG signals to measure mental stress // 2nd International Conference on Behavioral, Cognitive and Psychological Sciences. Maldives. 2011. P. 84–88.
- Aftanas L.I., Reva N.V., Varlamov A.A., Pavlov S.V., Makhnev V.P. Analysis of evoked EEG synchronization and desynchronization in conditions of emotional activation in humans: temporal and topographic characteristics // Neuroscience and behavioral physiology. 2004. № 8. P. 859–867.
- Nelson B.D., Hajcak G., Shankman S.A. Event-related potentials to acoustic startle probes during the anticipation of predictable and unpredictable threat // Psychophysiology. 2015. № 7. P. 887–894.
- Lin C.-T. et al. Nonparametric Single-Trial EEG Feature Extraction and Classification of Driver’s Cognitive Responses // EURASIP J. Adv. Signal Process. 2008. V. 2008. № 1. P. 849040.
- Chanel G. et al. Short-term emotion assessment in a recall paradigm // Int. J. Hum.-Comput. Stud. 2009. V. 67. № 8. P. 607–627.
- Hosseini S.A., Khalilzadeh M.A. Emotional Stress Recognition System Using EEG and Psychophysiological Signals: Using New Labelling Process of EEG Signals in Emotional Stress State // 2010 International Conference on Biomedical Engineering and Computer Science. 2010. P. 1–6.
- Saidatul A. et al. Analysis of EEG signals during relaxation and mental stress condition using AR modeling techniques // 2011 IEEE International Conference on Control System, Computing and Engineering. 2011. P. 477–481.
- Khosrowabadi R. et al. A Brain-Computer Interface for classifying EEG correlates of chronic mental stress // The 2011 International Joint Conference on Neural Networks. 2011. P. 757–762.
- Jun G., Smitha K.G. EEG based stress level identification // 2016 IEEE Int. Conf. Syst. Man Cybern. SMC. 2016. P. 003270–003274.
- Al-shargie F. et al. Towards multilevel mental stress assessment using SVM with ECOC: an EEG approach // Med. Biol. Eng. Comput. 2018. V. 56. № 1. P. 125–136.
- Shon D. et al. Emotional Stress State Detection Using Genetic Algorithm-Based Feature Selection on EEG Signals // Int. J. Environ. Res. Public. Health. 2018. V. 15. № 11.
- Vanitha V., Pandian Krishnan. Real time stress detection system based on EEG signals // Biomed. Res. 2016. V. 0. № 0.
- Ogino M., Mitsukura Y. Portable Drowsiness Detection through Use of a Prefrontal Single-Channel Electroencephalogram // Sensors. 2018. V. 18. № 12.
- Käthner I. et al. Effects of mental workload and fatigue on the P300, alpha and theta band power during operation of an ERP (P300) brain–computer interface // Biol. Psychol. 2014. V. 102. P. 118–129.
- Nguyen T. et al. Utilization of a combined EEG/NIRS system to predict driver drowsiness // Sci. Rep. 2017. V. 7. № 1. P. 1–10.
- Ma Y. et al. Driving Fatigue Detection from EEG Using a Modified PCANet Method [Electronic resource]: Research article // Computational Intelligence and Neuroscience. 2019. URL: https://www.hindawi.com/journals/cin/2019/4721863/ (accessed: 19.11.2019).
- Roohi-Azizi M. et al. Changes of the brain’s bioelectrical activity in cognition, consciousness, and some mental disorders // Med. J. Islam. Repub. Iran. 2017. V. 31. P. 53.
- Yeo M.V.M., Li X., Wilder-Smith E.P.V. Characteristic EEG differences between voluntary recumbent sleep onset in bed and involuntary sleep onset in a driving simulator // Clin. Neurophysiol. 2007. V. 118. № 6. P. 1315–1323.
- Грубов В.В., Овчинников А.А., Ситникова Е.Ю., Короновский А.А., Храмов А.Е. Вейвлетный анализ сонных веретен на ЭЭГ и разработка метода их автоматической диагностики // Нелинейная динамика и нейронаука. 2011. С. 91–108.
- Захаров Е.С. Автоматизированное распознавание стадий сна // Медицинская диагностика и терапия. 2008. С. 117–120.
- Schmitz A., Grillon C. Assessing fear and anxiety in humans using the threat of predictable and unpredictable aversive events (the NPU-threat test) // Nature Protocols. 2012. № 3. P. 527–532.
- Rhudy J.L., Meagher M.W. Noise stress and human pain thresholds: divergent effects in men and women // Journal of Pain. 2001. № 2. P. 57–64.
- Zunhammer M., Eberle H., Eichhammer P., Busch V. Somatic symptoms evoked by exam stress in university students: the role of alexithymia, neuroticism, anxiety and depression // PLOS One. 2013. № 12. P. 1–11.
- B., Basar E. A review of brain oscillations in perception of faces and emotional pictures // Neuropsychologia. 2014. № 58. P. 33–51.
- Антипов О.И. Захаров А.В. Повереннова И.Е. Неганов В.А. Ерофеев А.Е. Возможности различных методов автоматического распознавания стадий сна // Саратовский научно-медицинский журнал. 2012. Т. 8. № 2. С. 374 – 379.
- Rajendra Acharya U, Eric Chern-pin Chua, Kuang Chua, Lim Choo, Toshiyo Tamura Analysis and automatic identification of sleep stages using higher order spectra // International Journal of Neural Systems. 2010. № 20(6). P. 509–530.
- Zhumazhanova S.S. et al. Informativeness Assessment of the Thermal Pattern Features of the Face and Neck Region in the Tasks of Recognition of the Subject’s Changed State // 2019 20th International Conference of Young Specialists on Micro/Nanotechnologies and Electron Devices (EDM). 2019. P. 97–101.
- Cohen H.L., Porjesz B., Begleiter H. Ethanol-induced alterations in electroencephalographic activity in adult males // Neuropsychopharmacol. Off. Publ. Am. Coll. Neuropsychopharmacol. 1993. V. 8. № 4. P. 365–370.
- Lukas S.E., Mendelson J.H., Benedikt R. A., Jones B. EEG alpha activity increases during transient episodes of ethanol-induced euphoria // Pharmacol. Biochem. Behav. 1986. V. 25. № 4. P. 889–895.
- Saletu-Zyhlarz G.M. et al. Differences in brain function between relapsing and abstaining alcohol-dependent patients, evaluated by EEG mapping // Alcohol Alcohol. Oxf. Oxfs. 2004. V. 39. № 3. P. 233–240.
- Sun Y., Ye N., Xu X. EEG Analysis of Alcoholics and Controls Based on Feature Extraction // 2006 8th international Conference on Signal Processing. 2006. V. 1.
- Karungaru S. et al. Monotonous tasks and alcohol consumption effects on the brain by eeg analysis using neural networks // Int. J. Comput. Intell. Appl. 2012. V. 11. № 03. P. 1250015.
- Sarraf J., Chakrabarty S., Pattnaik P.K. EEG Based Oscitancy Classification System for Accidental Prevention // Proceedings of the 5th International Conference on Frontiers in Intelligent Computing: Theory and Applications / Ed. S.C. Satapathy et al. Singapore: Springer. 2017. P. 235–243.
- Tzimourta K.D. et al. Direct Assessment of Alcohol Consumption in Mental State Using Brain Computer Interfaces and Grammatical Evolution // Inventions. 2018. V. 3. № 3. P. 51.