350 rub
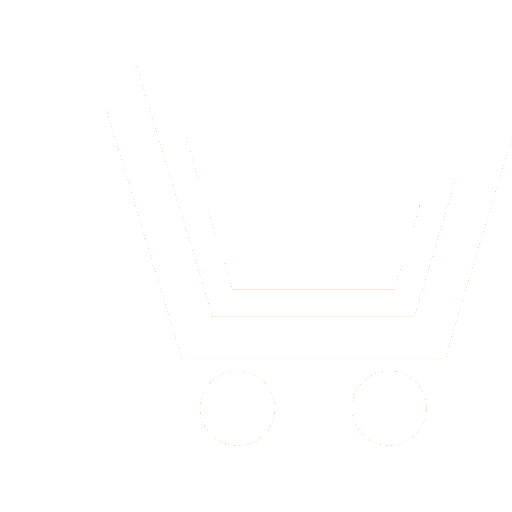
Journal Neurocomputers №2 for 2015 г.
Article in number:
Neural network modeling of the stress-deformation behavior of viscoelastic media
Keywords:
viscoelastic medium
linear Volterra integral operator
the deformation gradient and the shear stresses
finite deformation
dynamic neural network with associative and genetic memory
neural network in the state space
learning algorithms with the teacher
Authors:
Yu.A. Basistov - Ph. D. (Phys.-Math.), Senior Research Scientist, Institute of Applied Mechanics RAS (Moscow). E-mail: yuabas@mail.ru
Yu.G. Yanovsky - Dr. Sc. (Eng.), Professor, Institute of Applied Mechanics RAS (Moscow). E-mail: iam@iam.ras.ru
Abstract:
Currently, for the synthesis of models of viscoelastic media, typically used elements of Maxwell, or Jeffreys, either Voigt-Kelvin [Joseph DD Fluid Dynamics of Viscoelastic Liquids. Spinger-Verlag New York Inc. 1990, p.755]. Maxwell element is a series connection of elastic and viscous components. Maxwell stress relaxes to zero at a constant relaxation time. Therefore, the elements of Maxwell can be used only for the simulation of linear viscoelastic liquid media. From the parallel connection of these elements have been formed a linear mathematical model [Bernstein B., Kearsley E.A., Zapas I.J., A Study of Stress Relaxation with Finite Strain. Trans. Soc. Rheol., 7, 397−410, 1963], based on the integral Fredholm operator of the first kind. Kelvin-Voigt element consists of a parallel connection of the elastic and viscous components. It stress not relaxes to zero. Therefore, the element can only be used for the study of solid viscoelastic media. The above models use linear viscoelastic elements regarding stress and strain gradient, which may correspond to only small deformations. These elements in the model environment mutually connected and the deformation is not transmitted from one neighboring to another. These models can-t be used for modeling of complex heterogeneous environments, as adapted only for liquid or solid fractions of the medium. We synthesized the nonlinear model of viscoelastic substances, not having the above drawbacks [Basistov Yu.A., Yanovsky Yu.G., Neural network with associative and hereditary memory as a model of viscoelastic media. Neurocomputers, development and application. 2010., №7, s.29−39]. Note that the proposed model generalizes the well-known Hopfield network [Haykin, S., Neural Networks (full course), Moscow, Saint Petersburg, Kiev, 2006] with associative memory to space on L_2, and also has else genetic memory that neural Hopfield network is not. However, this model can only describe relaxation as inertial process of transition from the state of loading in a state of stable thermodynamic equilibrium with the minimum potential energy. In order for this model could work with external dynamic loading, it must be supplemented by input and output. Therefore, we turn to the state-space model, in which the first equation determines the evolution of the environment temporally (model offered by us), but under the influence of an external stress. The second equation is the equation of observation. It simulates the experimental apparatus - reoviscosimeter. Thus, we identify a modified Elman neural network to the real viscoelastic medium - uncured elastomeric composite based on a matrix of natural rubber (polyisoprene), filled with 20 wt% of carbon black mark number 330, PI 330 hereinafter. Follow the procedure for training a neural network to experiment with strain gradient gamma = 1. It is shown that the system has been reached a predetermined quality of training equal to 0.001 for 11 seconds at 100 neurons in the recurrent layer by processor CORE i7. It was made testing the neural network on signals with another parameters gamma = 2, gamma = 3 and gamma = 4, which is not given to the neural network for training. However, the neural network outputs were not significantly different from the experimental signals. Last experiment shows that the neural network has the property of generalization of the training material. Note that the models based on integral and differential equations, not have this property. It was made graph of the nonlinear dependence of the amplitude of shear stresses on the amplitude of the deformation gradient, reproduced neural network. It is shown that this schedule coincides with the experimental graph. Note that this graph which reproduced of nonlinear integral model with factorization nonlinearity function deviates from a similar experimental graph. The latter experiment also confirms the advantage of neural network model to nonlinear models based on integral and differential equations.
Pages: 20-28
References
- Joseph D.D. Fluid Dynamics of Viscoelastic Liquids. Spinger-Verlag New York Inc. 1990. 755 p.
- Giesecus H. A Simple Constitutive Equation for Polymer Fluids Based on the Concept of Deformation - Dependent Tensorial Mobility // J. Non-Newtonian Fluid Mech. 1982. № 11. P. 69−109.
- Phan-Thien N., Tanner R. A New Constitutive Equation Dreived from Network Theory // J. Non-Newtonian Fluid Mech. 1977. № 2. P. 353−365.
- Basistov JU.A., JAnovskijj JU.G. Nekorrektnye zadachi v mekhanike (reologii) vjazkouprugikh sred i ikh reguljarizacija // Mekhanika kompozicionnykh materialov i konstrukcijj. 2010. T. 16. № 1. S. 117−143.
- Bernstein B., Kearsley E.A., Zapas I.J. A Study of Stress Relaxation with Finite Strain // Trans. Soc. Rheol. 1963. № 7. P. 397−410.
- Basistov JU.A. Minimaksnoe reshenie uravnenija pervogo roda // DAN SSSR. 1983. T. 268. № 5. S. 1035−1038.
- Yanovsky Yu.G.,Basistov Yu.A. and Siginer D.A. Linear Inverse Problems in Viscoelastic Continua and a Minimax Method for Fredholm Equation of the First Kind // Int. J. Engng Sci. 1996. V. 34. № 11. P. 1221−1245.
- Basistov JU.A., JAnovskijj JU.G. Razvitie metoda identifikacii integralnykh nelinejjnykh modelejj vjazkouprugikh sred na baze nelinejjnojj «demping funkcii» // Mekhanika kompozicionnykh materialov i konstrukcijj. 2012. T. 18. № 4. S. 580−595.
- Basistov JU.A., JAnovskijj JU.G. Nejjrodinamicheskaja model vjazkouprugikh sred s associativnojj pamjatju // Doklady Akademii nauk (RAN). 2010. T. 430. № 4. S. 494−497.
- Basistov JU.A., JAnovskijj JU.G. Nejjronnaja set s associativnojj i nasledstvennojj pamjatju kak model vjazkouprugikh sred // Nejjrokompjutery, razrabotka, primenenie. 2010. № 7. S. 29−39.
- KHajjkin S. Nejjronnye seti (polnyjj kurs). Moskva-Sankt-Peterburg-Kiev. 2006.
- Neural Network Toolbox (For Use with MATLAB). Version 5.