350 rub
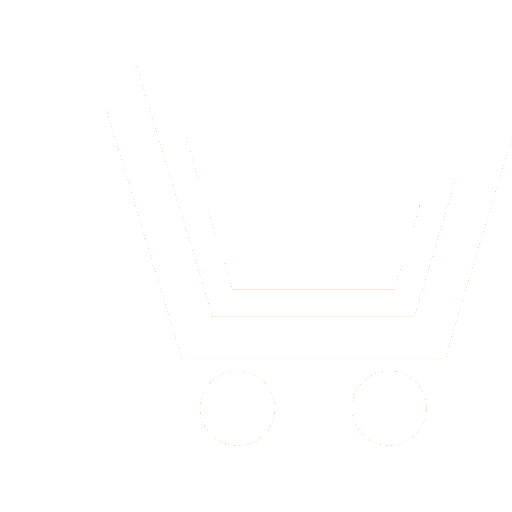
Journal Radioengineering №11 for 2014 г.
Article in number:
Effectiveness analysis of signal parameters estimation algorithm based on particle filtering through simulation
Keywords:
particle filter
error variance
Kalman filter
covariance matrix
conditional probability density function
Authors:
M.N. Sluzhivyi - Ph.D. (Eng.), Assistant Professor of Telecommunications dept. E-mail: m.sluzhivyi@ulstu.ru
S.M. Namestnikov - Ph.D., Assistant Professor of Telecommunications dept. E-mail: sernam@ulstu.ru
S.M. Namestnikov - Ph.D., Assistant Professor of Telecommunications dept. E-mail: sernam@ulstu.ru
Abstract:
Algorithms of recurrent estimation of signal parameters by means of particle filter are considered. Estimation error variance through simulation is carried out. It is shown that particle filter application enables to essentially increase estimation accuracy in certain spans of parameters values.
Particle filtering algorithms relate to Bayesian filtering methods and are based on sequential Monte Carlo sampling and consequent calculation and weighed summation of current values of conditional probability density function. These algorithms enable to effectively solve essentially nonlinear problems of non-Gaussian signal estimation.
It is also shown that experimental values of Kalman filtering error variance significantly exceed the theoretical bound whereas particle filter processing the same experimental data produces values of error variance close to the theoretical bound. The considered particle filtering algorithm enables to obtain estimates close to optimal ones and that is particularly clear at strong correlations of the desired signal. The obtained results can be applied when developing signal processing algorithms for perspective communication systems and moving vehicle navigation systems using data from terrain relief.
Pages: 79-81
References
- Doucet A., Godsill S., Andrieu C. On sequential Monte Carlo sampling methods for Bayesian filtering // Statistics and Computing. 2000. V. 10. P. 197-208.
- Haug A.J. Bayesian Estimation and Tracking: A Practical Guide. John Wiley & Sons. Inc. 2012. 369 p.
- Durrant-Whyte H., Bailey T. Simultaneous Localization and Mapping (SLAM): Part 1. The Essential Algorithms // IEEE Robotics and Automation Magazine. June. 2006. P. 99-108.