350 rub
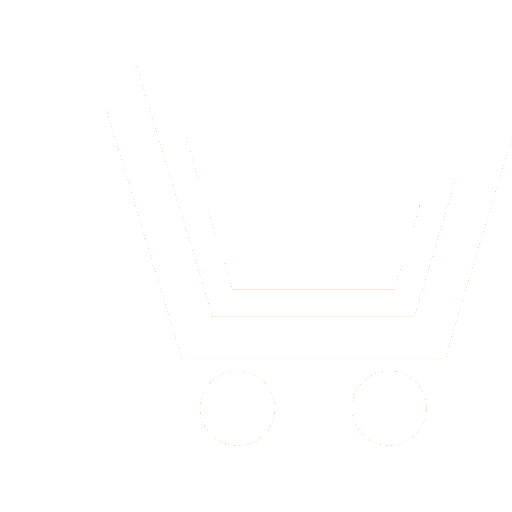
Journal Neurocomputers №2 for 2010 г.
Article in number:
Neural networks with non-uniqueness of values of current stats of elements
Authors:
А.С. Makarenko, V.M. Bilyuga
Abstract:
In the given work the model of neural network which has the multiple-valued solutions is explored. The basic feature is that in the activation function of neuron anticipation is entered. Thus, if on the entrance of neural network to give some entrance vector, on an output not single value can be received, as in the case of the already explored models. That is the feature of model that a multivaluedness appears in the case of sigmoid - like activation function, which is intrinsic to usual artificial neural models. For simplification of program realization as the basic case the neural Hopfield network with the piece-linear activation function had been chosen, that also the conduct of model enables analytically to explore. The possible consequences of the received solutions for the principles of operation of the biological systems (in particular functioning of consciousness) are considered, and also consequences for establishing of work principles of new types of the computer systems which would have the inherit principles of work common to the living systems are discussed.
Pages: 35-39
References
- Саймон ХайкинНейронные сети. Полный курс. М. Изд-во Вильямс. 2006. 1104 с.
- Галушкин А.И.Теория нейронных сетей. Т.1. Нейрокомпютеры и их применение. М.: Изд-во ИПРЖР. 2000. 416 с.
- Оссовский С.Нейронные сети для обработки информации. М.: Финансы и статистика. 2002. 344 с.
- Каллан Р. Основные концепции нейронных сетей. М.: Изд-во Вильямс. 2002. 287 с.
- Лоскутов А.С. , Михайлов А.С. Введение в синергетику. М.: Наука. 1990.
- Chua L.O., Yang L. Cellular neural networks: Theory // IEEE Trans. Circuits Systems I. 1988. V. 35. P. 1257 - 1272.
- Hopfield J.J. Neural Networks and Physical Systems with Emergent Collective Computational Abilities // Proceed. Of Nat. Acad. Sci. USA. 1982. V. 79. N. 8. P. 2554 - 2558.
- Tsang E.C.C., Qiu S.S., Yeung D.S. Stability Analysis of a discrete Hopfield Neural Network with Delay // Neurocomputing. 2007. V. 70. P. 2598 - 2602.
- Sompolinsky H., Kanter L. Temporal Association in Assymmetric Neural Networks // Phys. Rev. Lett. 1986. V. 57. P. 2961 - 2864.
- Forti M., Grazzini M., Nistri P., Pancioni L. Generalized Lyapunov Approach for Convergence of Neural Networks with Discontinuous or non - Lipshitz Activations // Physica D. 2006. V. 214. P. 88 - 99.
- Николс Джон, Мартин Роберт, Валлас Брюс, Фук Пол. Отнейронакмозгу. М.: ЕдиториалУРСС. 2003. 672 с.
- Труды международной конференции ICNC 09, Сентябрь 2009, Ростов - на - Дону, Т. 1-3. Ростов - на - Дону: Изд-во Южного Федерального университета. 2009.
- Макаренко А.С.Модели общественных явлений и сценарные подходы в принятиирешений //Системные исследования и информационные технологии. 2003. № 3. С.127 - 142.
- Макаренко А.С. Системный анализ и моделирование социальных систем: новые возможности // Актуальные проблемы экономики. 2004. № 9(39). С. 79 - 83.
- Makarenko A. Cellular Automata with Anticipation: Some new Research Problems // Int. Journal of Computing Anticipatory Systems (Belgium). 2008. V. 20. P. 230 - 242.
- Dubois D. Incursive and hyperincursive systems, fractal machine and anticipatory logic. Computing Anticipatory Systems: CASYS 2000 - Fourth International Conference // Published by the American Institute of Physics, AIP Conference Proceedings 573. P. 437 - 451.