350 rub
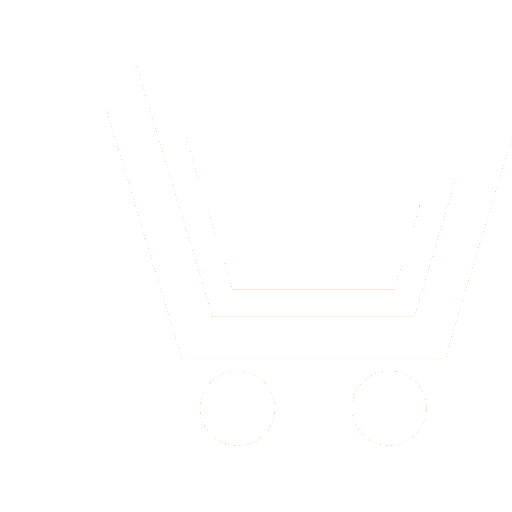
Journal Neurocomputers №9 for 2010 г.
Article in number:
Models of direct distribution computing artificial networks realizing tensor operations dimensional linear spaces over a complex field
Authors:
A.N. Macokha, T.E. Tyshler
Abstract:
Value of the device tensor operations, in particular is conclusive. Tensor operation, in various branches of physics, theoretical mechanics, differential geometry, electrodynamics. Theories of relativity. There are mathematical problems inside of most tensor algebras in which the special theory algebraic invariant linear transformations to various vector spaces and unitary modules above communicative-associative rings with unit and numerical fields of the characteristic zero is developed.
Computing volumes tensor operation in multivariate spaces are so great, that acceleration of performance tensor operations demands new approaches, in particular, parallel these operations by means of artificial networks. Modeling tensor operations with complex coordinates are used formed one-, two-and three-layer neural networks of direct distribution with linear and square-law functions of activation neural networks. The neural network is used as the mechanism of performance of parallel calculations. We shall consider modeling on a neural network of operation of addition of two trivalent tensor in three-dimensional space. For the decision the single-layered network with linear function of activation neuron as the algorithm of addition tensor is reduced to calculation of the sums of the certain inputs of a network with the weight factor equal 1 is chosen. Operations of addition, multiplication and convolution tensor As an example are lead is considered modeling on a neural network of operation of convolution trivalent tensor in measured space with two and first co variation an index on the first co variation and to the first contra variation to an index.
Models of the direct distribution computing artificial neural networks performing in coordinate-s tensor operations in finite dimensional linear spaces over a complex field are constructed. The computational program allowing obtaining data for testing the constructed models is created.
Pages: 64-70
References
- ГорбаньА. Н. Функции многих переменных и нейронные сети // Соросовский образовательный журнал. 1998. № 12. С. 105-112.
- МакохаА. Н. Выполнение операций тензорной алгебры в системе остаточных классов // Инфокоммуникационные технологии в науке, производстве и образовании: Третья международная научно-техническая конференция. Ч. III. Ставрополь: Северо-Кавказский технический университет. 2008. С. 229-235.
- МакохаА. Н., Тышляр Т. Е. Моделирование операций тензорной алгебры на базе нейронных сетей // Международная школа-семинар по геометрии и анализу памяти Н. В. Ефимова, Абрау-Дюрсо. 9-15 сентября 2008. Ростов-на-Дону: Северо-Кавказский научный центр высшей школы. 2008. С. 187-189.
- МакохаА. Н., Тышляр Т. Е. Построение нейронной сети, реализующей операции симметрирования и альтернирования тензорной алгебры // Научно-инновацион-ные достижения ФМФ в области физико-математических и технических дисциплин: Мат. 53-й научно-методической конференции преподавателей и студентов Ставропольского государственного университета «Университетская наука - региону». Ставрополь: Изд-во СГУ. 2008. С. 19-22.
- МедведевВ. С., Потемкин В. Г. Нейронные сети. MATLAB 6 / под общ. ред. В. Г. Потемкина. М.: ДИАЛОГ-МИФИ. 2002.
- РашевскийП. К. Риманова геометрия и тензорный анализ. М.: Наука. 1967.