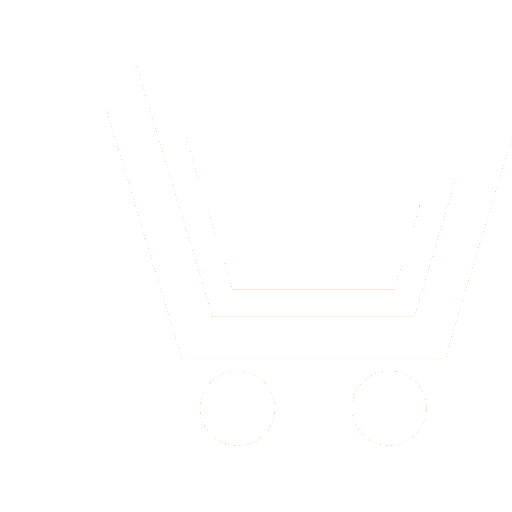
E.N. Gorlacheva¹, N.P. Goncharova²
1,2 Bauman Moscow State Technical University (Moscow, Russia),
1 gorlacheva@yandex.ru, 2 nataly.gonn79@gmail.com
Management decision making is associated with a high level of uncertainty. Uncertainty is associated with the presence of a large amount of low-quality information. It is possible to simplify making management decisions based on such information using machine learning methods that allow you to quickly and cost-effectively process information by presenting it to a decision-maker in a convenient form.
The article analyzes the classes of machine learning problems, such as: supervised learning, unsupervised learning, and reinforcement learning. In each class, those tasks are identified that solve tasks within the framework of various project management processes. For each task of project management, an algorithm is selected, initial data and results are indicated. The purpose of the article is to adapt machine learning tasks to solve management problems within the framework of project management processes. Based on the results of the study, the task of identifying anomalies is solved within the framework of the process of managing changes in the scientific and technical project.
- Belikova K. Obuchenie nejroseti s uchitelem, bez uchitelya, s podkrepleniem — v chem otlichie - Kakoj algoritm luchshe - [Elektronnyj resurs] Rezhim dostupa: https://neurohive.io/ru/osnovy-data-science/obuchenie-s-uchitelem-bez-uchitelja-s-podkrepleniem/ (data obrashcheniya 11.09.2020) (In Russian).
- Vetrov D.P., Kropotov D.A. Lekciya 1. Razlichnye zadachi mashinnogo obucheniya. MGU, VMiK, kaf. MMP Speckurs «Bajesovskie metody mashinnogo obucheniya» (In Russian).
- Galimov R.G. Osnovy algoritmov mashinnogo obucheniya – obuchenie s uchitelem. Alleya Nauki. 2017. № 14. S. 810–817 (In Russian).
- Gudfellou I., Bengio J., Kurvill A. Deep Learning an MIT press book. [Elektronnyj resurs]. Rezhim dostupa: https:// deeplearningbook.org (data obrashcheniya 23.09.2020).
- Gorlacheva E.N., Ochichenko N.P. Analiz sovremennyh metodov prognozirovaniya na primere indeksa promyshlennogo proizvodstva. Nelinejnyj mir. 2020. T. 18. № 2. S. 42–52 (In Russian).
- Donskoj V.I. Algoritmicheskie modeli obucheniya klassifikacii: obosnovanie, sravnenie, vybor. Simferopol': DIAJPI. 2014 (In Russian).
- D'yakonov A. Poisk anomalij [Elektronnyj resurs] Rezhim dostupa: https://dyakonov.org/2017/04/19/%D0%BF%D0%BE%D0% B8%D1%81%D0%BA-%D0%B0%D0%BD%D0%BE%D0%BC%D0%B0%D0%BB%D0%B8%D0%B9-anomaly-detection/ (data obrashcheniya 10.10.2020) (In Russian).
- Kilin G.A., Zhdanovskij E.O. Preimushchestva ispol'zovaniya obucheniya s podkrepleniem dlya obucheniya nejronnyh setej. Avtomatizirovannye sistemy upravleniya i informacionnye tekhnologii. 2018. T. 1. S. 152–158 (In Russian).
- L'yu T. Learning to Rank for Information Retrieval. Foundations and Trends in Information Retrieval. 2009.V. 3. № 3. P. 225–331.
- Malahova O.V. Sushchnost' informacionnogo obespecheniya i ego rol' v innovacionnoj deyatel'nosti. Transportnoe delo Rossii. 2013. № 5(108). S 24–29 (In Russian).
- Romanyuk A.V. Neopredelennost' v predprinimatel'skoj deyatel'nosti. Gornyj informacionno-analiticheskij byulleten' (nauchnotekhnicheskij zhurnal) 2007. № 9. S 80–86 (In Russian).
- Sadovnikov P. Interesnye algoritmy klasterizacii, chast' pervaya: Affinity propagation [Elektronnyj resurs] Rezhim dostupa: https://habr.com/ru/post/321216/ (data obrashcheniya 10.10.2020) (In Russian).
- Bannat A. Artificial Cognition in Production Systems. IEEE Transactions on automation science and engineering. 2011. V. 8. № 1, P. 148–174.
- Hobbs L., Hilson S., Louend Sh. Oracle9iR2. Razrabotka i ekspluataciya hranilishch dannyh. M.: Kudic-obraz., 2004. , Portal znanij [Elektronnyj resurs] Rezhim dostupa: http://statistica.ru/ (data obrashcheniya: 3.10.2020) (In Russian).
- Chatkin V.V. Razlichiya mezhdu iskusstvennym intellektom, mashinnym obucheniem i glubokim obucheniem. Konkurentosposobnost' territorij. 2019. № 3. S. 185–186 (In Russian).
- Erikson G., Frenks L., Rorer B. Kak vybirat' algoritmy dlya mashinnogo obucheniya Microsoft Azure [Elektronnyj resurs] Rezhim dostupa: https://habr.com/ru/company/microsoft/blog/317512/ (data obrashcheniya 5.10.2020) (In Russian).
- Gorlacheva E.N. Upravlenie znaniyami. Povyshenie nadezhnosti GIS i MIS SVCh: Monografiya / Pod red. V.N. V'yuginova, A.G. Gudkova, V.V. Popova. Kn. 3. M.: Avantest. 2016. S. 230–248 (In Russian).
- Obuchenie s podkrepleniem v mashinnom obuchenii [Elektronnyj resurs] Rezhim dostupa: https://evergreens.com.ua/ru/ articles/reinforcement-learning.html (data obrashcheniya 10.10.2020) (In Russian).
- Prikaz Goskomvuza RF ot 15.09.1994 N 929 «Ob utverzhdenii poryadka formirovaniya, finansirovaniya i vypolneniya innovacionnyh nauchno-tekhnicheskih programm i proektov» [Elektronnyj resurs] Rezhim dostupa: http://www.consultant.ru/ cons/cgi/online.cgi-req=doc&base=EXP&n=507996#031932508714757657 (data obrashcheniya 10.10.2020) (In Russian).
- Frenks L. Popular Machine Learning Data [Elektronnyj resurs] Rezhim dostupa: https://github.com/FranksMachine/PopularMLData (data obrashcheniya 04.10.2020) (In Russian).