350 rub
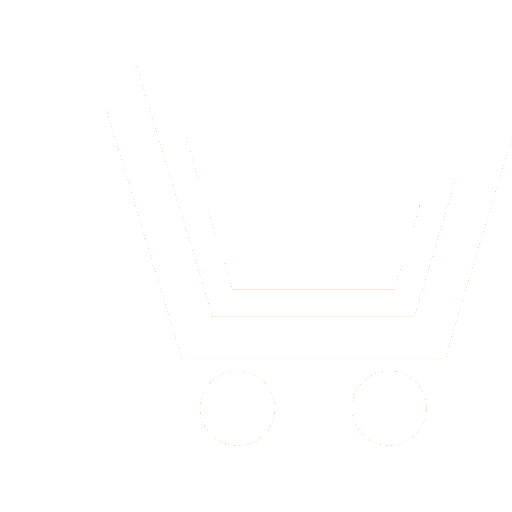
Journal Neurocomputers №8 for 2011 г.
Article in number:
Main fuzzy models classes and applying knowledge species
Authors:
A. V. Klimenko, A. V. Bobryakov, M. M. Zernov
Abstract:
Substantial part of some fuzzy model is making up by subject field knowledge. At that certain fuzzy model adaptability for making decision support (DSM) tasks in many respects depends on model using knowledge correspondence with respect to DSM task requirements.
Knowledge species at that are considered in according to classification, which bases on the next set of features:
knowledge subject;
temporal character;
character of uncertainty, which takes into consideration at knowledge description;
the way of knowledge getting.
We should mention that classification described doesn-t take into consideration direct subject field information. It leads to common knowledge character description and allows indication of different knowledge species representation by models of diverse initial appointment.
New hierarchical fuzzy model main type-s classification is offered in this article, including as universal applicable as problem-oriented models.
Models, representing linguistic values (inference and relational models), and fuzzy functional models belong to universal models.
Problem oriented models contain:
functional and relational fuzzy estimate models,
models of events (linguistic lotteries, graph models of events, models of fuzzy games),
models of states and control («situation-action» models, fuzzy situational nets, fuzzy cognitive maps, fuzzy Markov-s chains, fuzzy automates, fuzzy classification trees, fuzzy Petry-s nets).
Mane model types, belonging mentioned classes, are considered. Considered type-s short characteristics are given. Knowledge species, they operate, are defined. At that knowledge under analysis were not only knowledge, base for model-s functionality, but those that models produce.
Considered classification covers most of using fuzzy models. Detected correspondence between fuzzy models and knowledge species, they represent, allow predetermine model adequacy for DSM tasks solving. Obtained correspondence also allows elimination of model groups, adequate for single or complex DSM tasks solving.
Pages: 10-17
References
- Борисов В.В., Зернов М.М. Классификация типов концептуальных знаний, применяемых в нечетких моделях // Материалы III Всероссийской конференции студентов, аспирантов и молодых ученых «Искусственный интеллект: философия, методология, инновации». Москва: МИРЭА. 2009. С.346-349.
- Борисов В.В., Круглов В.В., Федулов А.С. Нечеткие модели и сети. М.: Горячая линия - Телеком. 2007. 284 с.
- Pedrycz W. Fuzzy Control and Fuzzy Systems. New York: John Wiley and Sons, 1993.
- Борисов А.Н., Алексеев А.В. Меркурьев Г.В. Обработка нечеткой информации в системах принятия решений. М.:
Радио и связь. 1989. 304 с. - Андрейчиков А.В. Андрейчикова О.Н. Анализ, синтез и планирование решений в экономике. М.: Финансы и статистика. 2001. 368 с.
- Дюбуа Д., Прад А. Теория возможностей. Приложения к представлению знаний и информатике: Пер. с фр. М.: Радио и связь. 1990. 288 с.
- Леоненков А.В.Нечеткое моделирование в среде MATLAB и fuzzyTECH. СПб.: БХВ-Петербург. 2003.736 с.
- Мелихов А.Н., Берштейн Л.С., Коровин С.Я. Ситуационные советующие системы с нечеткой логикой. М.: Наука. 1990. 272 с.
- Поспелов Д.А. Большие системы. Ситуационное управление. М.: Знание. 1975. 64 с.
- Силов Б.В. Принятие стратегических решений в нечеткой обстановке. М.: ИНПРО-РЕС. 1995. 228 с.