350 rub
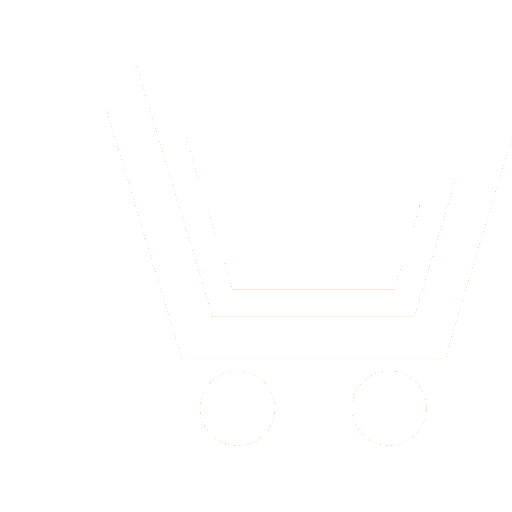
Journal Nonlinear World №10 for 2013 г.
Article in number:
On the statistical analysis of data from hybridization DNA microarray in the study of radiation-induced dynamics of the transcriptome of cancer cells and K562 Me45
Authors:
R.T. Sibatov - Dr. Sc. (Phys.-Math.), Engineering and Physical Department of High Technologies, Ulyanovsk State University. E-mail: ren_sib@bk.ru
J.V. Saenko - Ph. D. (Biol.), Senior Research Scientist, Research Institute of Technology, Ulyanovsk State University. E-mail: saenkoyv@yandex.ru
E.S. Glushhenko - Junior Research Scientist, Research Institute of Technology, Ulyanovsk State University. E-mail: JaneG@yandex.ru
A.N. Byzykchi - Master Student, Engineering and physical dept. of high technologies, Ulyanovsk State University. E-mail: azy.baza@gmail.com
J.V. Saenko - Ph. D. (Biol.), Senior Research Scientist, Research Institute of Technology, Ulyanovsk State University. E-mail: saenkoyv@yandex.ru
E.S. Glushhenko - Junior Research Scientist, Research Institute of Technology, Ulyanovsk State University. E-mail: JaneG@yandex.ru
A.N. Byzykchi - Master Student, Engineering and physical dept. of high technologies, Ulyanovsk State University. E-mail: azy.baza@gmail.com
Abstract:
The aim of the study was to analyze the data of radiation-induced dynamics of gene expression obtained by hybridizing the DNA chip of high density. We used data from experiments of the study of gene expression in K562 and Me45 cells, irradiated at a dose of 4 Gy. Transcriptome was analyzed in 1, 12 and 24 hours after irradiation using the Affymetrix microarray HGU133A series. It was demonstrated that the expression of the probability characteristics of differences of an expression significantly depend on intensity of a basic level. Procedure of a filtration of the genes, based on the model «bending around noise» was offered and carried out. This procedure significantly improved the results of a clustering by a method of k-averages or k-medians. The successful clustering is meant as an association of replicated measurements in one cluster. The best of methods of a clustering is the method of k-medians in comparison with a method of k-averages, and from similarity metrics are cosine distance (uncentered correlation) and coefficient of correlation of Pearson. For the genes which have been filtered, by methods of a hierarchical clustering dendrograms were constructed. On the basis of these dendrograms the comparative studying of dynamics of change of an expression of genes of key alarm ways connected with programmable cellular death and DNA reparation in cancer cells with a normal and mutant gene of TP53 is planned.
Pages: 739-746
References
- Wei Keat Lim, Kai Wang, Celine Lefebvre, Andrea Califano. Comparative analysis of microarray normalization procedures: effects on reverse engineering gene networks // Bioinformatics. 2007. V. 23 ISMB/ECCB. P. i282-i288.
- Oficzial'ny'j sajt kompanii Affimetrics // Affimetrics Inc. Statistical algorithms description document. 2013. URL: http://www.affymetrics.com/ support/technical/whitepapers.affx.
- Bolstad B.M., Irizarry R.A., Astrand M., Speed T.P. A comparison of normalization methods for high density oligonucleotidearray data based on variance and bias // Bioinformatics. 2003. V. 19. № 2. R. 185-193.
- Irizarry R.A., Bolstad B., Collin F., Cope L., Hobbs B., Speed T. Summaries of Affymetrix Gene Chip probe level data. 2003. Nucleic Acids Research V. 31. № 4.
- Gentleman R.C., Carey V.J., Bates D.M., Bolstad B., Dettling M., Dudoit S., Ellis B., Gautier L., Ge Y., Gentry J., Hornik K., Hothorn T., Huber W., Iacus S., Irizarry R., Leisch F., Li C., Maechler M., Rossini A.J., Sawitzki G., Smith C., Smyth G., Tierney L., Yang J.Y., Zhang J. Bioconductor: Open software development for computational biology and bioinformatics // Genome Biology. 2004. V. 5. № 10. P. R80:1-R80:16.
- Gautier L. Affy-analysis of Affymetrix GeneChip data at the probe level // Bioinformatics. 2004. V. 20. № 3. P. 307-315.
- Smith C.A. Browser-based Affymetrix Analysis and Annotation // In: Bioinformatics and Computational Biology Solutions Using R and Bioconductor. New York: Springer. 2005. P. 313-326.
- Smyth G.K. Limma: linear models for microarray data // In: Bioinformatics and Computational Biology Solutions using R and Bioconductor. New York: Springer. 2005. P. 397-420.
- Gentleman R., Carey V., Huber W., Hahne F. Genefilter: methods for filtering genes from microarray experiments. Version 1.42.0. 2013. URL: http://www.bioconductor.org/ packages/2.12/bioc/manuals/genefilter/man/genefilter.pdf
- Irizarry R.A., Bolstad B., Collin F., Cope L., Hobbs B., Speed T. Summaries of Affymetrix Gene Chip probe level data // Nucleic Acids Research. 2003. V. 31. № 4. P. e15.
- Millenaar F.F., Okyere J., May S.T., van Zanten M., Voesenek L. A. C. J. and Peeters A. J. M. How to decide - Different methods of calculating gene expression from short oligonucleotide array data will give different results // BMC Bioinformatics. 2006. № 7. P. 137.
- Methods in Microarray Normalization / ed. by Phillip Stafford. Taylor & Francis Group. LLC. 2008.
- Pepper S.D., Saunders E.K., Edwards L.E., Wilson C.L., Miller C.J. // BMC Bioinformatics. 2007. № 8. P. 273.
- Baldi P., Long A.D. A Bayesian framework for the analysis of microarray expression data: regularized t-test and statistical inferences of gene changes // Bioinformatics. 2001. V. 17. № 6. P. 509-519.
- Miller Jr.R.G., Brown B.W. Beyond ANOVA: basics of applied statistics. Chapman & Hall/CRC. 1997.
- Girden E.R. ANOVA: Repeated measures. SAGE Publications. Incorporated. 1992.