350 rub
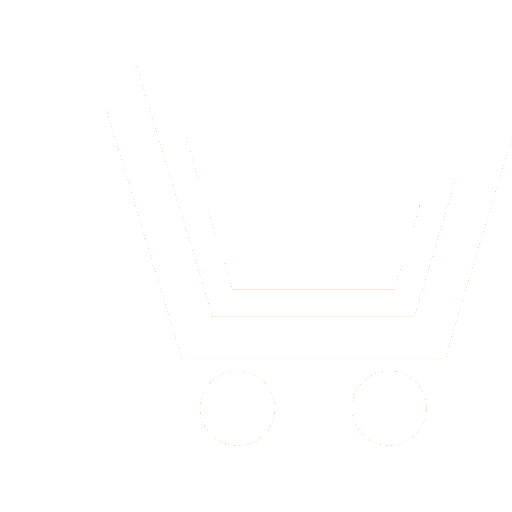
Journal Neurocomputers №5 for 2009 г.
Article in number:
Neural Net-s Usage for Stationary Field-s Modelling
Authors:
N.I. Korsunov, K.E. Burnaev, A.A. Yudin
Abstract:
The approach of modelling stationary physical fields by using cellular neural networks is considered. The basis of the field-s mathematical model is elliptic partial differential equation with nonlinear boundary conditions. Examples of the problem-s solving by the neuroemulator are given.
Cellular neural networks allow normalizing the stationary physical fields described by the partial differential equations, receiving results, comparable to the solutions base on widely used numerical methods with a small saving of time. Its application facilitates paralleling process of calculations at realization of algorithms on usual computer techniques and ideally approaches for realization of algorithms on neurocomputers
Pages: 44-56
References
- Тихонов А.Н., Самарский А.А. Уравнения математической физики. М: Издат. МГУ. 1977.
- Халоу Ф.Н. Вычислительные методы в гидродинамике. / Под ред. Б.Олдера и др. М: Мир. 1967.
- Ильин В.П. Линейная алгебра: от Гаусса до суперкомпьютеров будущего. Природа. 1999. №6.
- Apel T., Mille F. Realization and comparison of various mesh refinement strategies near edges.
- David L Marcum, Kelly P Gaither. Solution adaptive Unstructured grid Generation Using Pseudo-Pattern Recognition Techniques.
- Gupta A., Karyptis G., Kumar V. Highly Scalable Parallel Algorithms for Sparse Matrix Factorizations // IEEE Transactions on Parallel and Distributed Systems. 1995. V.8, № 5. P.502-520.
- Lagaris I.E., Likas A., Fotiadis D.I. Artificial Neural Networks for Solving Ordinary and Partial Differential Equations // IEEE Transactions on Neural Networks. 1998. V.9. № 5. P.422-448.
- Meade A.J., Jr and Fernandez A.A. The numerical solution of linear ordinary differential equations by feedforward neural networks // Mathematical Computer Modeling. 1994. V.19. № 12. P. 1-25.
- Wang L.and Mendel J.M. Structured trainable networks for matrix algebra // IEEE Int. Joint Conference on Neural Networks. 1990.V. 2. P. 125-128.
- Lee H.and Kang I. Neural algorithms for solving differential equations // Journal of Computational Physics. 1990. V.91. P.110-117.
- Lagaris I.E., Likas A., Fotiadis D.I. Artificial Neural Networks for Solving Ordinary and Partial Differential Equations // IEEE Transactions on Neural Networks. 1998. V.4. P. 987-1000.
- Fortuna L., Arena P., Balya D., Zarandy A. Cellular Neural Networks: A Paradigm for Nonlinear Spatio-Temporal Processing // Circuits and Systems Magazine. IEEE. 2001. V. 1. № 4. P. 6-21
- Csaba Rekeczky, Istvan Szatmari, Peter Foldesy and Tamas Roska. Analogic Cellular PDE Machines. Analogical and Neural Computing Systems Laboratory. Computer and Automation Research Institute. Hungarian Academy of Sciences. Budapest. Hungary. Tech. Report. 2004.