350 rub
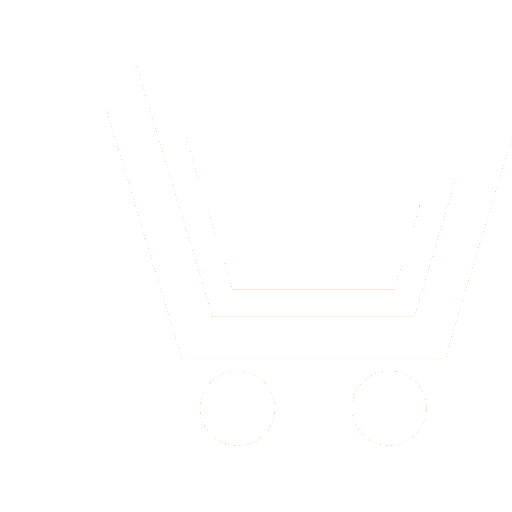
Journal Science Intensive Technologies №5 for 2013 г.
Article in number:
Analysis of objective functions for image distortion estimationA.G. Tashlinskii, S.V. Voronov
Keywords:
image distortion estimation
objective function
interframe correlation coefficient
mean squared difference
mutual information
Authors:
A.G. Tashlinskii, S.V. Voronov
Abstract:
A comparison of interframe correlation coefficient, mean squared difference and mutual information as objective functions for image distortion estimation is performed. Additive noise, linear and non-linear distortions of image brightness are investigated as interfering factors. Experimental studies were conducted on simulated and real images with different classes of interframe brightness distortions: additive noise, linear and nonlinear distortions. The results showed that the recursive estimation of interframe deformations for images that do not have multiplicative brightness distortions, as the objective function it is appropriate to use the mean squared difference. For small additive noise mutual information has the greatest slope that potentially provides greater speed and convergence of the parameter estimations. However, as the noise and the maximum slope of the characteristics of the mutual information sharply diminishes. Larger effective working range of estimation procedures is provided by interframe correlation coefficient and the mean squared difference. According to this criterion, the mutual information gives about half. For images of different modalities and image with linear brightness transformation, mutual information shows the best results, a little behind interframe correlation coefficient. However, given the significant gain coefficient interframe correlation computationally expensive relative to the mutual information, in most cases, the first is preferable. Finally, for images with significant non-linear luminance distortion, the only measure among the investigated that provides an acceptable efficiency was mutual information.
Pages: 16-21
References
- Brown L.G. A survey of image registration techniques // ACM C.S. 1992. V. 24. № 4. P. 325-376.
- De Castro E., Morandi C. Registration of translated and rotated images using finite Fourier transform // IEEE Transactions on Pattern Analysis and Machine Intelligence. 1987. V. 9. № 5. P. 700-703.
- Goshtasby A.A. Image registration. Principles, tools and methods // Advances in Computer Vision and Pattern Recognition. Springer 2012. 441 p.
- Tashlinskii A. Computational Expenditure Reduction in Pseudo-Gradient Image Parameter Estimation )/ Computational Science - ICCS 2003. V. 2658 // Proceeding. Part II. Berlin: Springer. 2003. P. 456-462.
- D\'Agostino E, Maes F, Vandermeulen D, Suetens P. An information theoretic approach for non-rigid image registration using voxel class probabilities / Med Image Anal. 2006. V 6(3). P. 413-431.
- Tashlinskijj A.G. Ocenivanie parametrov prostranstvennykh deformacijj posledovatelnostejj. Uljanovsk: Izd-vo UlGTU. 2000.
- Huntington E.V. Mathematics and statistics, with an elementary account of the correlation coefficient and the correlation ratio // Am. Math. Mon. 1919. V. 26(10). P. 421-435.
- Viola P., Wells III W.M. Alignment by maximization of mutual information // International Journal of Computer Vision. 1997. V. 24. P. 137-154.
- Vasilev K.K., Krasheninnikov V.R. Statisticheskijj analiz mnogomernykh izobrazhenijj. Uljanovsk: Izd-vo UlGTU. 2007.
- Tashlinskii A.G., Safina G.L., Voronov S.V. Pseudogradient optimization of objective function in estimation of geometric interframe image deformations // Pattern recognition and image analysis. 2012. V. 22. №. 2. P. 386-392.