350 rub
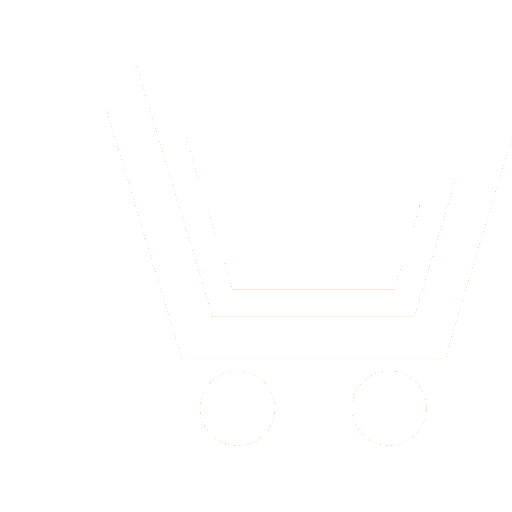
Journal Neurocomputers №10 for 2015 г.
Article in number:
Research approximation of fuzzy models
Authors:
T.S. Legotkina - Ph.D. (Eng.), Associate Professor, Department of Automation and Telemechanics, Perm National Research Polytechnic University. E-mail: luda@at.pstu.ac.ru
Y.N. Khizhnyakov - Dr.Sc. (Eng.), Professor, Associate Professor, Department of Automation and Telemechanics, Perm National Research Polytechnic University. E-mail: luda @ at.pstu.ac.ru
V.S. Nikulin - Student, Department of Automation and Telemechanics, Perm State National Research University. Е-mail: loskutov90@mail.ru
Abstract:
Specific quality systems fuzzy synthesize object model based on heuristic information received from an expert or as a result of the experiment. Today fuzzy inference methods used to approximate functions of recognition and classification of images, modeling and control of nonlinear objects, decision making under uncertainty.
The main stages of fuzzy inference rules are building a base of fuzzy inference; fuzzification of the input variables; processing the values of degrees of belonging and defuzzification of output variables.
Please note that with the number of inputs of the fuzzy model, the number of rules increases dramatically. From a practical point of view it is acceptable enough to have a good approximation accuracy. In this case, the challenge is to find a possible compromise between the specified accuracy, the number of laws and the formation of rules which allow an approximation to a given accuracy in a reasonable time.
All the methods are different approximation method for determining the output signal (deffazifikatsii method) method of processing degree of membership and the type of membership functions. Selection of specific methods determined by the need to ensure the required accuracy of approximation and conversion time.
In the present article, a comparison of different methods of presentation rules. Compare conducted for various specific systems. It has been used even build the rule base and distribution rules chebyshev law. For different cases determined by the relative error. The comparison shows that the construction of the rule base chebyshev reduces the relative approximation error, the minimal number of rules (Chebyshev), for example, n = 5 provides the same accuracy as the uniform construction rules for n = 10.
Pages: 56-61
References
- Sigeru Omatu, Marzuki KHalid, Rubija JUsof. Nejjroupravlenie i ego prilozhenija. Izd. 2-e. M.: IPRZHR. 2000. 272 s.
- Borisov V.V., Kruglov V.V., Fedulov A.S. Nechetkie modeli i seti. M.: Gorjachaja linija-Telekom. 2007. 284 s.
- Suetin P.K. Klassicheskie ortogonalnye mnogochleny. Izd. 3-e, pererab. i dop. M.: Fizmatlit. 2005. 480 s.
- Makarov E.G. Mathcad: ucheb. kurs. SPb: 2009. 384 s.