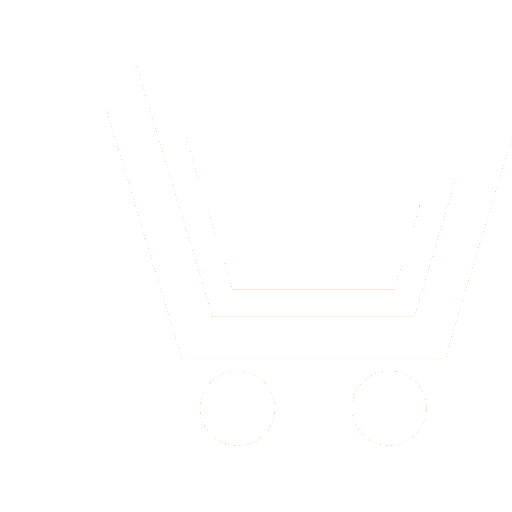
S.V. Sokolov1, E.G. Chub2, A.A. Manin3
1 North Caucasus Branch of Moscow Technical University of Communications and Informatics (Rostov-on-Don, Russia)
2 Don State Technical University (Rostov-on-Don, Russia)
Annotation. Currently, the problem of evaluating stochastic processes observed under noisy conditions on a finite time interval is solved only for datasets in the form of time series using a limited number of statistical variational or spectral analysis methods, as well as various modifications of regression methods. In this case, parametric criteria are used that depend on individual parameters of the distribution density of the observed process, and not on the density itself, which significantly limits the possibilities of increasing the estimation accuracy. To solve the problem of high-precision estimation of stochastic processes on a finite time interval of their observation, an approach is proposed, firstly, providing optimal estimation according to the criterion depending on the posterior distribution density - the most informative characteristic of the observed process, and secondly, taking into account the dynamic structure of the process and the finiteness of the interval observation. A numerical example is considered to illustrate the effectiveness of the developed approach.
Relevance. Currently, the problem of evaluating stochastic processes observed under noisy conditions on a finite time interval (terminal filtering problem) is solved only for datasets in the form of time series using a limited number of statistical variational or spectral analysis methods, as well as various modifications of regression methods. In this case, parametric criteria are used that depend on individual parameters of the distribution density of the observed process, and not on the density itself, which significantly limits the possibilities of increasing the estimation accuracy.
Target. In this regard, for stochastic processes of a general form - described by nonlinear stochastic differential equations, it is necessary to develop a method of terminal filtering according to a criterion that takes into account the finiteness of the observation time interval and depends on the posterior distribution density - the most informative characteristic of the observed process (and not on its individual parameters).
Results. The proposed solution to the problem of high-precision terminal filtering of stochastic processes - their optimal estimation over a finite observation time interval - is based on the use of a terminal criterion that depends directly on the posterior distribution density and takes into account the finiteness of the observation time interval. When describing the observed stochastic processes, their most general representation was used - nonlinear stochastic differential equations, which significantly expands the field of application of the results obtained in comparison, for example, with time series. The general solution to the problem of optimal terminal filtering is obtained using the Pontryagin maximum principle, the solution to the problem of suboptimal filtering, which significantly reduces computational costs, is based on the method of invariant immersion. Practical significance. A numerical example is considered to illustrate the effectiveness of the developed method. The proposed approach can be widely used in various fields of scientific and technical research: radio engineering, Earth sensing, satellite navigation, astronomy, seismology, geodesy, etc.
Sokolov S.V., Chub E.G., Manin A.A. Terminal filtering of stochastic processes observed on a finite time interval. Nonlinear World. 2021. V. 19. № 3. 2021. P. 57−67. DOI: https://doi.org/10.18127/j20700970-202103-06 (In Russian)
- Lohman A.W., Weigelt G., Wiruitzer B. Speckle masking in astronomy: triple correlation theory and applications. Applied Optics. 1983. V. 22. Р. 4028−4037.
- Jenkin A.B. DEBRIS: A Computer Program for Debris Cloud Modeling. Paper No. IAA.6.3-93-746. 44th Congress of the International Austronautical Federation. Graz, Austria. 1993.
- Vasilenko V.F., Prytkov A.S. Modelirovanie vzaimodejstvija litosfernyh plit na o. Sahalin po dannym GPS nabljudenij. Tihookeanskaja geologija. 2012. T. 31. № 1. S. 42-48 (In Russian).
- Ljubushin A.A. Analiz dannyh sistem geofizicheskogo i jekologicheskogo monitoringa. M.: Nauka. 2007. 228 s. (In Russian).
- Totsky A.V., Gorbunenko B.F. Investigations of the synthetic aperture radar images formed by processing of bispectral data. International Journal of Electronics and Communications. 1999. V. 53. № 3. Р. 146−150.
- Savinyh V.P., Cvetkov V.Ja. Geoinformacionnyj analiz dannyh distancionnogo zondirovanija. M.: Kartgeocentr – Geodezizdat. 2001. 228 s. (In Russian).
- Chandra A.M., Gosh S.K. Distancionnoe zondirovanie i geograficheskie informacionnye sistemy. M.: Tehnosfera. 2008. 312 s. (In Russian).
- Anshakov G.P., Goljakov A.D., Petrishhev V.F., Fursov V.A. Avtonomnaja navigacija kosmicheskih apparatov. Samara: Izd-vo GNPRKC «CSKB-Progress., 2011. 569 s. (In Russian).
- Gurevich G., Wertz J.R. Autonomous On-board Orbit Control Flight Results and Applications. AIA Apaper 2000-5226.-Long Beach, CA. 2000. P. 19−21.
- Spiridonov A.I. Osnovy geodezicheskoj metrologii. M.: Kartgeocentr − Geodezizdat. 2003. 247 s. (In Russian).
- Boks Dzh., Dzhenkins G. Analiz vremennyh rjadov prognoz i upravlenie. M.: SINTEG. 2002. 178 s. (In Russian).
- Simchera V.M. Metody mnogomernogo analiza statisticheskih dannyh. M.: Finansy i statistika. 2008. 400 c. (In Russian).
- Kulaichev A.P. Metody i sredstva kompleksnogo analiza dannyh. M.: Forum. 2018. 160 s. (In Russian).
- Kumkov S.I., Zholen L. Sravnenie metodov interval'nogo analiza i statisticheskih metodov v zadache ocenivanija jeksperimental'nyh dannyh s neopredelennost'ju. Izmeritel'naja tehnika. 2019. № 2. S. 13−17 (In Russian).
- Krivenko M. P. Software of research in statistical data analysis. Systems and Means of Informatics. 2020. V. 30. Is. 4. Р. 4−13.
- Brillindzher D. Vremennye rjady. Obrabotka dannyh i teorija. M.: Mir. 1980. 310 s. (In Russian).
- Rudoj G.I. Modifikacija funkcionala kachestva v zadachah nelinejnoj regressii dlja ucheta geteroskedastichnyh pogreshnostej izmerjaemyh dannyh. Informatika i ee primenenija. 2017. T. 11. № 2. S. 74−84 (In Russian).
- Mirkin B.G. Vvedenie v analiz dannyh. Ljubercy: Jurajt. 2016. 174 s. (In Russian).
- Afanas'ev V.N., Lebedeva T.V. Modelirovanie i prognozirovanie vremennyh rjadov. M.: Finansy i statistika. 2009. 292 s. (In Russian).
- Tihonov V.I., Harisov V.N. Statisticheskij analiz i sintez radiotehnicheskih ustrojstv i sistem. M.: Radio i svjaz'. 1991. 608 s. (In Russian).
- Pugachev V.S., Sinicyn I.N. Stohasticheskie differencial'nye sistemy. Analiz i fil'tracija. M.: Nauka. 1990. 462 s. (In Russian).
- Sinicyn I.N. Fil'try Kalmana i Pugacheva. M.: Universitetskaja kniga, Logos. 2006. 640 s. (In Russian).
- Sokolov S.V., Kovalev S.M., Kucherenko P.A., Smirnov Ju.A. Metody identifikacii nechetkih i stohasticheskih sistem. M: Fizmatlit. 2018. 432 s. (In Russian).
- Chernov A.A., Jastrebov V.D. Metod ocenki vozmushhenij v algoritmah reshenija navigacionnyh zadach. Kosmicheskie issledovanija. 1984. T. 22. № 3 (In Russian).
- Pervachev S.V., Perov A.I. Adaptivnaja fil'tracija soobshhenij. M.: Radio i svjaz'. 1991. 160 s. (In Russian).
- Sejdzh Je., Mels Dzh. Teorija ocenivanija i ee primenenie v svjazi i upravlenii. M.: Svjaz'. 1976. 496 s. (In Russian).