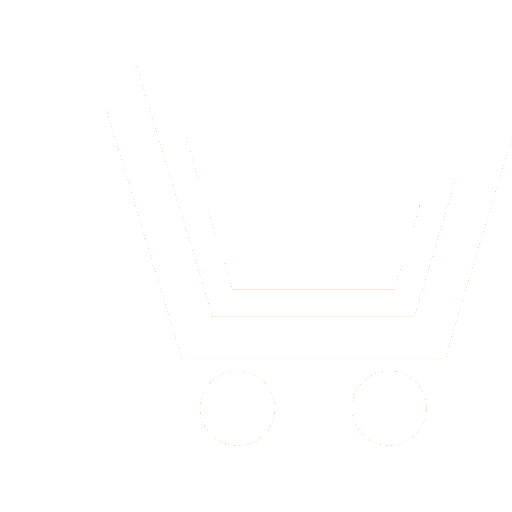
A.A. Petrov1, O.V. Druzhinina2, O.N. Masina3
1, 3 Bunin Yelets State University (Yelets, Russia)
2 FRС «Computer Science and Control» of RAS (Moscow, Russia)
The development of algorithmic support for modeling nonlinear control switching systems is an actual problem. Promising methods for solving this problem include methods of optimal control and methods of intellectual analysis. The purpose of this paper is to develop an approach to modeling nonlinear control switching systems based on the synthesis of generalized models and taking into account the development of new algorithmic support and the use of intelligent methods. The construction of models of generalized nonlinear control switching systems is proposed. The switching methodology for the studied models is described. A symbolic tree is developed to formalize the generalized model with switching. A new control quality criterion is proposed, which is associated with geometric restrictions imposed on the possible trajectories of the studied dynamic system. Such a combined model of a switching system is proposed, which is based on a combination of polynomial approximation and linear feedback. The formalized apparatus of a Markov process with discrete time is used to model perturbations. A block diagram of a generalized reinforcement learning algorithm for modeling switching is created. New combined switching algorithms are proposed using combinations of different types of controllers. The possibilities of implementing the developed switching algorithms are analyzed. The practical significance of the results lies in the fact that the proposed algorithmic support can be used in the problems of modeling nonlinear technical systems of intelligent control, in particular, control systems for aircraft and transport systems. The obtained results can be used in various problems of intelligent modeling and machine learning.
Petrov A.A., Druzhinina O.V., Masina O.N. Development of algorithmic support for modeling nonlinear control switching systems. Nonlinear World. 2022. V. 20. № 1. P. 5-13. DOI: https://doi.org/10.18127/j20700970-202201-01 (In Russian)
- Liberzon D., Morse A.S. Basic problems in stability and design of switched systems. IEEE Control syst. 1999. V. 19. № 5.
P. 59–70. - Vasil'ev S.N., Malikov A.I. O nekotoryh rezul'tatah po ustojchivosti perekljuchaemyh i gibridnyh sistem. Aktual'nye problemy mehaniki sploshnoj sredy. Kazan': Foliant. 2011. T. 1. S. 23–81 (In Russian).
- Shpilevaja O.Ja., Kotov K.Ju. Perekljuchaemye sistemy: ustojchivost' i proektirovanie (obzor). Avtometrija. 2008. T. 44. № 5. S. 71−87 (In Russian).
- Druzhinina O.V., Masina O.N., Petrov A.A. The synthesis of the switching systems optimal parameters search algorithms. Communications in Computer and Information Science. 2019. V. 974. P. 306–320.
- Druzhinina O.V., Masina O.N., Petrov A.A., Lisovsky E.V., Lyudagovskaya M.A. Neural network optimization algorithms for controlled switching systems. Advances in Intelligent Systems and Computing. 2020. V. 1225. P. 470–483.
- Druzhinina O.V., Korepanov Je.R., Belousov V.V., Masina O.N., Petrov A.A. Razvitie instrumental'nogo obespechenija otechestvennoj vychislitel'noj platformy «Jel'brus 801-PC» v zadachah nejrosetevogo modelirovanija nelinejnyh dinamicheskih sistem. Nelinejnyj mir. 2021. T. 19. № 1. S. 15−28 (In Russian).
- Masina O.N., Petrov A.A., Druzhinina O.V., Rapoport L.B. Modelirovanie upravljaemyh sistem s primeneniem metodov stabilizacii i algoritmov poiska optimal'nyh traektorij: ucheb. posobie. Elec: Eleckij gosudarstvennyj universitet im. I.A. Bunina. 2021. 117 s. (In Russian).
- Pinter J.D. Global optimization: software, test problems, and applications. Handbook of Global Optimization. Boston: Kluver. 2002. P. 515–569.
- Karpenko A.P. Sovremennye algoritmy poiskovoj optimizacii. M.: MGTU im. N.Je. Baumana. 2017. 447 s. (In Russian).
- Gudfellou Ja., Bendzhio I., Kurvill' A. Glubokoe obuchenie. M.: DMK-Press. 2017. 652 s. (In Russian).
- Druzhinina O.V., Masina O.N., Petrov A.A. Up-to-date software and methodological support for studying models of cont-
rolled dynamic systems using artificial intelligence. Lecture Notes in Networks and Systems (LNNS). Springer, 2021. V. 228.
P. 670–681. - Petrov A.A. Struktura programmnogo kompleksa dlja modelirovanija tehnicheskih sistem v uslovijah perekljuchenija rezhimov raboty. Jelektromagnitnye volny i jelektronnye sistemy. 2018. T. 23. № 4. S. 61–64 (In Russian).
- Kashirina I.A., Demchenko M.V. Issledovanie i sravnitel'nyj analiz metodov optimizacii, ispol'zuemyh pri obuchenii nejronnyh setej. Vestnik VGU. Ser. Sistemnyj analiz i informacionnye tehnologii. 2018. № 4. S. 123–132 (In Russian).
- Masina O.N., Petrov A.A., Druzhinina O.V., Chernomordov S.V., Openkin D.Y. Algorithms for switching technical systems modeling. CEUR Workshop Proceedings. 2021. V. 2922. P. 109–118 (Proceedings of the II International Conference «Information technologies and intellectual decision-making systems» ITIDMS-II-2021. Moscow. Russia. July 1 2021).
- Ang K.H., Chong G., Li Y. PID control system analysis, design, and technology. IEEE Transactions on Control Systems Technology. 2005. V. 13(4). P. 559–576.