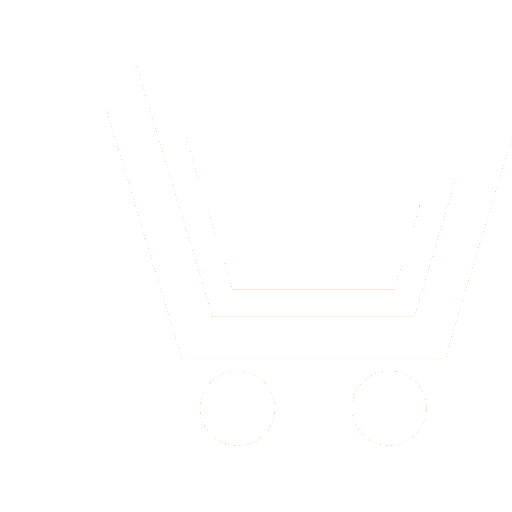
V.V. Dobriyan1
1 Saratov State Technical University named after Yu.A. Gagarin (Saratov, Russia)
Problem setting. The development and application of methods for calculating Lyapunov exponents is an urgent task in the analysis of nonlinear dynamics of systems of various kinds. A promising direction in solving this problem is the use of neural networks.
Target. To propose an algorithm and a method for calculating the spectrum of Lyapunov exponents based on a sample from a single coordinate using neural networks.
Results. A modification of the neural network method for calculating the spectrum of Lyapunov exponents based on a sample from a single coordinate is proposed. Various methods of calculating Lyapunov exponents (the Benettin method, the Wolf method, the Rosenstein method, the Kantz method, the synchronization method, the Sano-Sawada method and the method proposed in the paper) for such classical problems of nonlinear dynamics as: Henon map, generalized Henon map, Lorenz attractor are analyzed. The method proposed in the paper turned out to be the most effective in terms of sample size and accuracy compared to other methods presented. It is shown that the method gives good computational results for various types of systems with a small sample size from one coordinate, without requiring the presence of the initial equations of the system.
Practical significance. The results obtained can be used in the analysis of nonlinear dynamics of distributed systems of various kinds - both mechanical (beams, shells, plates) and non–mechanical (historical, financial, demographic data, biomedical signals).
Dobriyan V.V. Neural network method for Lyapunov exponents spectrum calculation in analysis of classic systems nonlinear dynamics. Neurocomputers. 2023. V. 25. № 2. Р. 30-40. DOI: https://doi.org/10.18127/j19998554-202302-03 (In Russian)
- Devaney R.L. An introduction to chaotic dynamical systems, 3rd ed. New York: Chapman and Hall/CRC. 2021. 432 p. DOI: doi.org/10.1201/9780429280801.
- Banks J., Brooks J., Cairns G., Davis G., Stacey P. On Devaney’s Definition of Chaos. American Mathematical Monthly. 1992. V. 99. № 4. P. 332–334. DOI: doi.org/10.2307/2324899.
- Knudsen C. Chaos without Periodicity. American Mathematical Monthly. 1994. V. 101. P. 563–565.
- Gulick D. Encounters with Chaos and Fractals. 2nd ed. CRC Press. 2012. 387 p.
- Benettin G., Galgani L., Strelcyn J.M. Kolmogorov entropy and numerical experiments. Physical Review. 1976. V. 14. № 6. P. 2338–2345. DOI: 10.1103/PhysRevA.14.2338.
- Oseledets V.I. Multiplicative ergodic theorem. Lyapunov's characteristic indicators of dynamical systems. Proceedings of the Moscow Mathematical Society. 1968. V. 19. P. 179–210. (In Russian).
- Pesen Ya.B. Lyapunov characteristic indicators and ergodic properties of smooth dynamical systems with invariant measure. Reports of the USSR Academy of Sciences. 1976. V. 226. № 4. P. 774–777. (In Russian).
- Rosenstein M.T., Collins J.J., De Luca C.J. A practical method for calculating largest Lyapunov exponents from small data sets. Physica D. 1993. V. 65. P. 117–134.
- Kantz H. A robust method to estimate the maximal Lyapunov exponent of a time series. Physics Letters A. 1994. V. 185. № 1. P. 77–87. DOI: 10.1016/0375-9601(94)90991-1.
- Golovko V.A. Neural network methods of processing chaotic processes. VII All-Russian Scientific and Technical conference "Neuroinformatics 2005": Lectures on neuroinformatics. Moscow: MIEP, 2005. P. 43–88. (In Russian).
- Stefanski A. Estimation of the largest Lyapunov exponent in systems with impacts. Chaos, Solitons & Fractals. 2000. V. 11. № 15.
P. 2443–2451. DOI: 10.1016/S0960-0779(00)00029-1. - Stefanski A., Kapitaniak T. Estimation of the dominant Lyapunov exponent of non-smooth systems on the basis of maps synchronization. Chaos, Solitons & Fractals. 2003. V. 15. №. 2. P. 233–244. DOI: 10.1016/S0960-0779(02)00095-4.
- Sato S., Sano M., Sawada Y. Practical Methods of Measuring the Generalized Dimension and the Largest Lyapunov Exponent in High Dimensional Chaotic Systems. Progress of Theoretical Physics. 1987. V. 77. № 1. P. 1–5.
- Eckmann J.P., Ruelle D. Ergodic theory of chaos and strange attractors. Reviews of Modern Physics. 1985. V.57. № 3. P. 617–656. DOI: 10.1103/RevModPhys.57.617.
- Awrejcewicz J., Krysko A.V., Dobriyan V., Papkova I.V., Krysko V.A. On the Lyapunov exponents computation of coupled non-linear Euler-Bernoulli beams. Proceedings of the Fourteenth International Conference on Civil, Structural and Environmental Engineering Computing, Civil-Comp Press, Stirlingshire, UK. 2013. P. 53. DOI:10.4203/ccp.102.53.
- Wolf A., Swift J.B., Swinney H.L., Vastano J.A. Determining Lyapunov exponents from a time series. Physica D: Nonlinear Phenomena. 1985. V. 16. № 3. P. 285–317. DOI: 10.1016/0167-2789(85)90011-9.
- Masina O.N., Petrova N.P., Karpechenkova O.N. Application of linguistic variables and Lyapunov functions for stabilization of nonlinear fuzzy systems. Nonlinear world. 2010. V. 8. № 9. P. 590–594. (In Russian).
- Nguyen K.T., Nguyen M.K. Statistical approach to the formulation of Lyapunov stability conditions in modeling a controlled dynamic system. Information-measuring and control systems. 2017. V. 15. №. 7. P. 61–66. (In Russian).
- Henon M. A two-dimensional mapping with a strange attractor. Communications in Mathematical Physics. 1976. V. 50. № 1. P. 69–77. DOI: 10.1007/BF01608556.
- Baier G., Klein M. Maximum hyperchaos in generalized Henon maps. Physics Letters A. 1990. V. 151. №. 6-7. P. 281–284.
- Lorenz E.N. Deterministic nonperiodic flow. Journal of the Atmospheric Sciences. 1963. V. 20. № 2. P. 130–141. DOI: 10.1175/1520-0469(1963)020<0130:DNF>2.0.CO;2.
- Yaroshenko T.Y., Dobriyan V., Zhigalov M.V., Krysko V.A., Krysko D.V., Vandenabeele P., Vos H. Wavelet modeling and prediction of the stability of states: The Roman Empire and the European Union. Communications in Nonlinear Science and Numerical Simulation. 2015. V. 26. № 1-3. P. 265–275. DOI: 10.1016/j.cnsns.2015.02.013.
- Kutepov I.E., Krysko A.V., Dobriyan V.V., Yakovleva T.V., Krylova E.Yu., Krysko V.A. Visualization of EEG signal entropy in schizophrenia. Scientific Visualization. 2020. V. 12. №. 1. P. 1–9. DOI: 10.26583/sv.12.1.01.
- Awrejcewicz J., Krysko A.V., Zagniboroda N.A., Dobriyan V.V., Krysko V.A. On the general theory of chaotic dynamics of flexible curvilinear Euler-Bernoulli beams. Nonlinear Dynamics. 2015. V. 79. № 1. P. 11–29. DOI: 10.1007/s11071-014-1641-5.
- Krysko V.A., Dobriyan V., Papkova I.V., Awrejcewicz J. Size-dependent parameter cancels chaotic vibrations of flexible shallow nano-shells. Journal of Sound and Vibration. 2019. V. 446. P. 374–386. DOI: 10.1016/j.jsv.2019.01.032.
- Lozi R. Can we trust in numerical computations of chaotic solutions of dynamical systems? World Scientific Series on Nonlinear Science Series. Topology and Dynamics of Chaos. 2013. P.63–98. DOI: 10.1142/9789814434867_0004.