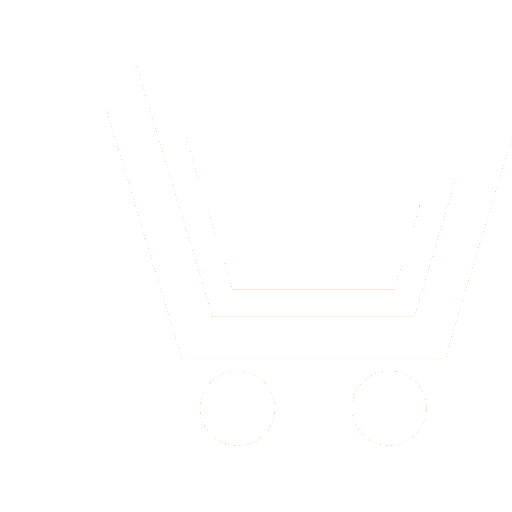
E.N. Gorlacheva – Ph.D. (Econ.), Associate Professor, Bauman Moscow State Technical University
E-mail: gorlacheva@yandex.ru
Statement of the problem. Modern high-technology enterprises of radio complex work in complex conditions that are characterized by the market glut, drastically changes in market demand dynamics, increasing production variety and decreasing volumes of output. From the one hand such requirements could be fulfilled in terms of high stability and well-tuned production processes. From the other, these requirements could be fulfilled by in-time reaction on production systems or production processes variances. However the efficiency of such adaptive and reactive steps depends on synchronized with the production processes information that is available online.
Aim of the work – the purpose of the paper is to show how modern information systems change the high- technology enterprises in many aspects starting from the automation of several processes and reshaping the whole organization structure in total. Using the new approach based on the neuro networks the author describes the appearance of the new type of manufacturing production system the kern of which is the cyber twin of the enterprise.
Results. The usage of modern information and communication technologies will allow collecting operative information from the information modules of the production and commercial systems. This process could be considered as the quantitative shift from management systems that work offline to management systems that work online. Traditional automation is not capable to cope with the increasing complexity of the modern production and commercial systems. The automated intellectual systems are necessary that allow reacting on the unpredictable situation, planning further actions capable to learning (machine learning). As trends one could consider principles of machine learning where in the base of each high technology enterprise the development goes on despite of the industry. The other aspect of this problem is the velocity of the market entrance and the picking up growth of the enterprises where new technologies are implemented in the business processes. The problems appear in scaling the enterprises and the increasing of any volume: traffic, output, etc. It is important that such enterprises increase competences inside the enterprises and use open source decisions.
Practical significance. Thus, it is not enough for the elaboration of a new product the only concept and program code, the elaborators should think over about promotion of the product and today it will realize by the marketing on data management. The realization of this approach will definitely increase the efficiency of the manufacturing and distributive systems in radioelectronic complex.
- Pavellek G. Kompleksnoe planirovanie promyshlennyh predpriyatij: bazovye principy, metodika, IT-obespechenie: Per. s nem. M. Al'pina Pablisher. 2015. 366 s. (In Russian).
- Gudkov A.G. i dr. Povyshenie nadezhnosti GIS i MIS SVCH. Pod red. A.G. Gudkova, V.V. Popova. Kn. 2. M.: OOO «Avantest». 2013. 214 s. (In Russian).
- Gudkov A.G. i dr. Povyshenie nadezhnosti GIS i MIS SVCH. Pod red. A.G. Gudkova, V.V. Popova. Kn. 1. M.: OOO «Avantest. 2012. 212 s. (In Russian).
- Gudkov A.G. i dr. Povyshenie nadezhnosti GIS i MIS SVCH. Pod red. V.N. V'yuginova, A.G. Gudkova, V.V. Popova. Kn. 3. M.: OOO «Avantest». 2016. 252 s. (In Russian).
- Gudkov A.G. Radioapparatura v usloviyah rynka. Kompleksnaya tekhnologicheskaya optimizaciya. M.: Sajns-Press. 2008. 336 s. (In Russian).
- Bannat A. et al. Artificial Cognition in Production Systems. IEEE Transactions on automation science and engineering. 2011. V. 8. № 1. P. 148–174.
- Turovec O.G., Rodionova V.N. Sovremennye problemy organizacii mashinostroitel'nogo proizvodstva. Voronezh: FGBOU VO «Voronezhskij gosudarstvennyj tekhnicheskij universitet». 2017. 161 s. (In Russian).
- Stankevich L.A. Kognitivnye sistemy i roboty. SPb.: Politekh-press. 2019. 631 s. (In Russian).
- Stankevich L.A. Kognitivnye povedencheskie sistemy. HIH Mezhdunar. nauch.-tekhn. konfer. «Nejroinformatika – 2017»: Lekcii po nejroinformatike. M.: Izd-vo NIYAU MIFI. 2017. S. 31–97 (In Russian).
- Tanriverdi H. Information Technology Relatedness, Knowledge Management Capability and Performance of Multibusiness Firms. MIS Quarterly. 2005. V. 29. P. 311–334.
- Kulikovski R. Optimal'nye i adaptivnye processy v sisteme avtomaticheskogo regulirovaniya. M.: Nauka. 1967 (In Russian).
- Volkova V.N., Voronkov V.A., Denisov A.A. i dr. Teoriya sistem i metody sistemnogo analiza v upravlenii i svyazi. M.: Radio i svyaz' (In Russian).
- LeCun, Yann et al. Gradient-based learning applied to document recognition. Proceedings of the IEEE. 1998. V. 86.11. P. 2278–2324. Rezhim dostupa: http://yann.lecun.com/exdb/publis/pdf/lecun-98.pdf (data obrashcheniya: 25.05.2018)
- Goodfellow, Ian et al. Generative adversarial nets. Advances in Neural Information Processing Systems. 2014. Rezhim dostupa: https://arxiv.org/pdf/1406.2661v1.pdf (data obrashcheniya: 29.05.2018).
- Elman J.L. Finding structure in time. Cognitive science 1990. V. 14.2. P. 179–211. Rezhim dostupa: https://crl.ucsd.edu/~elman/Papers/fsit.pdf (data obrashcheniya: 29.05.2018).
- Nikolenko S., Kadurin A., Arhangel'skaya E. Glubokoe obuchenie. SPb.: Piter. 2020.480 s. (In Russian).