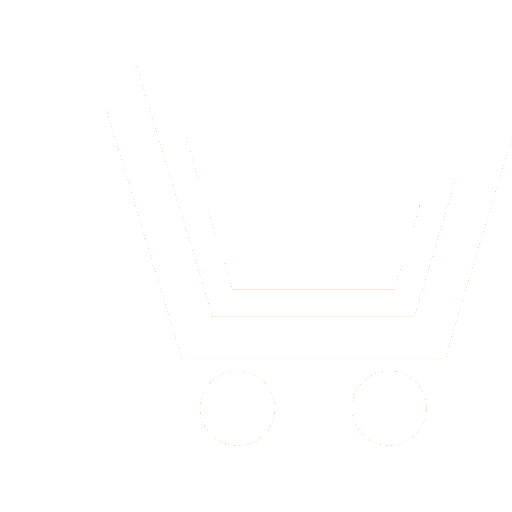
G.N. Zholobova1, E.D. Sinitsyna2
1,2 Financial University under the Government of the Russian Federation (Moscow, Russia)
1 gnzholobova@fa.ru, 2 221766@edu.fa.ru
Analyzing employee loyalty and the sentiment of their reviews is a crucial task for corporate analysts and HR specialists. The accuracy of these analyses directly depends on the quality of text data preprocessing, which is currently insufficiently studied and requires deeper attention. This study aims to substantiate methods for improving the quality of sentiment analysis and employee loyalty assessment by identifying the most effective preprocessing techniques for machine learning models such as BERT, DistilBERT, and RoBERTa. The research provides practical recommendations for text data preprocessing, including cleaning, normalization, stop-word removal, stemming, and lemmatization. It was demonstrated that optimal preprocessing can achieve an accuracy of up to 91% for the RoBERTa model. This research identified key text preprocessing methods that significantly enhance the accuracy of machine learning models in analyzing employee loyalty. Optimizing such preprocessing processes as text cleaning, normalization, stop-word removal, and decoding professional jargon allows for significantly improved sentiment analysis of employee reviews. These findings can be integrated into analytical systems used by HR specialists and corporate analysts to achieve more accurate sentiment and engagement analysis, ultimately contributing to better corporate culture management and increased overall employee satisfaction.
Zholobova G.N., Sinitsyna E.D. The impact of text preprocessing methods on the efficiency of machine learning models for employee loyalty analysis. Nonlinear World. 2024. V. 22. № 4. P. 102–107. DOI: https://doi.org/10.18127/ j20700970-202404-13 (In Russian)
- Rezapova D.B. Vovlechennost' sotrudnikov v rabotu. Universitetskoe upravlenie: praktika i analiz. 2016. № 1 (101). URL: https://cyberleninka.ru/article/n/vovlechennost-sotrudnikov-v-rabotu (In Russian).
- Miriam Alzate, Marta Arce-Urriza, Javier Cebollada. Mining the text of online consumer reviews to analyze brand image and brand positioning. 2022. URL: https://www.sciencedirect.com/science/article/pii/S0969698922000820.
- Bunina E.V. Innovacionnye metody upravleniya personalom. 2019. URL: https://cyberleninka.ru/article/n/innovatsionnye-metody-upravleniya-personalom-1 (In Russian).
- Majed A. Alshamari. Evaluating User Satisfaction Using Deep-Learning-Based Sentiment Analysis for Social Media Data in Saudi Arabia’s Telecommunication Sector. 2023. URL: https://www.mdpi.com/2073-431X/12/9/170.
- Bakhshi A., Kumar K. Organizational Commitment as predictor of Organizational Citizenship Behavior. URL: https://core.ac.uk/download/pdf/234624049.pdf.
- Onuchin A. Vovlechennost' personala: ot izmereniya k upravleniyu. URL: https://www.ecopsy.ru/insights/vovlechennost-personala-ot-izmereniya-k-upravleniyu/ (In Russian).
- Qurvenianto H.A., Tobing D.S.K., Handriyono The Effect Of Employee Loyalty And Work Creativity On Work Performance Of Employee Through Career Development PT. Astra International, Tbk Isuzu Malang.
- Narariya Dita Handani, Angellie Williady, Hak-Seon Kim. An Analysis of Customer Textual Reviews and Satisfaction at Luxury Hotels in Singapore’s Marina Bay Area (SG-Clean-Certified Hotels). 2022. URL: https://www.mdpi.com/2071-1050/14/15/9382.
- Oehler K. Модель вовлеченности сотрудников Kincentric. Aon Hewitt. URL: https://axes.ru/articles/model-vovlechennosti-sotrudnikov-aon-hewitt/.
- Allen N.J., Meyer J.P. The measurement and antecedents of affective, continuance and normative commitment to the organization. Journal of occupational psychology/ 1990. V. 63(1). P. 1–18.
- Tom Young, Devamanyu Hazarika, Soujanya Poria, Erik Cambria. Recent Trends in Deep Learning Based Natural Language Processing. 2020.