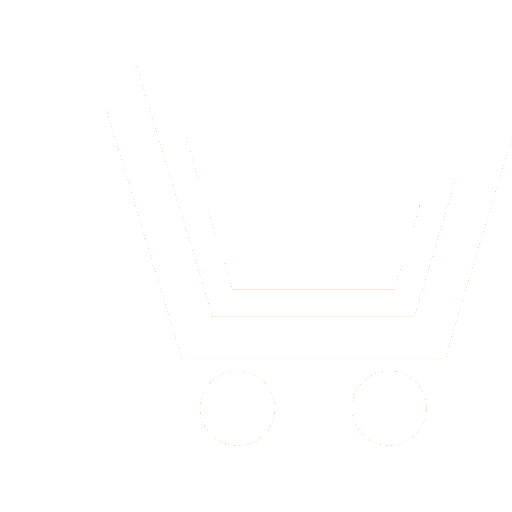
O. S. Litvinov – Dr.Sc. (Phys.-Math.), Professor,
Department of Physics, Bauman Moscow State Technical University
E-mail: oleglitv@mtu-net.ru
D. V. Murodyants – Student,
Department of Physics, Bauman Moscow State Technical University
E-mail: dmitriy1994.94@mail.ru
V. S. Boruta – Ph.D. (Phys.-Math.), Associate Professor,
Department of Physics, Bauman Moscow State Technical University
B. E. Vintaykin – Dr.Sc. (Phys.-Math.), Professor,
Department of Physics, Bauman Moscow State Technical University
The aim of this work was to create an adaptive antenna array (AAA) on neural network phase control, which maximizes the ratio of the power of the useful signal to noise at the output with minimal loss of power of the useful signal. The choice of a neural network as a control unit was determined by the difficulty of solving the problem of phase control of the AAA, which does not have a known analytical solution. Also, the tasks of the work included conducting studies of various noise suppression characteristics in an adaptive antenna array on neural network phase control and comparing the results obtained with the results of AAA operation on a common amplitude-phase control. The main considered characteristics were the dependence of the ratio of the power of the useful signal to noise at the output on the angle of arrival of the cluster from a different number of interferences, the dependence of the ratio of the powers of the useful signal at the AAA output of phase and total control on the angle, and the dependence of the S/N ratio on the angle of arrival of the interference as a result fluctuations in the synapses of the neural network. The success of the neural network in the problem of phase control of the AAA, its advantage in terms of the power loss of the useful signal for a different number of incoming noise, as well as noise immunity in relation to random fluctuations in the synapses of the neural network are demonstrated. It was also shown that AAA on neural network phase control is inferior in the task of maximizing S/N to the traditional control AAA because of the imposition of additional restrictions on the variation of the amplitudes of the weights. Based on the obtained S/N dependences, the criteria for the optimal angle of arrival of the interference were determined: the angle of arrival should not be close to π/2, since the received signal will have a high spatial frequency. The stability of AAA on neural network control to random fluctuations
in the elements of the neural network of the main control unit was also demonstrated.
- Pistol'kors A.A., Litvinov O.S. Vvedenie v teoriyu adaptivnykh antenn. M.: Nauka. 1991.
- Khajkin S. Nejronnye seti: Polnyj kurs. Izd. 2-e. M.: ID «Vil'yams». 2008.
- Uossermen F. Nejrokomp'yuternaya tekhnika: Teoriya i praktika. M.: Mir. 1992.
- Kalatskaya L.V., Novikov V.A., Sadov V.S. Organizatsiya i obuchenie iskusstvennykh nejronnykh setej. Eksperimental'noe ucheb. posobie. Minsk: Izd-vo BGU. 2003.
- Acharya N., Acharya S., Panda S., Nanda P. An artificial neural network model for a diesel engine fuelled with mahua biodiesel. Computational Intelligence in Data Mining: Proceedings of the International Conference on CIDM. 2017. P. 193–201. DOI: 10.1007/978981-10-3874-7_19
- Liu G., Jia L., Kong B., Guan K., Zhang H. Artificial neural network application to study quantitative relationship between silicide and fracture toughness of Nb-Si alloys. Materials & Design. 2017. V. 129. P. 210–218.
- Hinton G.E., Osindero S., Teh. Y.W. A fast learning algorithm for deep belief nets. Neural Computation. 2006. V. 18. № 7. P. 1527–1554. DOI: 10.1162/neco.2006.18.7.1527
- Deng G., Ferris M.C. Neuro-dynamic programming for fractionated radiotherapy planning. Springer Optimization and Its Applications. 2008. V. 12. P. 47–70.
- Islam Md.S., Mohandes M., Rehman S. Vertical extrapolation of wind speed using artificial neural network hybrid system. Neural Computing and Applications. 2017. V. 28. P. 2351–2361.
- Zinov'ev A.Yu. Vizualizatsiya mnogomernykh dannykh. Krasnoyarsk: Izd-vo Krasnoyarskogo gos. tekhn. un-ta. 2000.
- Liventsev V.V. Primenenie polyarizatsii signalov dlya povysheniya effektivnosti ispol'zovaniya radiochastotnogo spectra. Mobil'nye sistemy. 2007. № 7. S. 10–15.
- Zelkin E.G., Kravchenko V.F. Sintez antenn na osnove atomarnykh funktsij. M.: Radiotekhnika. 2003.