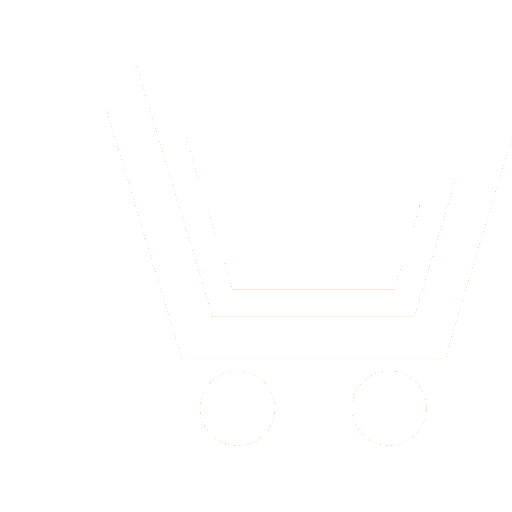
A.V. Budykina1, T.V. Zarubina2, S.E. Rauzina3, E.V. Tikhomirova4, E.D. Fedorov5, O.I. Yudin6, R.V. Plakhov7, M.V. Bordikov8
1–5,7 Pirogov Russian National Medical University (Moscow, Russia)
6 Clinical «K+31» (Moscow, Russia)
8 Clinical Hospital № 31 (Moscow, Russia)
Difficulties in the timely identification of the source of gastrointestinal bleeding affect the outcome and quality of medical care provided to patients with a surgical profile.
Development of an algorithm for differential diagnosis of gastrointestinal bleeding using the methods of mathematical statistics.
A multilevel algorithm for diagnosing patients with gastrointestinal bleeding is presented, the accuracy of which is 88-98%. The resulting rules include parameters that determine the clinic, localization and severity of gastrointestinal bleeding (21 parameters in total).
The created rules can be introduced into medical information systems of medical organizations to clinical decision support by medical specialists of surgical and gastroenterological profiles.
Budykina A.V., Zarubina T.V., Rauzina S.E.., Tikhomirova E.V., Fedorov E.D., Yudin O.I., Plakhov R.V., Bordikov M.V. Development of an automated algorithm for differential diagnostics of gastrointestinal bleeding using methods of mathematical statistics. Technologies of Living Systems. 2022. V. 19. № 2. Р. 46-56. DOI: https://doi.org/10.18127/j20700997-202202-05 (In Russian)
- Kotayev A.Yu. Zheludochno-kishechnyye krovotecheniya: Uchebnoye posobiye. Rostov-na-Donu: Feniks. 2015. S. 8–18. (in Russian).
- Rukovodstvo po skoroy meditsinskoy pomoshchi pri zheludochno-kishechnom krovotechenii. M.: Minzdrav RF. 2014. (in Russian).
- Besedina N.K., Glavnov P.V., Molostova A.S., Ermolenko E.I., Varzin S.A. Problema zheludochno-kishechnogo krovotecheniya (obzor literatury). Zdorovye – osnova chelovecheskogo potentsiala: problemy i puti ikh resheniya. 2020. T. 15. № 2. S. 738–754. (in Russian).
- Taveras L.R., Weis H.B., Weis J.J., Hennessy S.A. Acute Gastrointestinal Bleeding. In: Renton D., Lim R., Gallo A., Sinha P. (eds) The SAGES Manual of Acute Care Surgery. Springer. Cham. 2020. https://doi.org/10.1007/978-3-030-21959-8_7
- Domarev L.V. Kapsulnaya endoskopiya v diagnostike zabolevaniy tonkoy kishki. M.: Institut Khirurgii im. A. V. Vishnevskogo. 2007. (in Russian).
- Kobrinskiy B.A. Sistemy podderzhki prinyatiya resheniy v zdravookhranenii i obuchenii. Vrach i informatsionnyye tekhnolo-gii. 2010.
№ 2. S. 39–45. (in Russian). - Gavrilov E.L., Khomanov K.E., Korotkova A.V., Aslibekyan N.O., Shevchenko E.A. Aktualnyye napravleniya razvitiya spravoch-no-informatsionnykh on-layn prilozheniy dlya vrachey. Vestnik Natsionalnogo mediko-khirurgicheskogo tsentra im. N.I. Pi-rogova. 2017.
T. 12. № 1. S. 83–87. (in Russian). - Seo D.W., Yi H., Park B., Kim Y.J., Jung D.H., Woo I., Sohn C.H., Ko B.S., Kim N., Kim W.Y. Prediction of Adverse Events in Stable Non-Variceal Gastrointestinal Bleeding Using Machine Learning. J. Clin. Med. 2020. Aug 11. V. 9(8). P. 2603. DOI: 10.3390/jcm9082603
- Shung D., Laine L. Machine Learning Prognostic Models for Gastrointestinal Bleeding Using Electronic Health Record Data. Am. J. Gastroenterol. 2020. V. 115(8). P. 1199–1200. DOI: 10.14309/ajg.0000000000000720
- Shung D. Advancing care for acute gastrointestinal bleeding using artificial intelligence. Journal of Gastroenterology and Hepatology. 2021. V. 36. P. 273–278. DOI: 10.1111/jgh.15372
- Budykina A.V., Tikhomirova E.V., Kiselev K.V., Zarubina T.V., Rauzina S.E., Fedorov E.D., Yudin O.I. Formalizatsiya znaniy o zheludochno-kishechnom krovotechenii neyasnogo geneza dlya ispolzovaniya v intellektualnykh sistemakh podderzhki prinyatiya vrachebnykh resheniy. Vestnik novykh meditsinskikh tekhnologiy. 2020. № 4. S. 98–101. DOI: 10.24411/1609-2163-2020-16741 (in Russian).
- Budykina A.V. Razrabotka bazy znaniy ekspertnoy sistemy diagnostiki patsiyentov s zheludochno-kishechnymi krovotecheniyami. Integrirovannyye modeli i myagkiye vychisleniya v iskusstvennom intellekte. Sb. nauchnykh trudov X-y Mezhdunar. nauchno-tekhn. konf. (IMMV-2021. Kolomna. 17-20 maya 2021 g.). V 2-kh tomakh. T. 2. Smolensk: Universum. 2021. S. 303–310. (in Russian).
- Bekkenbakh E. Prikladnaya kombinatornaya matematika. Sb. statey. M.: 2015. 528 s. (in Russian).