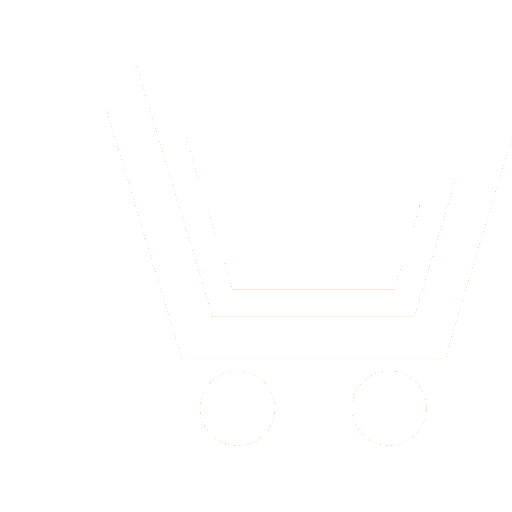
D.E. Zavelev1, M.V. Tsodikov2
1,2 A.V. Topchiev Institute of Petrochemical Synthesis of RAS (Moscow, Russia)
One of the most important trends of the development of modern industrial organic chemistry is changing its feedstock from fossil sources to renewable ones. As fossil feedstocks mostly contain hydrocarbons while renewable feedstocks mostly contain lipids and hydrocarbons, the change of feedstock requires the development of new industrial processes and thus the search of new catalysts, mostly heterogeneous ones. Despite a lot of various experimental investigation techniques exist, it’s not possible in many cases to understood the structure of active sites and the nature of catalytic activity only from experimental studies. Another problem is the prediction of candidate catalytic systems for their subsequent preparation and catalytic tests. In many cases for both kinds of tasks, the methods of computational quantum chemistry, mostly DFT, were used in recent 20-25 years.
Cluster DFT models usually include atoms of catalytically active sites and some surrounding atoms, several dozens of atoms being enough for modelling. The advantage of cluster method is relative easiness of transitional state search that allows one to estimate activation barriers. An example of studying bimetallic Pt–Sn/γ-Al2O3 hydrodeoxygenation catalysts is provided. It was experimentally determined that Pt–Sn(1:5)/γ-Al2O3 catalyst exhibits ~90% selectivity, while Pt–Sn(1:1, 1:3)/γ-Al2O3 and industrial Pt/γ-Al2O3 show 70% and less selectivity. Structural studies confirmed the presence of bimetallic PtSnx particles on the catalyst surface, but it was not possible to distinguish particular intermetallic particles. Several cluster models for particular possible intermetallic compounds. It was shown that for all the clusters activation barrier of hydrogen chemosorption was negligible as well as activation barrier of H transition between Pt atoms, while the barrier of H transition from Pt to Sn decreased as Sn content increased. Then all the possible reactions of model ester were studied for all the models considered. It was concluded that Pt or Pt-Sn catalyst with low Sn content allowed many reactions, most of which are accompanied by carbon mass loss, to take place, while Pt-Sn catalyst with high content has significantly higher barriers of unwanted reactions in comparison with the barrier of target reaction (hydrodeoxygenation, i.e. deoxygenation without carbon mass loss). Using the obtained data (e.g. structures of pre-reaction complexes, intermediates and especially transition states) it’s possible to solve “from the theory to the experiment” task for similar hypothetic catalytic systems, i.e. “noble metal – p-element” bimetallic systems those have several binary compounds.
Periodic DFT models allow to exclude scaling effect which can be important in many cases. Such models are based on model supercell containing several layers of atoms of chosen index surface. An example of studying bimetallic Pd–Cu/γ-Al2O3 alcohols homologation catalysts is provided. It was experimentally determined that Pd/γ-Al2O3 was a highly active and highly active catalyst, but it had very low stability. Pd2Cu3/γ-Al2O3 nanoalloy catalyst exhibits similar activity and selectivity and outstanding stability. CO poisoning was suspected to be the main way of catalyst deactivation, so it was important to understand which factors mostly influence CO adsorption energy decrease: a change of zone structure of the bimetallic as a whole or local effects associated with the dopant. Two kind of models were used: virtual crystal approach (VCA) and supercell approach (SCA). For both kinds of models d zone center positions and CO adsorption energies were calculated for the whole range of compositions. It appeared that for both models adding more than 50% (atomic) Cu to Pd made CO binding moderate from strong that could explain the stability of the catalyst. Also there were no correlation between CO adsorption energy and d zone center position except the dramatic changes of both values in the same range of concentrations. So it was concluded that doping action of Cu was mostly accounted for local effects. The study can also be expanded “from the theory to the experiment”, but the choice of candidate bimetallic system is really challenging.
Thus computational quantum chemistry supplements experimental studies in modern complex studies of heterogenous catalysts. But as any modelling can’t take in the account all the properties of considered objects, it’s impossible to completely substitute experimental studies by theoretical ones. Only wise combining of both kinds of method allows to obtain relevant results.
Zavelev D.E., Tsodikov M.V. Applications of computational quantum chemistry methods for investigations of heterogeneous catalytic reactions of conversion of organic substrates. Science Intensive Technologies. 2023. V. 24. № 6. P. 5−21. DOI: https://doi.org/ 10.18127/j19998465-202306-01 (in Russian)
- Queneau Y., Han B. Biomass: Renewable carbon resource for chemical and energy industry. The Innovation. 2022. V. 3. № 1. P. 100184. DOI: 10.1016/j.xinn.2021.100184.
- Anastas P.T., Zimmerman J.B. The periodic table of the elements of green and sustainable chemistry. Green Chemistry. 2019. № 21. P. 6545. DOI: 10.1039/C9GC01293A.
- Tupikin E.I. Obshchaya neftekhimiya. SPb.: Lan'. 2020 (in Russian).
- Kumar R.P., Gnansounou E., Raman J.K., Baskar G. (Ed.) Refining Biomass Residues for Sustainable Energy and Bioproducts. Academic Press. 2020. 617 p.
- Chorkendorf I., Najmantsvedrajt H. Sovremennyj kataliz i himicheskaya kinetika. Dolgoprudnyj: ID «Intellekt». 2010. 504 s. (in Russian).
- Takashiro A. (Ed.) Computational and Data-Driven Chemistry Using Artificial Intelligence Fundamentals Methods and Applications. Fundamentals, Methods and Applications. Elsevier. 2022. 264 p.
- Heredia C.L., Ferraresi-Curotto V., López M.B. Characterization of Ptn (n = 2–12) clusters through global reactivity descriptors and vibrational spectroscopy, a theoretical study. Computational Materials Science. 2012. V. 53. P. 18. DOI 10.1016/j.commatsci.2011.09.005.
- Zavelev D.E., Zhidomirov G.M., Codikov M.V. Teoreticheskoe izuchenie vzaimodejstviya vodoroda s malymi klasterami intermetallidov Pt–Sn. Kinetika i kataliz. 2018. T. 59, № 4. S. 404. DOI 10.1134/S0453881118040160 (in Russian).
- Chamsiev R.S., Danilov F.O., Flid V.R., Chmidt E.Yu. Teoreticheskoe modelirovanie vzaimodejstviya molekul fenilacetilena i stirola s poverhnost'yu Pd{111}. Izv. AN. Ser. him. 2017. № 12. C. 2234 (in Russian).
- Zavelev D.E., Zhidomirov G.M., Codikov M.V. Teoreticheskoe izuchenie deoksigenacii slozhnyh efirov na malyh klasterah intermetallidov Pt–Sn. Kinetika i kataliz. 2020. T. 61. № 1. S. 5. DOI 10.31857/S0453881120010153 (in Russian).
- Codikov M.V., Chistyakov A.V., Netrusov A.I. Produkty biomassy i ih prevrashcheniya v komponenty topliv i monomery. Saarbrucken: LAMBERT Academic Publishing. 2017. 181 s. (in Russian).
- Chistyakov A.V., Zharova P.A., Codikov M.V., Chapovalov S.S., Pasynskij A.A., Murzin V.Yu., Gekhman A.E., Moiseev I.I. Vysokoselektivnaya pererabotka rastitel'nogo masla v uglevodorody. DAN. 2015. T. 460. № 1. S. 57. DOI 10.7868/S0869565215010132 (in Russian).
- Chistyakov A.V., Krivencov V.V., Naumkin A.V., Pereyaslavcev A.Yu., Zharova P.A., Codikov M.V. Evolyuciya aktivnyh komponentov i kataliticheskie svojstva Pt-Sn/Al2O3-katalizatorov v reakcii selektivnoj deoksigenacii rastitel'nyh masel. Neftekhimiya. 2016. T. 56. № 4. S. 375. DOI 10.7868/S0028242116040043 (in Russian).
- Zharova P.A., Chistyakov A.V., Shapovalov S.S., Pasynskii A.A., Tsodikov M.V. Pt–Sn/Al2O3 catalyst for the selective hydrodeoxygenation of esters. Mendeleev Commun. 2018. V. 28. № 1. P. 91. DOI 10.1016/j.mencom.2018.01.031.
- Zavelev D.E., Tsodikov M.V., Chistyakov A.V., Nikolaev S.A. A periodic DFT study of CO adsorption over Pd–Cu alloy (111) surfaces. Research on Chemical Intermediates. 2022. V. 48. P. 853. DOI 10.1007/s11164-021-04635-z.
- Kozlowski J.T., Davis R.J. Heterogeneous Catalysts for the Guerbet Coupling of Alcohols. ACS Catal. 2013. V. 3. № 7. P. 1588. DOI 10.1021/cs400292f.
- Nielsen A.T., Houlihan W.J. The Aldol Condensation. Organic Reactions. American Cancer Society. 2011. P. 1. DOI 10.1002/0471264180.or016.01. ISBN 978-0-47-126418-7.
- Nikolaev S., Tsodikov M., Chistyakov A., Zharova P., Ezzgelenko D. The activity of mono- and bimetallic gold catalysts in the conversion of sub- and supercritical ethanol to butanol. J. Catal. 2019. V. 369. P. 501. DOI 10.1016/j.jcat.2018.11.017.
- Riittonen T., Toukoniitty E., Madnani D.K., Leino A.R., Kordas K., Szabo M., Sapi A., Arve K., Wärnå J., Mikkola J.P. One-Pot Liquid-Phase Catalytic Conversion of Ethanol to 1-Butanol over Aluminium Oxide: The Effect of the Active Metal on the Selectivity. Catalysts. 2012. V. 2. № 1. P. 68. DOI 10.3390/catal2010068.
- Jiang D., Wu X., Mao J., Ni J., Li X. Continuous catalytic upgrading of ethanol to n-butanol over Cu/CeO2/AC catalysts. Chem. Commun. 2016. V. 52. № 95. P. 13749. DOI 10.1039/c6cc05860d.
- Nikolaev S., Tsodikov M., Chistyakov A., Chistyakova P., Ezzhelenko D., Shilina M. PdCu nanoalloy supported on alumina: A stable and selective catalyst for the conversion of bioethanol to linear α-alcohols. Catal. Today. 2021. V. 379. P. 50. DOI 10.1016/j.cattod.2020.06.061.
- Bellaiche L., Vanderbilt D. Virtual crystal approximation revisited: Application to dielectric and piezoelectric properties of perovskites. Phys. Rev. B. 2000. V. 61. № 12. P. 7877. DOI 10.1103/physrevb.61.7877.
- Sakong S., Mosch C., Groß A. CO adsorption on Cu–Pd alloy surfaces: ligandversus ensemble effects. Phys. Chem. Chem. Phys. 2007. V. 9. № 18. P. 2216. DOI 10.1039/B615547B.
- Zhou Y., Che F., Liu M., Zou C., Liang Z., Luna P.D., Yuan H., Li J., Wang Z., Xie H., Li H., Chen P., Bladt E., Quintero-Bermudez R., Sham T.K., Bals S., Hofkens J., Sinton D., Chen G., Sargent E.H. Dopant-induced electron localization drives CO2 reduction to C2 hydrocarbons. Nat. Chem. 2018. V. 10. № 9. P. 974. DOI 10.1038/s41557-018-0092-x.