350 rub
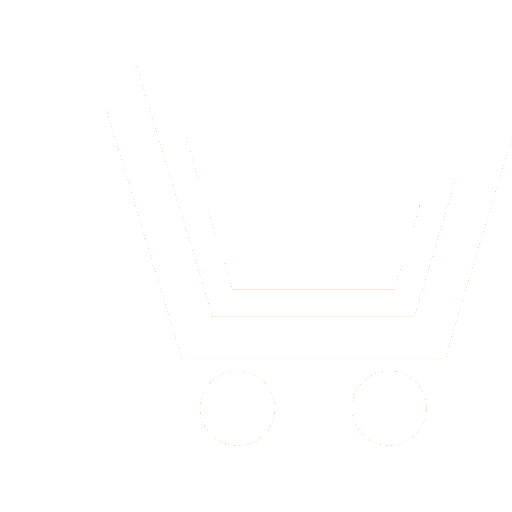
Journal Neurocomputers №8 for 2015 г.
Article in number:
Neurocomputing between sciences and pseudoscience
Keywords:
Neural Networks
Neuroscience
Neurocomputing Methodology
Principles of Demarcation
Artificial Intelligence
Data Analysis
Logistic Regression
Discriminant Analysis
Human Resources Management
Astrology
Authors:
Yu.Yu. Petrunin - Ph.D., Dr.Sc. (Philos.), Professor, School of Public Administration, Lomonosov Moscow State University, Co-Chairman of the Organizing Committee, the leading symposium Neyrophilosophy\". E-mail: petrunin@spa.msu.ru
Abstract:
Fashion on neurocomputing plays both a positive role, contributing to attract new curious minds to advanced researches, and also a negative one, by attracting adventurers looking for quick results and not less rapid popularity as well, who are not squeamish about any methods of achieving their goals and discrediting the neuroscience in general. In some publications neural network methods and models are exhibited as a panacea, called to solve all the challenges facing humanity. In this regard, there is growth not only of the interest in neurocomputing, but also growth of doubt among colleagues, who cognize the world around them, in being scientific. To divide science and pseudoscience in neurocomputing, this article proposes methodological principles of applied neurocomputing: the principle of authenticity and verifiability of methods, the principle of epistemological evolutionism, the principle of systematic knowledge and the principle of determinism. The article analyzes the consequences of violating these principles in the application of neurocomputing in Human Resources Management. Using computer models based on neural networks to meet challenges of different application areas (neurocomputing), including poorly formalized - for example social and humanitarian, is an important area of science. Of course, the application of neurocomputing in HR Management may be welcomed. This kind of research can be both a serious theoretical value, which opens new, often unexpected dependence in this domain, and practical, helping to improve the quality of actual governance. This work is pioneering in its nature and therefore deserves special attention. The authors set themselves the task to identify the factors affecting on the efficiency of manager, or rather on \"a person\'s ability to perform work of leadership\". This study examined not only the traditional features, which can affect this efficiency (gender, age, marital status, etc..), but also unusual for classical science astrological characteristics of the person (zodiac signs, elements and other natal characteristics). At the same research methods are accurate, verifiable, based on empirical data, i.e. they are quite relevant to scientific criteria. Despite its relevance, prospectivity, logical clarity and originality of used methodology in the work, there are some serious flaws, to a summary of which we turn now.
First of all, despite the wide erudition and the authors absolute priority in many areas neurocomputing, this work shows a very common, unfortunately, in the native scientific literature attribute to ignore publications of colleagues on the same theme. As it is known, any scientific article begins with a review of the literature. Apparently, the authors believe that outside of a respected Perm scientific school to which they belong, there is nothing worthy. Even publications, issued in generalizing work \"neurocomputing paradigm and society\", the authors of one of the sections which are, by the way, the authors of reviewed article, are not even mentioned.
Even worse is the case with the literature in the field of HR management. With all due respect to the work of John Collins\' \"Good to Great\", there are other approaches to the understanding of which competencies are the most important for the manager - professional or basic. Inattention to the ad hoc literature immediately leads to the substitution of the term for what is actually studied.
The second observation relates to the obtained results. Its checkup in widespread and quite affordable programs NeuroShell Predictor and Statistica has shown the following. Indeed, if it is used as predictors separately just the \"traditional\" variables (gender, age, etc.), or just the \"astrological\" variables (zodiac sign, elements, etc.), the model shows worse results than if both groups of predictors are used together. The standard error of the trained neural network for traditional variables equals to 8%; for astrological 12.5%; at the sharing of all the variables, it decreases to 6%. However, the application of the trained neural network on test data results in such errors that it is hard to say the forecasts, because the square deviation reaches 60 % and even more. Given in the article low indicators of the errors magnitude are related to the trained neural networks. When they start to be applied to the test data, the results are disappointing.
Finally, the concluding remark. Neural networks - not a panacea, not a magic stick which opens the last truths that are inaccessible to other methods of knowledge. The classic method of solving article\'s problem is the method of logistic regression. As most predictors are described by low - or nominal informative variables (gender, sphere of activity, zodiac sign, the element) or ordinals variables (the number of children in the family), it is true that the quality of the regression can not be good (especially for a small number of observations), and the use of neural networks seems promising. However, it is quiet possible to analyze the connection between individual pairs or even groups of variables (several independent and one dependent), and then to compare them with the results obtained by using the neural network. In other words, neural networks should be built over the conventional methods of data analysis, refining or developing (but not replacing) them.
Of course, since the independent variables have different types, it is necessary to use various methods. For example, to determine the connection between the nominal dependent variable (business status of the respondent) and the number of children and / or age (interval or ordinal variables) application of Student test (or Mann-Whitney test when the conditions of Student\'s test are not applicable) shows that there is a firmly established statistical dependence between these variables.
This work was financially supported by RFH in grant № 15-03-00519a - Post-non-classic paradigm of artificial intellect?.
Pages: 52-64
References
- Hess E., Jokeit H. Neurocapitalism // Merkur. 2009. № 6. P. 1-8.
- Nejjrokompjuternaja paradigma i obshhestvo / Pod red. JU.JU. Petrunina. M.: MGU. 2012.
- Chechkin A.V., Alexeev A.Yu., Petrunin Yu.Yu., Savelyev A.V., Yankovskaya E.A. Neurophilosophy // Nejjrokompjutery: razrabotka, primenenie. 2014. № 10. S. 3-13.
- SHiller R. EHkonomika mozga // Vedomosti.ru. 23.11.2011.
- JAsnickijj L.N., Mikhaleva JU.A., CHerepanov F.M. Vozmozhnosti metodov iskusstvennogo intellekta dlja vyjavlenija i ispolzovanija novykh znanijj na primere zadachi upravlenija personalom // ZHurnal formirujushhikhsja napravlenijj nauki. 2014. № 6 (2). C. 32-41.
- Tjurina I.O. Kadrovyjj menedzhment: process otbora personala // Sociologicheskie issledovanija. 2000. № 4. S. 22-32.
- Dorrer M.G. Intuitivnoe predskazanie nejjrosetjami vzaimootnoshenijj v gruppe // Metody nejjroinformatiki / Pod red. A.N. Gorbanja. Krasnojarsk: KGTU. 1998. 205 s.
- Dorrer M.G. Psikhologicheskaja intuicija iskusstvennykh nejjronnykh setejj: Diss. - kand. tekhn. nauk. Krasnojarsk: STGU. 1998. 124 s.
- Alekseev A.JU., Kuznecov V.G., Petrunin JU.JU., Savelev A.V. Nejjrofilosofija kak konceptualnaja osnova nejjrokompjutinga // Nejjrokompjutery: razrabotka, primenenie. 2015. № 5. S. 69-77.
- CHerepanov F.M. Simuljator nejjronnykh setejj dlja vuzov // Vestnik Permskogo universiteta. Matematika. Mekhanika. Informatika. 2012. № 3. S. 98-105.
- CHerepanov F.M., JAsnickijj L.N. Issledovatelskijj simuljator nejjronnykh setejj // Iskusstvennyjj intellekt: filosofija, metodologija, innovacii: Materialy Pjatojj Vserossijjskojj konferencii. Moskva, MGTU MIREHA. 9-11 nojabrja 2011 g. M.: Radio i svjaz. 2011. S. 137-139.
- CHerepanov F.M., JAsnickijj L.N. Issledovatelskijj simuljator nejjronnykh setejj // X vserossijjskaja nauchnaja konferencija «Nejjrokompjutery i ikh primenenie»: tezisy dokladov. M.: MGPPU. 2012. S. 31.
- CHerepanov F.M., JAsnickijj L.N. Svidetelstvo ob otraslevojj registracii razrabotki № 8756. Simuljator nejjronnykh setejj «Nejjrosimuljator 1.0». Zaregistrirovano v Otraslevom fonde algoritmov i programm 12.07.2007.
- JAsnickijj L.N., CHerepanov F.M. Iskusstvennyjj intellekt dlja polittekhnologov // http://www.permai.ru/files/projects/P05.pdf
- Bongard M.M. Problema uznavanija. M., 1967.
- DjukV., SamojjlenkoA. Data Mining. Uchebnyjj kurs. SPb. 2001. C. 184-244.
- KHekht-Nilsen R. Nejjrokompjuting: istorija, sostojanie, perspektivy // Otkrytye sistemy. SUBD. 1998. № 4. S. 10-14.
- Petrunin JU.JU. Nasledie I.M. Sechenova i sovremennyjj prikladnojj nejjrokompjuting // Biomedicinskaja radioehlektronika. 2015. № 4. S. 56-57.
- Alekseev A.JU., Kuznecov V.G., Petrunin JU.JU., Savelev A.V., JAnkovskaja E.A. Aktualnye voprosy nejjrofilosofii // Nejjrokompjutery: razrabotka, primenenie. 2015. № 4. S. 9-11.
- Petrunin JU.JU.Nejjrofilosofija v sisteme nejjronauk // Nejjrokompjutery: razrabotka, primenenie. 2015. № 4. S. 63-64.
- Brjancev I.S., Savelev A. V. V zashhitu nejjrokompjutinga // Nejjrokompjutery: razrabotka, primenenie. 2015. № 4. S. 24-25.