350 rub
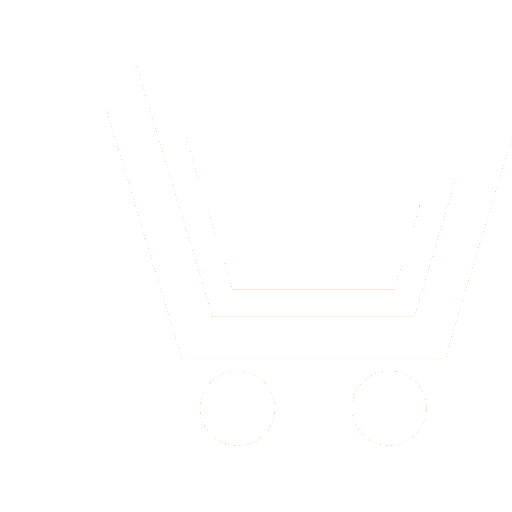
Journal Nonlinear World №3 for 2017 г.
Article in number:
Handwriting examination based on radial basis neural networks Pearson-Hamming
Keywords:
handwriting examination
chi-square distribution
neural network
biometrics
heavily dependent continuous data
heavily dependent output code of the state of
the probability of error of handwriting examination
Authors:
A.Kh. Gilmutdinov - Dr.Sc. (Eng.), Professor, Department of Radio Electronics and information and Measuring Equipment, Kazan National Research Technical University n.a. A.N. Tupolev - KAI (KNRTU - KAI); Head of the Industry Certification Center, JSC «Scientific-production Association «Radioelectronics» to them. V.I. Shimko»
E-mail: agilmutdinov@rambler.ru
Ye.I. Kachaykin - Adviser of the Department of Information Security Management and Control of the Ministry of Justice of the Russian Federation
E-mail: kachajkin@gmail.com
A.I. Ivanov - Dr.Sc. (Eng.), Associate Professor, Head of the Laboratory of Biometrics and Neural Network Technologies, Penza Scientific Research Electrotechnical Institute
E-mail: ivan@pniei.penza.ru
A.V. Bezyaev - Ph.D. (Eng.), Leading Specialist, Penza Branch (Federal State Unitary Enterprise Scientific-Technical Center«Atlas») FSUE STC «Atlas»
E-mail: bezyaev_alex@mail.ru
Abstract:
In this paper the method of automated expert handwriting analysis, which can take into account the hundreds of biometric parameters and at the same time to evaluate the probabilities of errors of the first and second kind.
Normal handwriting examination carried out by people, the experts, accounts for about 16 parameters. Each accounted for experts-people option is meaningful and well understood by people. At the automated handwriting examination the big artificial neural networks considering 416 biometric parameters, making sense senior factors of a matrix of two-dimensional transformation of Fourie can be used. How are these biometric data is difficult to imagine a person, but they are easily processed by artificial neural networks.
The Reader of the article may independently obtain biometric parameters autograph or handwriting using freely available simulation environment «BioNeyroAvtograf» http://пниэи.рф/activity/science/noc.htm. This simulation environment has been specially created in laboratory of biometrics and neural network technologies of JSC «Penza Research Electrotechnical Institute» for universities in Russia, Kazakhstan and Belarus. For this reason, in the formulas, the article provides the formula with the summation over 416 parameters and 208 options (half of 416). This form emphasizes the fact record high dimension of the problem being solved by neural networks biometric handwriting examination.
The paper discusses the use of radial basis neural networks or Pearson chi-square neurons. The undeniable advantage of radial basis neural networks is that they have an analytical description of the probabilities of errors of the first and second kind. However, this description is not suitable for the actual biometric data having a strong correlation.
To take into account the strong correlation of data requested to implement multidimensional symmetrization of biometric data, replacing them with equivalent value of the entropy data. It is proposed to synthesize data from the same (equal) correlation coefficients. This technique makes it possible to simplify the task before the effect of correlation of biometric data in the probability of errors of the first and second kind. The method of simple simulation dependent data for assessing the distribution of values of the chi-square dependent data with equal correlated.
It is shown that taking into account correlations leads to the fact that the degree of freedom chi-square distributions ceases to be an integer of 1, 2, 3, ... as seen in the classic chi-square distribution Pearson. The index number of degrees of freedom for the dependent biometric data is fractional (fractal). This fact has to be taken into account when assessing the likelihood of errors of the first and second kinds of aut.
Pages: 3-10
References
- Elfimov A.V., Vojachek S.A., Kachajjkin E.I., Kulikov S.V. Obuchenie nejjrosetevogo identifikatora avtorstva rukopisnykh tekstov // Nejjrokompjutery: razrabotka, primenenie. 2009. № 6. S. 17-21.
- Seregin V.V. Pocherkovedenie i pocherkovedcheskaja ehkspertiza / Pod red. V.V. Seregina. Volgograd: Izd-vo VA MVD Rossii. 2007. 340 s.
- Ishhenko E.P., Toporkov A.A. Kriminalistika. M.: Kontrakt. 2006. 748 s..
- Sreda modelirovanija «BioNejjroAvtograf». http://pniehi.rf/activity/science/noc.htm.
- Patent № 2390843 (RU). Sposob raspoznavanija znakov / A.I. Ivanov, D.JU. Andreev, S.A. Vojachek, A.V. Elfimov.
- Galushkin A.I., Cypkin JA.Z. Nejjronnye seti: istorija razvitija. M.: Radiotekhnika. 2001. 840 s.
- KHajjkin S. Nejjronnye seti: polnyjj kurs. M.: Viljams. 2006. 1104 s.
- Akhmetov B.S., Volchikhin V.I., Ivanov A.I., Malygin A.JU. Algoritmy testirovanija biometriko-nejjrosetevykh mekhanizmov zashhity informacii. Kazakhstan, Almaty: Izd-vo KazNTU im. K.I. Satpaeva. 2013. 152 s. http://portal.kazntu.kz/files/publicate/2014-01-04-11940.pdf.
- Akhmetov B.S., Nadeev D.N., Funtikov V.A., Ivanov A.I., Malygin A.JU. Ocenka riskov vysokonadezhnojj biometrii. Monografija. Almaty: Izd-vo KazNTU im. K.I. Satpaeva. 2014. 108 s.
- SHalygin A.S., Palagin JU.I. Prikladnye metody statisticheskogo modelirovanija. L.: Mashinostroenie. 1986. 320 s.
- Bezjaev A.V., Ivanov A.I., Funtikova JU.V. Optimizacija struktury samokorrektirujushhegosja bio-koda, khranjashhego sindromy oshibok v vide fragmentov khesh-funkcijj // Vestnik Uralskogo federalnogo okruga. Bezopasnost v informacionnojj sfere. 2014. № 3(13). S. 4-14.
- EHjjkkhoff P. Osnovy identifikacii sistem upravlenija. M.: Mir. 1975. 517 s.
- Ivanov A.I. Simple Numerical Method of SeparabelVolterra Kernels Symmetrization // Engineering Simulation. 1999. V. 16. R. 411-416.
- Volchikhin V.I., Ivanov A.I., Funtikov V.A., Malygina E.A. Perspektivy ispolzovanija iskusstvennykh nejjronnykh setejj s mnogourovnevymi kvantovateljami v tekhnologii biometriko-nejjrosetevojj autentifikacii // Izvestija vysshikh uchebnykh zavedenijj. Povolzhskijj region. Tekhnicheskie nauki. 2013. №4(28). S. 88-99.
- Ivanov A.I., Malygina E.A. Biometricheskaja autentifikacija lichnosti: obrashhenie matric nejjrosetevykh funkcionalov v prostranstve metriki KHemminga // Voprosy zashhity informacii. 2015. № 1. S. 23-29.