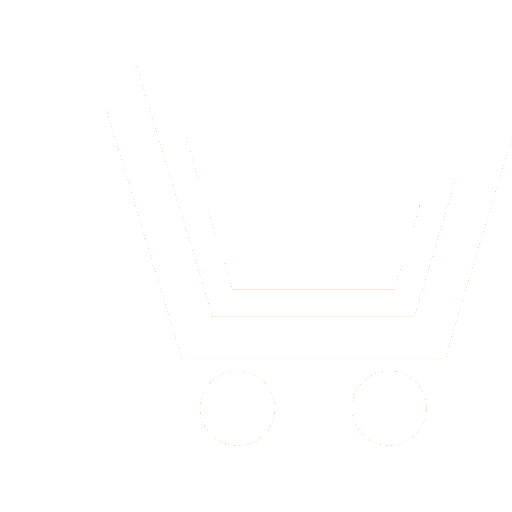
A.G. Gudkov1, N.A. Vetrova2, S.V. Chizhikov3
1,2 Bauman Moscow State Technical University (National Research University) (Moscow, Russia)
2 RUDN University (Moscow, Russia)
1,3 Ltd. Hyperion (Moscow, Russia)
The article discusses and substantiates the need and prospects for developing a methodology for complex technological optimization of the parameters of heterostructure microwave devices. For currently widely used microwave MMICs based on nanosized multilayer semiconductor heterostructures in a variety of fields, the problem of quality assurance is relevant due to the sensitivity of the heterostructure parameters to degradation processes at small layer thicknesses. Degradation of a heterostructure can be caused by both technological factors and operational factors that cause accelerated diffusion of the elements that make up the heterostructure. To ensure the required level of parameters for the purpose of such devices, it is necessary to develop practice-oriented methods (development of a model based on artificial intelligence) for complex technological optimization of the parameters of microwave MIS. Carrying out complex technological optimization based on an intelligent approach with modern advances in machine learning will make it possible to ensure high requirements for product parameters at a minimum cost.
The purpose of the work is to develop a methodological concept for complex technological optimization of the parameters of heterostructural microwave devices, taking into account the specifics of the monolithic integrated design of the circuits of such devices and the design and technological difficulties in ensuring the required purpose parameters both during manufacture and during their operation during the designated service life.
As a result, an intelligent CTO model of heterostructural microwave devices in a monolithic integrated design was developed based on four basic CTO principles, including methods of bagging, boosting, concepts of Bayesian inversion networks, neural network approaches with training using supervised, unsupervised, and deep learning methods. An algorithm has been developed for statistical control of the technological process of manufacturing microwave devices based on heterostructures.
The results of the study can be used in the development, production and operational support by the manufacturer of heterostructure microwave devices for a wide range of purposes, which will increase production efficiency, reduce costs and improve product quality.
The research was carried out with the financial support of the Russian science Foundation in the framework of agreement No. 19-19-00349-П in the theme: “A method and a multichannel multifrequency microwave radiothermography on the basis of monolithic integrated circuits for finding the 3D distribution and dynamics of brightness temperature in the depths of the human body”.
Gudkov A.G., Vetrova N.A., Chizhikov S.V. Methodological aspects of instrumentation technology: machine learning methods and artificial intelligence for the development and production of heterostructure microwave devices. Nanotechnology: development and applications – XXI century. 2023. V. 15. № 3. P. 47–56. DOI: https://doi.org/10.18127/j22250980-202303-05 (in Russian)
- Gudkov A.G. Radioapparatura v usloviyah rynka. Kompleksnaya tekhnologicheskaya optimizaciya. M.: «SAJNS-PRESS». 2008. 336 s. (in Russian).
- Gudkov A.G. et al. The sensitivity research of multiparameter biosensors based on HEMT by the mathematic modeling method. Journal of Physics: Conference Series. 2017. V. 917, Art. № 042016 (Saint Petersburg OPEN 2017) DOI:10.1088/1742-6596/917/4/042016.2.
- Tikhomirov V.G. et al. Optimization of the parameters of HEMT GaN/AlN/AlGaN heterostructures for microwave transistors using numerical simulation//Semiconductors. 2016. V. 50. № 2. R. 244–248.
- Sal'nikov A.S. i dr. Eksperimental'noe issledovanie i postroenie modelej passivnyh komponentov SVCh monolitnyh integral'nyh skhem s uchetom tekhnologicheskogo razbrosa parametrov. Doklady TUSURa. Chast' 2 «Elektronika izmeritel'naya tekhnika, radiotekhnika i svyaz'». 2012. №2 (26). S. 113–118 (in Russian).
- Sal'nikov A.S. i dr. Programmnoe obespechenie dlya hraneniya rezul'tatov izmerenij SVCh MIS i statisticheskogo analiza v sostave sistemy INDESYS-MS. Doklady TUSURa. Chast' 2 «Informacionnye tekhnologii». 2012. №2 (26). S. 218–223 (in Russian).
- Gudkov A.G. Neopredelennosti tekhnologicheskoj optimizacii. Naukoemkie tekhnologii. 2003. T. 4. № 6. S. 65–68 (in Russian).
- Gudkov A.G. Process razrabotki novogo vysokotekhnologichnogo naukoemkogo tovara. Naukoemkie tekhnologii. 2003. T. 4. № 6. S. 69–83 (in Russian).
- Gudkov A.G. et al. A Termostating Device for Storage of Thrombocyte-Containing Media. Biomedical Engineering. 2012 V. 46 (3). P. 104–105.
- Gudkov A.G. i dr. Termostatiruemoe ustrojstvo dlya hraneniya trombocitsoderzhashchih sred. Medicinskaya tekhnika. 2012. №3(273). S. 54 (in Russian).
- Bushminskij I.P. i dr. Konstruktorsko-tekhnologicheskie osnovy proektirovaniya poloskovyh mikroskhem. Pod. red. I.P. Bushminskogo. M.: Radio i svyaz'. 1987. 272 s. (in Russian).
- Agasieva S.V. i dr. Povyshenie nadezhnosti i kachestva GIS i MIS SVCh. Kniga 1. Pod red. A.G. Gudkova i V.V. Popova. M.: OOO «Avtotest». 2012. 212 s. (in Russian).
- Agasieva S.V. i dr. Povyshenie nadezhnosti i kachestva GIS i MIS SVCh. Kniga 2. Pod red. A.G. Gudkova i V.V. Popova. M. OOO «Avtotest». 2013. 214 s. (in Russian).
- Agasieva S.V. i dr. Povyshenie nadezhnosti i kachestva GIS i MIS SVCh. Kniga 3. Pod red. V.N. V'yuginova, A.G. Gudkova i V.V. Popova. M.: OOO NTP «Virazh-Centr». 2016. 252 s. (in Russian).
- Vetrova N.A., Filyaev A.A. Kriterij ocenki nejrosetevoj modeli geterostrukturnyh nanoelektronnyh ustrojstv dlya prognozirovaniya ih elektricheskih parametrov. Vestnik Rossijskogo universiteta druzhby narodov. Ser.: Inzhenernye issledovaniya. 2022. T. 23. № 1. S. 7–14 (in Russian).
- Pchelintsev K.P., Vetrova N.A., Shashurin V.D. Modeling of the degradation of resonsnt-tunneling diodes using artificial neural networks. Journal of Surface Investigation: X-Ray, Synchrotron and Neutron Techniques. 2022. T. 16. № 1. S. 82–85.
- Vetrova N.A., Pchelincev K.P., Shashurin V.D., Gudkov A.G., Solov'ev Yu.V. Nejrosetevye metody dlya TCAD-sred modelirovaniya geterostrukturnyh nanoelektronnyh priborov s poperechnym tokoperenosom. Nanotekhnologii: razrabotka, primenenie – XXI vek. 2021. T. 13. № 1. S. 5–11. DOI: https://doi.org/10.18127/j22250980-202101-01 (in Russian).
- Vetrova N.A., Pchelincev K.P., Shashurin V.D. Perseptronnaya set' prognozirovaniya elektricheskih harakteristik rezonansno-tunnel'nogo dioda. Nanotekhnologii: razrabotka, primenenie – XXI vek. 2021. T. 13. № 4. S. 5–9 DOI: https://doi.org/10.18127/j22250980-202104-01 (in Russian).
- Svidetel'stvo o registracii programmy dlya EVM 2021681910. Prediktor pokazatelej bezopasnosti donorskoj krovi i ee komponnentov v usloviyah statisticheski upravlyaemogo tekhnologicheskogo processa. N.A. Vetrova, A.A. Filyaev, V.Yu. Leushin, A.G. Gudkov, V.N. Lemondzhava. 2021 (in Russian).
- Pham H. (ed.). Springer handbook of engineering statistics. Springer Science & Business Media. 2006.
- Allen Dauni. Bajesovskie modeli. Bajesovskaya statistika na yazyke Python. DMK-Press. 2018 (in Russian).
- GOST R ISO 22514-1-2015 Statisticheskie metody. Upravlenie processami. Chast' 1. Obshchie principy. Vved. 2016. 01. 07. M.: Standartinform. 2016. 23 s. (in Russian).
- GOST R ISO 22514-2-2015 Statisticheskie metody. Upravlenie processami. Chast' 2. Ocenka prigodnosti i vosproizvodimosti processa na osnove modeli ego izmeneniya vo vremeni. Vved. 2015. 20. 11. M.: Standartinform. 2016. 24 s. (in Russian).
- GOST R ISO 22514-3-2015 Statisticheskie metody. Upravlenie processami. Chast' 3. Analiz prigodnosti mashin na osnove dannyh izmerenij edinic produkcii. Vved. 2015. 18. 11. M.: Standartinform. 2016. 27 s. (in Russian).
- GOST R ISO 7870-1-2011 Statisticheskie metody. Kontrol'nye karty. Chast' 1. Obshchie principy. Vved. 2011. 09. 11. M.: Standartinform. 2012. 16 s. (in Russian).
- GOST R ISO 7870-2-2015 Statisticheskie metody. Kontrol'nye karty. Chast' 2. Kontrol'nye karty Shuharta. Vved. 2015. 06. 10. M.: Standartinform. 2019. 42 s. (in Russian).
- GOST R ISO 7870-4-2013 Statisticheskie metody. Kontrol'nye karty. Chast' 4. Karty kumulyativnyh summ. Vved. 2013. 17. 12. M.: Standartinform. 2014. 52 s. (in Russian).