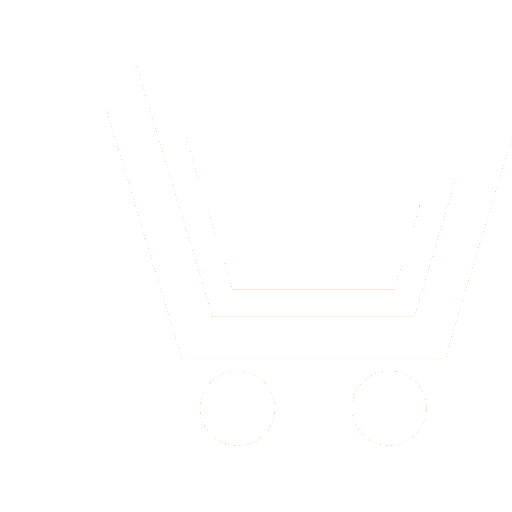
Y.S. Balashov1, S.N. Panychev2, M.V. Horoshaylova3
1,3 Voronezh State Technical University (Voronezh, Russia)
2 АО «Научно-технический центр радиоэлектронной борьбы» (г. Воронеж, Россия)
Formulation of the problem. Neural network decoders for quantum error correction codes have recently attracted great interest, especially in the context of topological codes. But for these codes, optimal (or very fast suboptimal) decoding algorithms have already been proposed, using their correct trellis structure. Before training, the BP decoder performance is worse for large code sizes at all levels of physical errors. After training, performance improves significantly for all code sizes at all levels of physical errors, and performance curves begin to intersect with each other. This indicates the development of the threshold. After training, performance increases with size for reasonably low error rates, and this trend indicates that further improvements in training may lead to a BP decoder with a finite threshold. Learning greatly improves the performance of BP decoders for all code families that have been tested and can solve the degeneration problem that prevents decoding of quantum LDPC codes.
Purpose. Analysis, development and training of neural BP (from English Neural Believeration, NBP) decoder for quantum LDPC codes.
To guide the learning process, we construct a loss function that takes into account the degeneracy of errors.
Practical significance. Propagation of confidence (BP) decoders play an important role in modern coding theory, but they are not suitable for decoding quantum error-correcting codes due to a quantum function called error degeneracy. Using the exact mapping properties between BP and deep neural networks, here we train BP neural decoders for low density parity check (LDPC) quantum codes with a loss function adapted for degenerate errors. There is a fundamental difference between classical and quantum error correction. In the classical case, successful decoding means that the estimated error einf is exactly the same as the true error e; while in the quantum case only the total error is required etot: = e + einf: mod 2 belonging to the “stabilizer group” - the set of all Pauli string operators spanned by H. This is due to the fact that two Pauli strings are operators E and F = ESj, differing by the stabilizer, have the same effect on all states of the code. To check if there is a stabilizer group etot, you just need to check that it commutes with all operators that commute with stabilizers, that is, where is the matrix that generates the orthogonal complement to H with respect to the symplectic dot product,. It has been shown that significant improvement can be achieved in the range of physical errors. When using the original PSU, an increase in the code size leads to a decrease in performance.
Balashov Yu.S., Panychev S.N., Horoshaylova M.V. Neural decoders for quantum codes. Radiotekhnika. 2021. V. 85. № 6. P. 17−22. DOI: https://doi.org/10.18127/j00338486-202106-03 (In Russian)
- Horoshajlova M.V. Arhitektura kanal'nogo kodirovanija na osnove PLIS dlja 5G besprovodnoj seti s ispol'zovaniem vysokourovnevogo sinteza. Vestnik Voronezhskogo gosudarstvennogo tehnicheskogo universiteta. 2018. T. 14. № 2. S. 99-105 (In Russian).
- Poulin D., Chung Y. On the iterative decoding of sparse quantum codes. 2008. arXiv:0801.1241.
- Yun-Jiang Wang, Sanders Barry C., Bao-ming Bai, Xin-mei Wang. Enhanced Feedback Iterative Decoding of Sparse Quantum Codes. IEEE Trans. Inf. Theory. 2012. № 58. Р. 1231–1241.
- Horoshajlova M.V. Arhitektura dlja stohasticheskih LDPC-dekoderov c ispol'zovaniem jeffektivnoj ploshhadi kristalla na osnove PLIS. Vestnik Voronezhskogo gosudarstvennogo tehnicheskogo universiteta. 2018. T. 14. № 1. S. 95-100 (In Russian).
- Bashkirov A.V., Horoshajlova M.V. Algoritmy nizkoj slozhnosti dekodirovanija i arhitektura dlja nedvoichnyh nizkoplotnostnyh kodov. Radiotehnika. 2016. № 6. S. 10-14 (In Russian).
- Bashkirov A.V., Muratov A.V., Horoshajlova M.V., Sitnikov A.V., Ermakov S.A. Nizkoplotnostnye kody maloj moshhnosti dekodirovanija. Radiotehnika. 2016. № 5. S. 32-37 (In Russian).