350 rub
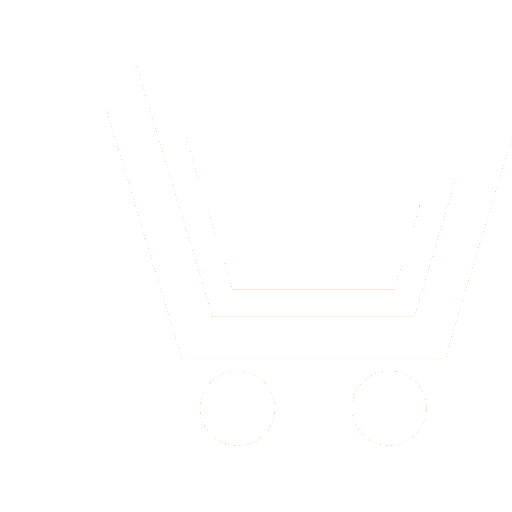
Journal Neurocomputers №10 for 2013 г.
Article in number:
The use of neural-fuzzy systems for adjustment of scales of rules for fuzzy productions on making decisions in electromyography
Authors:
N.T. Abdullayev - Ph.D. (Eng.), Azerbaijan State Oil Academy
K.Sh. Ismaylova - Assistent, Azerbaijan State Oil Academy
K.Sh. Ismaylova - Assistent, Azerbaijan State Oil Academy
Abstract:
In many cases of medical diagnosis, there arisesa problem of diagnosis for the solution of which it is impossible to obtain complete information or identification of which is notcomplete enough.
The problem of eliminating fuzziness of information is settlesby using the theory of fuzzy sets.
The popularity of the theory of fuzzy sets on designing is explained by the fact that fuzzy systems are developed faster, obtained easier and cheaper than crisp analogues.
However, on drawing up rules of fuzzy productions with given input parameters and determining of a relation with outputs, there are certain difficulties.
In ordinary artificial neural networks, there is no possibility of developing a systematic approach in generating fuzzy rules with input and output data, which is their major disadvantage. To resolve these problems Sugeno fuzzy neural network, which allows clear tracing of the output system was used.
This article considersthe possibility of determining settings rules of fuzzy productions for making decisions in electromyography by using a fuzzy neural network.
Pages: 64-69
References
- Shtovba S.D. Proektirovvanie nechetkix sistem sredstvami MATLAB. M.: Goryachaya liniya - Telekom. 2007.
- Cy'pkin Ja.Z. Osnovy' informaczionnoj teorii identifikaczii. M.: Nauka. 1984.
- Minaev Ju.N., Filimonova O.Ju., Lies B. Metody' i algoritmy' identifikaczii i prognozirovaniya v usloviyax neopredelennosti v nejrosetevom logicheskom bazise. M.: Goryachaya liniya - Telekom. 2003.
- Mityushkin Ju.I., Mokin B.I., Rotshtejn A.P. Soft-Computing: identifikacziya zakonomernostej nechetkimi bazami znanij. Vinnicza: UHIBERCUM - Binniczya. 2002.
- Rotshtejn A.P. Intellektual'ny'e texnologii identifikaczii: nechetkaya logika, geneticheskie algoritmy', nejronny'e seti. Vinnicza: UHIBERCUM - Binniczya. 1999.
- Leonenkov A.V. Nechetkoe modelirovanie v srede MATLAB i fuzzyTECH. SPb: BXV - Peterburg. 2005.
- Tagaki T., Sugeno M. Fuzzy identification of Systems and Its Applications to Modeling and Control // IEEE Trans. on Systems, Man, and Cybernetics. 1985. V. 15. № 1. R. 116-132.
- Abdullaev N.T., Ismajlova K.Sh. Oczenka informaczionnoj dostovernosti diagnosticheskix zaklyuchenij v e'lektromiografii s pomoshh'yu metoda nechetkogo logicheskogo vy'voda // Informaczionno-izmeritel'ny'e i upravlyayushhie sistemy'. 2012. T. 10. № 4. S. 60-67.
- Kasatkina L.F., Gil'vanova O.V. E'lektromiograficheskie metody' issledovaniya v diagnostike nervno-my'shechny'x zabolevanij. Igol'chataya e'lektromiografiya. M.: Medika. 2010.
- Akulov L.G. Muxa Ju.P., Naumov V.Ju. Modeli yadra bioinstrumental'ny'x izmeritel'ny'x kanalov v e'lektrofiziologii // Nejrokomp'yutery': razrabotka, primenenie. 2013. № 4. S. 39-47.