350 rub
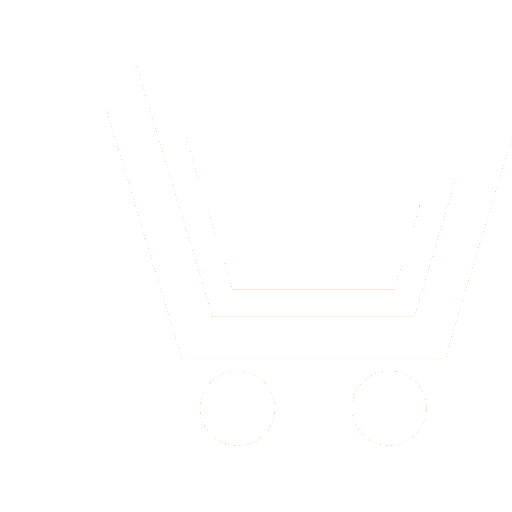
Journal Nonlinear World №1 for 2015 г.
Article in number:
Neural network modelling of heat exchange chlorobenzene in electric field
Authors:
A.R. Mukhutdinov - Dr.Sc.(Eng.), Professor, Department «Technology solid chemical substances», Kazan State Technological University E-mail: muhutdinov@rambler.ru M.G. Efimov - Bachelor, Department «Technology solid chemical substances», Kazan State Technological University. E-mail: jero07@bk.ru
Abstract:
Effective way of an intensification of heat exchange processes is use of electric fields. This method of an intensification of heat exchange is applied when traditional ways are inefficient and can\'t provide desirable result. The intensification of heat exchange by direct imposing of electric fields leads, other things being equal, to possibility of reduction of existing surfaces of heat exchange, and, therefore, dimensions and the mass of the heat exchange equipment. For achievement comparative on an absolute value of effects of an intensification of a heat in case of imposing of electric fields smaller expenses of electric power are required much more, than when using traditional ways. Object of research are the problem connected with direct impact of electric fields on chlorobenzene and processes occurring in it. Constants, variable low-frequency (50 Hz) and high-frequency (from 3 to 6∙105 Hz) electric fields have impact on heat exchange processes in chlorobenzene. It should be noted that forecasting of effects of influence of electric fields for heat exchange in chlorobenzene has great applied value.
The purpose of this work is development of neural network model and its application for detection of new dependences of influence of electric fields on coefficient of heat conductivity of chlorobenzene.
Object of research is relative change of coefficient of heat conductivity of chlorobenzene in electric field. The choice of liquid was carried out on value of its conductivity. By authors of work [7] it is established that influence of various electric fields leads to increase of coefficients of heat conductivity and dynamic viscosity of dielectric liquids more than twice and to reduction of coefficient of mutual diffusion three times.
At the solution of a task direct controlled training \"with the teacher\" INS on the basis of algorithm of the return distribution of a mistake is used. This algorithm is considered now one of the most effective algorithms of training of INS. It defines strategy of selection of scales of a multilayered network with application of gradient methods of optimization. Its basis is made by the criterion function formed, as a rule, in the form of the square sum of a difference between the actual and expected values of output signals. For finding of optimum values of weight coefficients the method modified ParTan is used. It is the improved modification of a gradient method (a method of the fastest descent) function minimization. During work got out optimum structure of INS (the total of the hidden layers - 2 and quantity of neurons in layers respectively is defined: 90; 50) activation function (sigmoidny function) and an optimization method (modified ParTan). According to number of input and output parameters, quantity of neurons in the first layer - 10, in the last - 1 (figure 2). Total number of steps of training by a method modified ParTan made 6210 steps for selection of 16 experiments.
Pages: 59-64
References
- Mukhutdinov A.R., Lyubimov P.E. Application of a neural network model for revealing specific features and regularities of solid fuel burning process // Thermal Engineering (Englich translation of Teploenergetika). 2010. V. 57 (4). P.336.
- Mukhutdinov A.R.,Okulin M.V. Development of a neural network programming module for predicting the strength properties of solid fuel // Chemical and Petroleum Engineering. 2011. V. 47 (3). P. 266.
- Mukhutdinov A.R., Vakhidova Z.R., KHakimova M.A.Nejjrosetevoe modelirovanie teploobmena v benzole pri chastote 600 kGc// Vestnik Kazan. tekhnol. un-ta. 2013. T.18. № 3. S. 96 - 99.
- Mukhutdinov A.R., Vakhidova Z.R., KHakimova M.A.Kompjuternoe modelirovanie teploobmena v benzole // Vychislitelnye tekhnologii. 2014. T.19. №2. S.107 - 113.
- Mukhutdinov A.R. Nejjrosetevoe prognozirovanie i upravlenie ehkspluatacionnymi parametrami processa gorenija topliva na teplovykh ehlektricheskikh stancijakh // Izvestija vysshikh uchebnykh zavedenijj. Problemy ehnergetiki. 2006. №7 - 8. S. 84 - 89.
- Mukhutdinov A.R., Marchenko G.N., Vakhidova Z.R. Nejjrosetevoe modelirovanie i optimizacija slozhnykh processov i naukoemkogo teploehnergeticheskogo oborudovanija // Kazan: Kazan. gos. ehnerg. un-t, 2011. 296 s.
- Savinykh B.V., Gumerov F.M. Svojjstva perenosa diehlektricheskikh zhidkostejj i teplo-massoobmen v ehlektricheskikh poljakh. Kazan: Fehn. 2002. 384 s.
- KHajjkinS. Nejjronnye seti: polnyjj kurs. Izd-e 2-e: Per. s angl. M.: Izdatelskijj dom «Viljams». 2006.