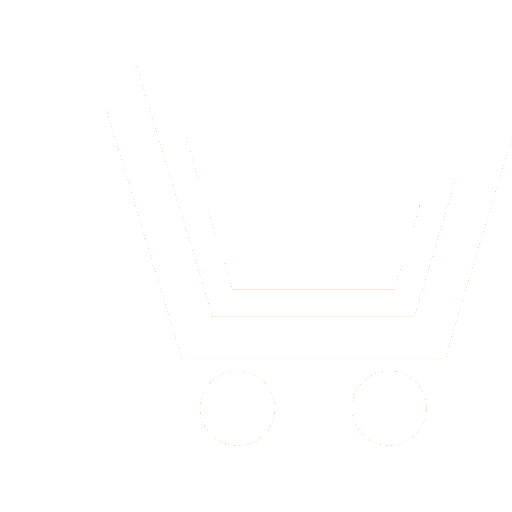
V. F. Los’1, I. O. Porokhov2, A. G. Gudkov3, I. A. Sidorov4
1 JSC “Radio Engineering Corporation “Vega” (Moscow, Russia)
2 Рeoples’ Friendship University of Russia (Moscow, Russia)
3, 4 Bauman Moscow State Technical University (Moscow, Russia)
3 Ltd. Hyperion (Moscow, Russia)
1 mail@vega.su
Rigorous analytical solutions to antenna synthesis problems have been obtained for several of their simplest designs, while numerical solutions to specific versions of these problems in a strict electrodynamic formulation for the required practical structures take so much time in most cases that obtaining an optimal solution in an acceptable time is not always possible. For this reason, approximate methods for solving various technical problems, including antenna problems, based on significantly simpler operators than in electrodynamics have become widespread. This allows for a significant gain in time and optimal solutions to synthesis problems in a reasonable time by direct, targeted enumeration of the space of combinations of parameters characterizing the problem under consideration. If it is necessary to increase the accuracy of the solution obtained in this way, approximate methods allow one to select from a possible number of options a significantly smaller number with the best values of the objective function for subsequent analysis of these options by strict methods.
A significant place among approximate optimization methods is occupied by evolutionary algorithms (EA), which have been developed since the second half of the last century. They imitate the behavior and evolution of various biological communities, generally obeying the theory of evolution and Darwin's natural selection.
The article provides a brief overview of modifications of both well-known algorithms and the control parameters they contain: the genetic algorithm (GA), the method of swarm of biological entities (PSO), the method of differential evolution (DE), with the help of which a number of problems on the synthesis of antennas with discretely and continuously changing parameters have been solved, and a relatively new algorithm – the method of grey wolves (GWO). An example of implementation using the PSO/FDTD optimization algorithm of a dual-frequency and broadband microstrip antenna with an E-shaped plate has been given. A mathematical model and a block diagram of the modified IGWO algorithm have been presented. The superiority of its characteristics in terms of the accuracy of determining the global extremum and the speed of convergence over such well-known algorithms as GWO, SCA, SSA, PSO, FPA, MFO and BA has been shown based on testing these algorithms on twenty-three reference functions.
Los’ V.F., Porokhov I.O., Gudkov A.G., Sidorov I.A. Methods for optimizing the characteristics of antenna elements and arrays simulating aggregative behavior and evolution of living beings in nature. Antennas. 2024. № 4. P. 50–66. DOI: https://doi.org/10.18127/ j03209601-202404-06 (in Russian)
- Los' V.F., Porokhov I.O., Agasieva S.V., Gudkov G.A. Perspektivy miniatyurizatsii razmerov elektricheski malykh antenn v usloviyakh ogranichenij na polosy rabochikh chastot. Elektromagnitnye volny i elektronnye sistemy. 2023. T. 28. № 4. S. 57–78. DOI: https://doi.org/ 10.18127/j15604128-202304-06. (in Russian)
- Wolpert D.H., Macready W.G. No free lunch theorems for optimization. IEEE Transactions on Evolutionary Computation. 1997. V 1. № 1. P. 67–82. DOI: 10.1109/4235.585893.
- Ho Y., Pepyne D. Simple explanation of the no-free-lunch theorem and its implications. Journal of Optimization Theory and Applications. 2002. V. 115. P.549–570. DOI: https://doi.org/10.1023/A:1021251113462.
- Rocca P., Oliveri G., Massa A. Differential evolution as applied to electromagnetic. IEEE Antennas and Propagation Magazine. 2011. V. 53. № 1. P. 38–49. DOI: 10.1109/MAP.2011.5773566.
- Goudos S.K., Kalialakis Ch., Mittra R. Evolutionary algorithms applied to antennas and propagation: a review of state of the art. International Journal of Antennas and Propagation. 2016. V. 2016. P. 1–12. DOI: 10.1155/2016/1010459.
- Yeo B.-K., Lu Y. Array failure correction with a genetic algorithm. IEEE Transactions on Antennas and Propagation. 1999. V. 47. № 5. P. 823–828. DOI: 10.1109/8.774136.
- Yang S., Gan Y.B., Tan P.K. Design of a uniform amplitude time modulated linear array with optimized time sequences. IEEE Transactions on Antennas and Propagation. 2005. V. 53. № 7. P. 2337–2339. DOI: 10.1109/TAP.2005.850765.
- Haupt R.L. Antenna design with a mixed integer genetic algorithm. IEEE Transactions on Antennas and Propagation. 2007. V. 55. № 3. P. 577–582. DOI: 10.1109/TAP.2007.891510.
- Zhu X., Shao W., Li J.-L., Dong Y.-L. Design and optimization of low RCS patch antennas based on a genetic algorithm. Progress in Electromagnetics Research. 2012. V. 122. P. 327–339. DOI: 10.2528/PIER11100703.
- Fu X., Chen X., Hou Q., Yin Y. An improved chaos genetic algorithm for T-shaped MIMO radar antenna array optimization. International Journal of Antennas and Propagation. 2014. V. 2014. DOI: https://doi.org/10.1155/2014/631820.
- Jayasinghe J.M.J.W., Anguera J., Uduwawala D., Andujar A. Nonuniform overlapping method in designing microstrip patch antennas using genetic algorithm optimization. International Journal of Antennas and Propagation. 2015. V. 2015. DOI: 10.1155/2015/805820.
- Clerc M. The swarm and the queen: towards a deterministic and adaptive particle swarm optimization. Proceedings of the 1999 Congress on Evolutionary Computation (CEC-99). Washington, D.C. 1999. V. 3. P. 1951–1957. DOI: 10.1109/CEC.1999.785513.
- Jin N., Rahmat-Samii Y. Parallel particle swarm optimization and finite-difference time-domain (PSO/FDTD) algorithm for multiband and wideband patch antenna design. IEEE Transactions on Antennas and Propagation. 2005. V. 53. № 11. P. 3459–3468. DOI: 10.1109/ TAP.2005.858842.
- Afshinmanesh F., Marandi A., Shahabadi M. Design of a dual-band dual-polarized printed microstrip antenna using a boolean particle swarm optimization. IEEE Transactions on Antennas and Propagation. 2008. V. 56. № 7. P. 1845–1852. DOI: 10.1109/TAP.2008. 924684.
- Panduro M., Brizuela C.A., Balderas L.I. A comparison of genetic algorithms, particle swarm optimization and the differential evolution method for the design of scannable circular antenna arrays. Progress in Electromagnetics Research B. 2009. V. 13. P. 171–186. DOI: 10.2528/PIERB09011308.
- Törn Ali M. Population set-based global optimization algorithms: Some modifications and numerical studies. Computers and Operations Research. 2004. V. 31. P. 1703–1725. DOI: 10.1016/S0305-0548(03)00116-3.
- Chakraborty U.K. Advances in differential evolution. Berlin: Springer. 2008.
- Qing A. Differential evolution. Fundamentals and applications in electrical engineering. Singapure: Wiley IEEE – Press. 2009.
- Qing A. Dynamic differential evolution strategy and applications in electromagnetic inverse scattering problem. IEEE Transactions on Geoscience and Remote Sensing. 2006. V. 44. № 1. P. 116–125. DOI: 10.1109/TGRS.2005.859347.
- Chen Y., Yang S., Nie Z. The application of a modified differential evolution strategy to some array pattern synthesis problem. IEEE Transactions on Antennas and Propagation. 2008. V. 56. № 7. P. 1919–1927. DOI: 10.1109/TAP.2008.924713.
- Mirjalili S., Mirjalili S.M., Lewis A. Grey wolf optimizer. Advances in Engineering Software. 2014. V. 69. P. 46–61. DOI: 10.1016/j.advengsoft. 2013.12.007.
- Faris H., Aljarah I., Azmi M., Al-Betar M.A., Mirjalili S. Grey wolf optimizer: a review of recent variants and applications. Neural Computing and Applications. 2018. V. 30. № 2. P. 413–435. DOI: https://doi.org/10.1007/s00521-017-3272.
- Saxena P., Kothari A. Optimal pattern synthesis of linear antenna array using grey wolf optimization algorithm. International Journal of Antennas and Propagation. 2016. P. 1–11. DOI: https://doi.org/10.1155/2016/1205970.
- Pappula L., Ghosh D. Linear antenna array synthesis using cat swarm optimization. AEU – International Journal of Electronics and Communications. 2014. V. 68. № 6. P. 540–549. DOI: 10.1016/j.aeue.2013.12.012.
- Rajo-Iglesias E., Quevedo-Teruel O. Linear array synthesis using ant-colony-optimization-based algorithm. IEEE Antennas and Propagation Magazine. 2007. V. 49. № 2. P. 70–79. DOI: 10.1109/MAP.2007.376644.
- Singh U., Kumar H., Kamal T.S. Linear array synthesis using biogeography based optimization. Progress in Electromagnetics Research M. 2010. V. 11. P. 25–36. DOI: 10.2528/PIERM09120201.
- Li Y., Lin X., Liu J. An improved grey wolf optimization algorithm to solve engineering problems. Sustainability. 2021. V. 13. № 6. DOI: 10.3390/su13063208.