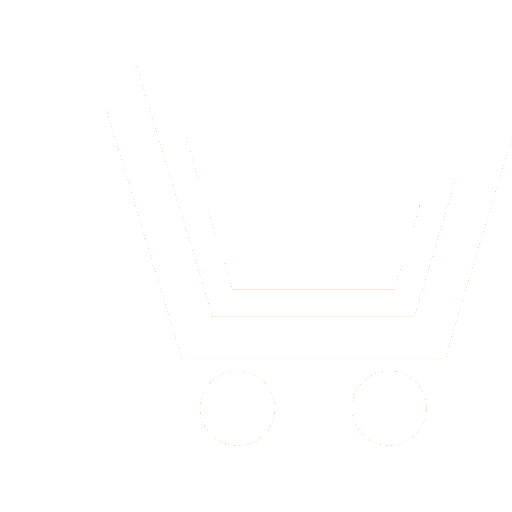
E.A. Nekrasov1, A.V. Degtyar2, T.A. Markina3, I.B. Gosudarev4
1–4 ITMO (Saint Petersburg, Russia)
1 evgeniy@nekrasov.pw, 2 degtyar.avlad@gmail.com, 3 markina_t@itmo.ru, 4 goss@itmo.ru
A significant number of distributed, complex, high-dimensional processes or control objects today are observed not by one sensor, but by a set of measuring instruments that make it possible to measure the same quantities repeatedly, providing redundancy of measurements, and therefore the basis for increasing the reliability of control using various pattern recognition methods. The maximum amount of information about such objects or processes can be obtained by extracting data not only on the absolute values of the controlled characteristics, but also on their mutual relationship, which makes it possible to make a group point representation. However, the processing of such representations has its own characteristics, requiring the use of metric methods that are resistant to noise and image distortion.
The work examines group point representations of objects or processes that have a complementary structure, distributed in time or space, and allowing one to interpret the physical and geometric meaning of each mark in the image.
Information that makes it possible to reliably compare the input sample (process) with one of the representatives of the classes (templates) is contained in the unique relative position of k points (graph vertices) and the characteristics of the points of the multipoint representation themselves. In practical applications, mathematical descriptions of group point representations are typically represented in matrix form, contour models, or associated solid images. And all mathematical models of GTO, according to the methods of synthesizing selective traits, can be reduced to three types: Cartesian, polar and chain. An analysis of known methods for recognizing images of a group point representation shows that at present there is no universal method that has such qualities as acceptable labor intensity, high noise immunity, performance in the presence of omissions of signal marks, the appearance of false marks and, most importantly, disorder of the points of the recognized image. The approach presented in the work makes it possible to implement a methodology for representing and subsequent recognition of controlled objects and processes with a sufficient degree of reliability and accuracy.
Nekrasov E.A., Degtyar A.V., Markina T.A., Gosudarev I.B. Methods of representation and processing of information about set of multidimensional processes and objects in the form of group point representations. Information-measuring and Control Systems. 2025. V. 23. № 1. P. 19−32. DOI: https://doi.org/10.18127/j20700814-202501-03 (in Russian)
- Evin I.A. Vvedenie v teoriyu slozhny`x setej // Komp`yuterny`e issledovaniya i modelirovanie. 2014. T. 2. № 2. C. 121–141.
- Cun-Lai Pu, Wen, Jiang Pei, Andrew Michaelson. Robustness analysis of network controllability. Physica A. 2022. T. 391. V. 18.
P. 4420–4425. - Albert-laszlo Barabasi, Frangos J. Linked: The New Science of Networks. Perseus Publishing. Cambridge. 2022. 324 p.
- Kitsak M., Gallos L.K., Havlin S., Liljeros F., Muchnik L., Stanley H.E., Makse H.A. Influential Spreaders in Networks. Nature Physics. 2019. T. 6. V. 11. P. 888–893.
- Mile Sikic, Alen Lancic, Nino Antulov-Fantulin, Hrvoje Stefancic. Epidemic centrality -- is there an underestimated epidemic impact of network peripheral nodes? European Physical Journal B. 2023. T. 86. № 10. P. 440–457.
- Timóteo S., Correia M., Rodríguez-Echeverría S., Freitas H., Heleno R. Multilayer networks reveal the spatial structure of seed-dispersal interactions across the Great Rift landscapes. Nature Communications. 2018. T. 9. V. 1. P. 745–755.
- Battiston F., Nicosia V., Latora V. Structural measures for multiplex networks. Physical Review E. 2021. T. 89. V. 3. P. 328–349.
- vDe Domenico M., Sole-Ribalta A., Omodei E., Gomez S., Arenas A. Ranking in interconnected multilayer networks reveals versatile nodes. Nature Communications. 2019. T. 6. P. 68–79.
- Doerry A.W., Dubbert D.F., Thompson M.E., Gutierrez V.D. A portfolio of fine resolution Ka-band SAR images: Part I. Sandia National Laboratories / SPIE Defense and Security Symposium. 2005. – March 28–April 1.
- Galereya RSA izobrazhenij Sandia National Laboratories. URL: http://www.sandia.gov/RADAR/imagery.html (data obrashheniya: 25.06.2020).
- A Sourcebook for the Use of the FGAN Tracking and Imaging Radar for Satellite Imaging. URL: https://fas.org/spp/military /program/track/fgan.pdf (data obrashheniya: 07.04.2019).
- Kaplin A.Yu., Korotin A.A., Nazarov A.V., Yakimov V.L. Algoritm klassifikacii i vosstanovleniya iskazhenny`x n-merny`x gruppovy`x tochechny`x ob``ektov na osnove kombinatornogo poiska fragmentov. Trudy` SPIIRAN. 2016. Vy`p. 6(49). C. 167–189.
- Furman Ya.A., Rozhenczov A.A., Xafizov R.G. i dr. Tochechny`e polya i gruppovy`e ob``ekty` / pod red. Ya.A. Furmana. M.: Fizmatlit. 2014. 340 s.