350 rub
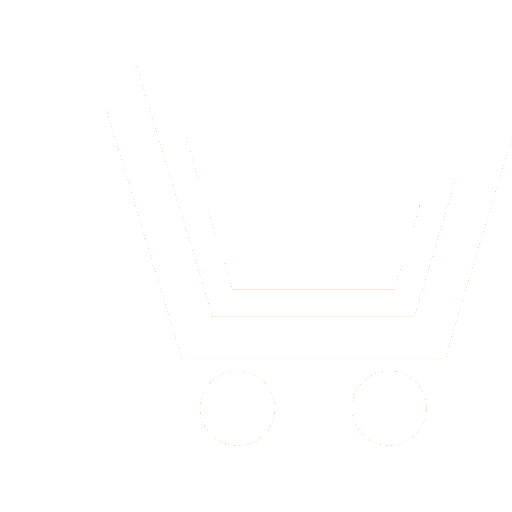
Journal Information-measuring and Control Systems №3 for 2011 г.
Article in number:
Estimation of logical rule quality indices based
on bayesian methodology
Authors:
V. R. Milov, V. G. Baranov, A. Yu. Epshtein, B. A. Suslov
Abstract:
Regularities of a system under study can be specified in a human-understandable way via descriptive models which are frequently represented with the aid of logical rules.
A rule "If R Then C" consists of a precondition R and a consequent C. For some data the statements both in the left and in the right part of the rule can be either true or false.
Application of a rule to a database gives the results which may be represented via a (2×2)-matrix (a contingency table). The main characteristics of a rule: accuracy, completeness, support-are calculated based on this matrix.
Strength of statistical relationship between a precondition and a consequent of the specified rule can be estimated by various means, for example, by association coefficient or contingency coefficient. The main disadvantage of these coefficients is the lack of a simple probabilistic interpretation.
To estimate strength of relationship between a precondition and a consequent within the scope of probability-theoretical approach it is suggested to represent a logical rule with the aid of a Bayesian network with two discrete variables respective to a precondition R and a consequent C.
A posteriori probability of the structure with the link between the variables is supposed to be the measure of statistical relationship between a precondition and a consequent. Thereby, the introduced index represents probability of existence of cause-and-effect relation between a precondition and a consequent of a logical rule depending on available data.
Informativeness is considered to be another index of a logical rule and determined as the average mutual information between a precondition and a consequent.
The indices introduced can be used in knowledge bases generation when building decision support systems.
Pages: 56-61
References
- Барсегян А. А., Куприянов М. С., Степаненко В. В., Холод И. И. Методы и модели анализа данных: OLAP и Data Mining. СПб.: БХВ-Петербург. 2004.
- Баранов В. Г., Милов В. Р., Суслов Б. А. Способы аналитической обработки информации для системы поддержки принятия решений // Информационно-измерительные и управляющие системы. 2010. Т. 8. № 2. С. 12-15.
- Баранов В. Г., Милов В. Р., Суслов Б. А. Интеллектуализация систем диспетчерского управления и сбора данных // Международная научно-техническая конференция «Информационные системы и технологии». НижнийНовгород: НГТУ. 2007.
- An, A., Rule quality measures for rule induction systems: description and evolution / Aijun An, Nick Cercone // Computational Intelligence. 2001. V. 17. № 3. P. 409-424.
- Cooper, G., Herskovits, E., A Bayesian method for the induction of probabilistic networks from data // Machine Learning. 1992. No. 9. P. 309-347.