350 rub
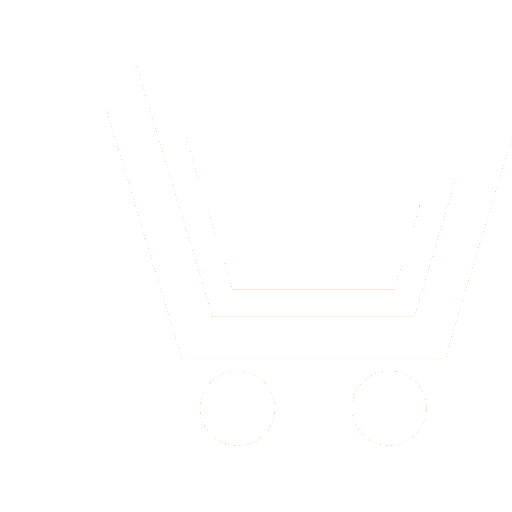
Journal Nonlinear World №3 for 2016 г.
Article in number:
Probabilistic parametric identification signals
Authors:
V.N. Zhurakovsky - Ph.D. (Eng.), Associate Professor, SM-6 Department, Bauman Moscow State Technical University. E-mail: zhurakovsky@sm.bmstu.ru
V.R. Kuznetsov - Engineer, SM2-2 Department, Bauman Moscow State Technical University. E-mail: wator1@mail.ru
S.I. Silin - Ph.D. (Eng.), Associate Professor, SM-5 Department, Bauman Moscow State Technical University. E-mail: sm2-2@inbox.ru
Abstract:
Modern signal processing is the extraction of certain information (parameters) of the signal for further use. In particular, the signal parameters can recognize its appearance, with a priori known types of signals. Existing approaches of designing signal recognition algorithms do not allow to fully ensure the correct processing of these parameters, as it does not take into account some important factors. These factors are associated primarily with the noise that does not allow you to uniquely identify useful signals in a noisy environment.
This problem was solved by the methods of parametric probability of selection, which are widely used in statistics, acoustics and radar. The method used is to combine the signals having similar characteristics parametric allocated from the received signals. Another problem that arises in the process step is to determine the ambiguity parameter selected by selection signals, extraction necessitates more probable signal form. Extraction most veroyatnogovida signal transmitted by the parameters is achieved by a formula derived by using the apparatus of probability theory and mathematical statistics for the a posteriori probability.
Thus, the developed approach to synthesizing signal recognition algorithm allows for «soft» signal recognition algorithm. The developed algorithm has been investigated in deciding whether a signal is present when applying a complex mixture of the specified signal and a set of interfering signals.
Pages: 65-70
References
- Tsihrintzis G.A., Tsakalides P., Nikias C.L. Signal Detection in Severely Heavy-Tailed Radar Clutter // Asilomar Conference on Signals, Systems and Computers, Asilomar Conference on Signals, Systems and Computers. 1995.R. 865.DOI:10.1109/ACSSC.1995.540823.
- Junyang Pan, Jinyan Du, Shie Yang Weak Signal Detection Based on Chaotic Prediction // International Workshop on Knowledge Discovery and Data Mining (WKDD, 2009). International Workshop on Knowledge Discovery and Data Mining. 2009. R. 300-303.DOI:10.1109/WKDD.2009.107.
- ZHurakovskijj V.N., Kondrashov K.S. Algoritm razdelenija podvizhnykh i malopodvizhnykh obektov v malogabaritnojj obzornojj RLS // Spectekhnika i svjaz. 2015. №2. C. 53-59.
- Pirogov A.A., Silin S.I., ZHurakovskijj V.N., Logvinenko A.S. Analiz ehffektivnosti cifrovojj realizacii izmerenija parametrov signalov // Radiooptika. 2015. №5. DOI: 10.7463/rdopt.0515.0812966.
- Jian-fei Liu, Jia-yu Liu, Ting-ting Zhang, Jing-chun Li Application of Cross-Correlation Algorithm in Radio Weak Signal Detection (CNSR, 2009) // Communication Networks and Services Research, Annual Conference on Communication Networks and Services Research, Annual. Sonference on 2009. R. 440-442.DOI:10.1109/CNSR.2009.78.
- Ghulam Rasool, Patrick Mader Flexible design pattern detection based on feature types (ASE, 2011) // 26th IEEE/ACM International Conference on Automated Software Engineering (ASE 2011). 2011 26th IEEE/ACM International Conference on Automated Software Engineering (ASE 2011). 2011. R. 243-252.DOI:10.1109/ASE.2011.6100060.
- Garbe M., Bunnig Ch., Gutschmidt A., Cap C. Moving Type Detection without Time Information (ICSC, 2012) // IEEE Sixth International Conference on Semantic Computing. 2012 IEEE Sixth International Conference on Semantic Computing 2012. R. 318-324. DOI:10.1109/ICSC.2012.40.
- Pustylnik E.I. Statisticheskie metody analiza i obrabotki nabljudenijj. M.: Nauka. 1968.
- Zamir R., Rose K. Natural type selection in addaptive lossy compression // IEEE Trans. Information Theory.2001.V. 47. R. 99-111.
- Zamir R., Rose K. Adaptive Parametric Vector Quantization by Natural Type Selection (DCC, 2002) // Data Compression Conference Proceedings. 2002. R. 392-401. DOI: 1109/DCC.2002.999979.
- Koichi Ichige, Mitsuharu Imai, Hiroyuki Arai Fastica-Based Blind Signal Separation and its Application to Radio Surveillance (SSP, 2007) // Statistical Signal Processing. IEEE/SP Workshop on, Statistical Signal Processing. IEEE/SP Workshop on 2007. R. 546-550.DOI:10.1109/SSP.2007.4301318.
- Gmurman V.E. Teorija verojatnostejj i matematicheskaja statistika. M.: Vysshee obrazovanie. 2005.
- Borzov A.B., Suchkov V.B., SHakhtarin B.I., Sidorkina JU.A. Matematicheskoe i imitacionnoe modelirovanie vkhodnykh signalov sistem blizhnejj radiolokacii // Radiotekhnika i ehlektronika. 2014. T. 59. № 12. S. 1195-1208.