350 rub
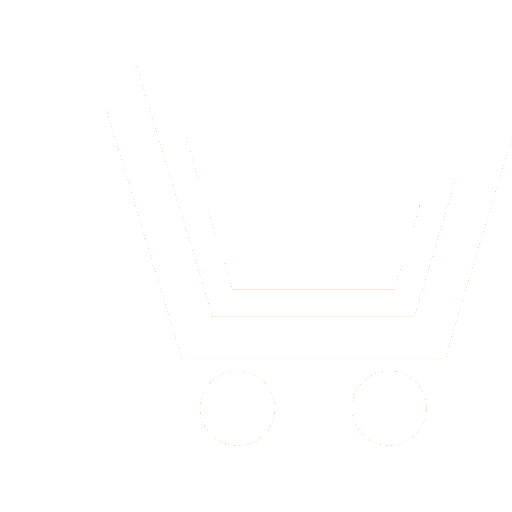
Journal Information-measuring and Control Systems №12 for 2016 г.
Article in number:
Current state and development possibility of impulse neuron network
Keywords:
spiking neural network
LIF-model
Hodgkin-Huxley model
Izhekevich neuron model
synapse plasticity in impulse neural network
Authors:
V.I. Terekhov - Ph.D. (Eng.), Associate Professor, Department of Information Processing and Control Systems, Bauman Moscow State Technical University
E-mail: terekchow@bmstu.ru
R.V. Zhukov - Post-graduate Student, Department of Information Processing and Control Systems, Bauman Moscow State Technical University
E-mail: zhukov@student.bmstu.ru
Abstract:
The article is devoted to the spiking neural networks and his practice usage. In current time classic neural network, based on models and principles witch was described in 1940s by McCulloch, Pitts and Donald Hebb, are widely used.
However, modelling more complex neurons behavior became possible with increasing computational power of modern computer engineering. This fact made it possible creating new models of artificial neuron, named spiking neurons. Three models of spiking neurons are apply in current time: LIF-model, Hodgkin-Huxley model and Izhekevich neuron model. Spiking neural networks (SNN) show results, comparable with classic neural networks.
Different researches show, that SNN can be used in different problems, such as classification problem of video, audio and data from inertial devices. In particular, SNN can be applied in the problem of municipal CCTV systems - optimization.
Building of hybrid intelligent systems with two types of neural networks and used genetic algorithms for optimization spiking neuron-s parameters is perspective direction for further research.
Pages: 143-145
References
- Gerstner W., Kistler W.M. Spiking neuron models: Single neurons, populations, plasticity. Cambridge university press. 2002.
- Burkitt A.N. A review of the integrate-and-fire neuron model: I. Homogeneous synaptic input // Biological cybernetics. 2006. V. 95. № 1. P. 1-19.
- Hodgkin A.L., Huxley A.F. A quantitative description of membrane current and its application to conduction and excitation in nerve // The Journal of physiology. 1952. V. 117. № 4. P. 500-544.
- Izhikevich E.M. et al. Simple model of spiking neurons // IEEE Transactions on neural networks. 2003. V. 14. № 6. P. 1569-1572.
- Diehl P.U., Cook M. Unsupervised learning of digit recognition using spike-timing-dependent plasticity// Front. Comput. Neurosci. 2015.
- Pravitelstvo Rossijjskojj Federacii. Koncepcija postroenija i razvitija apparatno-programmnogo kompleksa <Bezopasnyjj gorod>. 2014. URL: http://www.rg.ru/2014/12/11/gorod-site-dok.html